An analysis by Epoch AI shows that AI training runs of up to 2e29 FLOP could be technically feasible by the end of the decade. Power supply and chip production are considered the biggest obstacles.
According to a study by research company Epoch AI, AI training runs of up to 2e29 FLOP (floating point operations) could be technically feasible by 2030. This would mean that an AI lab could train a model by the end of the decade that surpasses GPT-4 in computing power to a similar degree as GPT-4 surpassed GPT-2, representing a 10,000-fold increase.
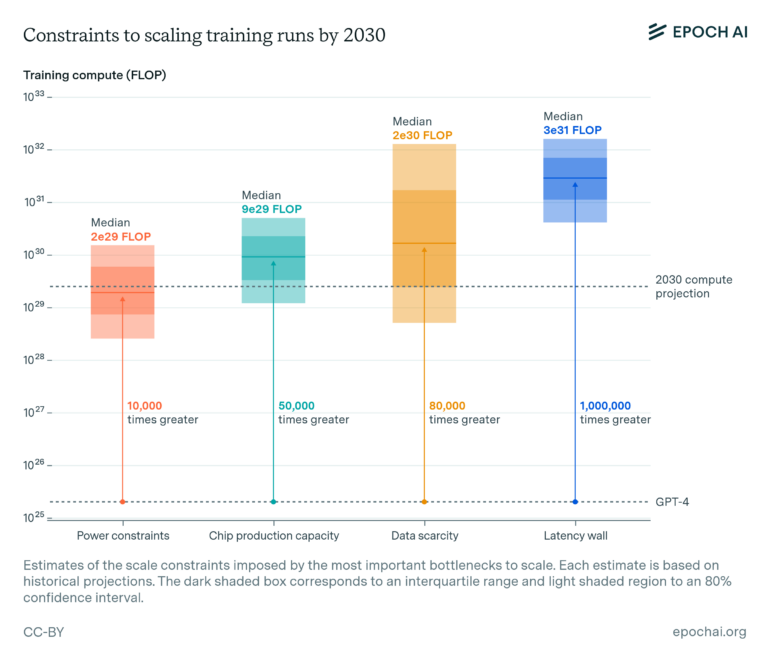
The researchers examined four potential bottlenecks for scaling up AI training runs: power supply, chip production capacity, data scarcity, and the so-called "latency wall," a fundamental speed limit due to unavoidable delays in AI computations.
Power supply and chip production are considered the biggest obstacles. According to the study, training runs supported by a local power supply by 2030 will likely require 1 to 5 GW of power. Geographically distributed training runs, on the other hand, could aggregate a supply of 2 to 45 GW.
Chip production is limited by capacity constraints for advanced packaging and high-bandwidth memory. The researchers estimate that by 2030, there will be enough manufacturing capacity to produce 100 million H100-equivalent GPUs for AI training. This would be sufficient to enable a 9e29 FLOP training run.
Data scarcity
Data scarcity turns out to be the most uncertain constraint: If current trends continue, AI labs would run into a "data wall" for text data in about five years. Multimodal data from images, video, and audio could moderately support scaling and triple the available training data. Synthetic data generation by AI models could further increase this significantly but comes with high computational costs.
The "latency wall" proves to be a distant but noteworthy hurdle. It could be overcome by more complex network topologies, reduced communication latencies, or more aggressive scaling of batch size. Overall, the results suggest that AI labs can scale at a rate of 4x per year by the end of this decade.
However, this presents major challenges that need to be addressed to maintain progress. If AI training runs of this magnitude actually take place, it would be of enormous significance. AI could attract investments of hundreds of billions of dollars and become the largest technological project in human history. If the sheer size translates into more performance and generality, we could experience similarly large advances in AI by the end of the decade as we have since the beginning of the decade.