Image generators can be controlled by more than just text prompts, e.g. with ControlNet. In the past, however, these methods required a great deal of training. FreeControl aims to change that.
Researchers at the University of California at Los Angeles, the University of Wisconsin-Madison, and Innopeak Technology Inc. have developed a new method for controllable text-to-image (T2I) generation, which they call "FreeControl".
FreeControl can be fed with various conditions in addition to the text prompt, such as a sketch, depth map, or point cloud, and can be combined with the Stable Diffusion 1.5, 2.1, and SDXL 1.0 models. The method is similar to the widely used ControlNet, but does not require specially trained models.
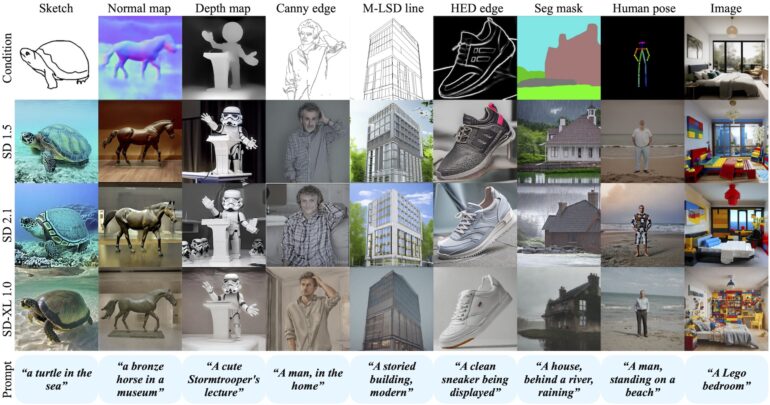
FreeControl requires no training
FreeControl is designed to provide users with fine-grained spatial control over diffusion models. Unlike previous approaches that require additional modules for each type of spatial condition, model architecture, and control point, FreeControl does not require this explicit training.
FreeControl first extracts a set of key features from sample images. These sample images consist of an input image that specifies the image structure, a generated image that is automatically generated from the respective prompt, and a further variant in which the prompt is adapted so that only the essential concept - e.g. a man - and not all details - e.g. a Lego man giving a speech - are included. The extracted features therefore include image composition, content, and style as well as the essential concept. Together they guide the generation of the final image.
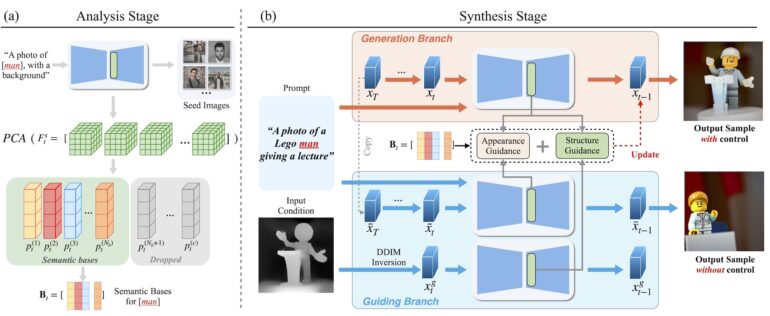
FreeControl supports image-to-image
According to the authors, FreeControl produces high-quality images and is said to achieve better results than other methods that attempt to guide image generation without training. The FreeControl method can also be easily adapted for image-to-image guidance.
ControlNet has provided an initial approach to controlling the output of Stable Diffusion models under conditions other than text-only prompts, and is widely used. GLIGEN, released shortly afterward, went a step further by incorporating position and size information for the required objects in the image.
FreeControl seems to be a logical, even more powerful development of these ideas, on the one hand working independently of resource-intensive training, and on the other hand, accepting a whole range of different input conditions.