Anthropic’s new "AI microscope" offers a limited view into the internal representations of its Claude 3.5 Haiku language model, revealing how it processes information and reasons through complex tasks.
One key finding, according to Anthropic, is that Claude appears to use a kind of language-independent internal representation—what the researchers call a "universal language of thought." For example, when asked to generate the opposite of the word "small" in multiple languages, the model first activates a shared concept before outputting the translated answer in the target language.
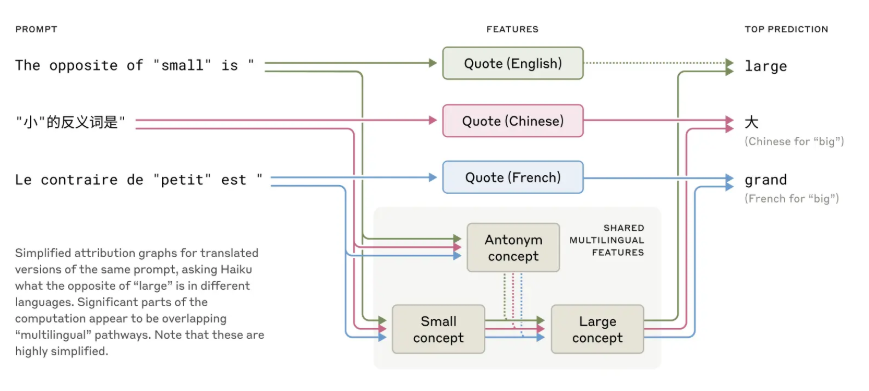
Anthropic reports that larger models like Claude 3.5 exhibit greater conceptual overlap across languages than smaller models. According to the researchers, this abstract representation may support more consistent multilingual reasoning.
The research also examined Claude’s response to questions requiring multiple steps of reasoning, such as: "What is the capital of the state in which Dallas is located?" According to Anthropic, the model activates representations for "Dallas is in Texas" and then links that to "the capital of Texas is Austin." This sequence indicates that Claude is not simply recalling facts but performing multi-step inference.
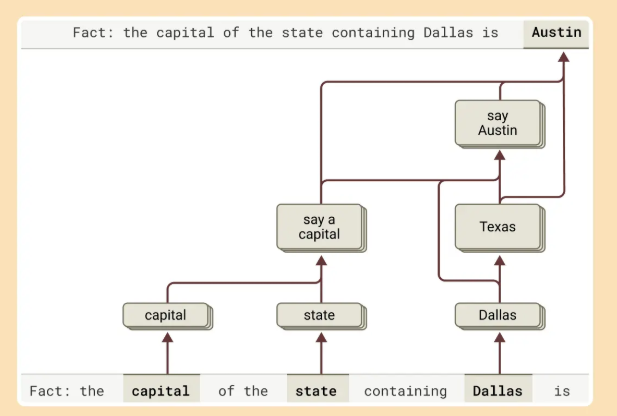
Detecting signs of planning
The researchers also discovered that Claude plans several words in advance when generating poetry. Rather than composing line by line, it begins by selecting appropriate rhyming words, then builds each line to lead toward those targets. If the target words are altered, the model produces an entirely different poem—an indication of deliberate planning rather than simple word-by-word prediction.
For mathematical tasks, Claude employs parallel processing paths - one for approximation and another for precise calculation. Yet when prompted to explain its reasoning, Claude describes a process different from the one it actually used—suggesting that it is mimicking human-like explanations rather than accurately reporting its internal logic. The researchers also note that when given a flawed hint, Claude often generates a coherent explanation that is logically incorrect.
Comparing AI and human language processing
Research from Google offers a parallel line of investigation. A recent study published in Nature Human Behavior analyzed similarities between AI language models and human brain activity during conversation. Google's team found that internal representations from OpenAI's Whisper model aligned closely with neural activity patterns recorded from human subjects. In both cases, the systems appeared to predict upcoming words before they were spoken.
Video: Google
Despite these similarities, the researchers emphasize fundamental differences between the two systems. Unlike Transformer models, which can process hundreds or thousands of tokens simultaneously, the human brain processes language sequentially—word by word, over time, and with repeated loops. Google writes, "While the human brain and Transformer-based LLMs share basic computational principles in natural language processing, their underlying neural circuit architectures differ significantly."