Despite access to research tools and high processing costs, leading language models fell short on complex financial tasks.
A new benchmark from Vals.ai suggests that even the most advanced autonomous AI agents remain unreliable for financial analysis. The best-performing model, OpenAI's o3, managed just 48.3% accuracy—at an average cost of $3.69 per query.
The benchmark was developed in collaboration with a Stanford lab and a global systemically important bank. It consists of 537 tasks modeled on real-world responsibilities of financial analysts, including SEC document review, market research, and forecasting. In total, 22 leading foundation models were evaluated.
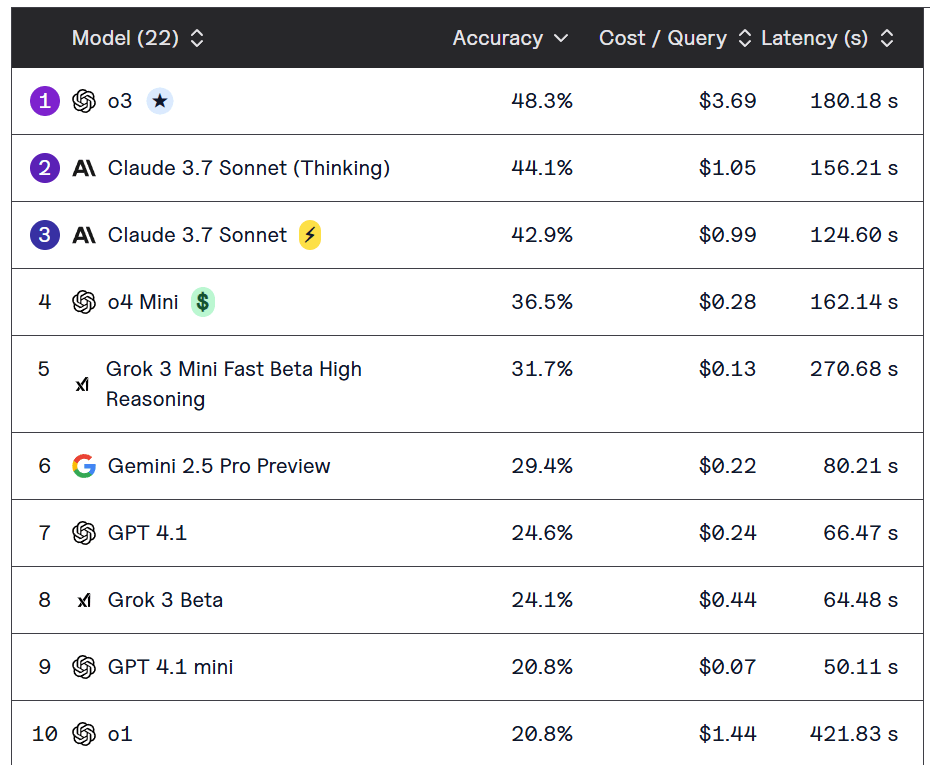
Basic tasks show promise, but financial reasoning remains unreachable
The models demonstrated limited success with basic assignments like extracting numerical data or summarizing text, where average accuracy ranged from 30% to 38%. However, they largely failed on more complex tasks. In the "Trends" category, ten models scored 0%, with the best result—28.6%—coming from Claude 3.7 Sonnet.
To complete these tasks, the benchmark environment gave agents access to tools such as EDGAR search, Google, and an HTML parser. Models like OpenAI's o3 and Claude 3.7 Sonnet (Thinking), which made more frequent use of these tools, generally performed better. In contrast, models like Llama 4 Maverick often skipped tool usage entirely, producing outputs without conducting any research—and showed correspondingly weak results.
But heavy tool use wasn't always a sign of better performance. GPT-4o Mini, which made the most tool calls, still delivered low accuracy due to consistent errors in formatting and task sequencing. Llama 4 Maverick, by contrast, regularly gave responses without conducting searches at all.
In some cases, processing a single query cost over $5. OpenAI's o1 model stood out as particularly inefficient: it had low accuracy and high costs. In practical applications, these expenses would need to be weighed against the cost of human labor.
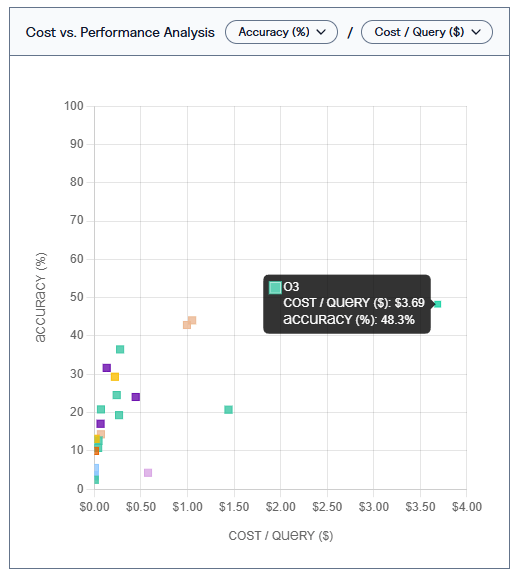
Model performance varied widely. In one task focused on Netflix’s Q4 2024 share buybacks, Claude 3.7 Sonnet (Thinking) and Gemini 2.5 Pro returned accurate, source-backed answers. GPT-4o and Llama 3.3, on the other hand, either missed the relevant information or gave incorrect responses. These inconsistencies highlight the ongoing need for human oversight in areas like prompt engineering, system setup, and internal benchmarking.
A striking gap between investment and real-world readiness
Vals.ai concludes that today's AI agents are capable of handling simple but time-consuming tasks, yet remain unreliable for use in sensitive and highly regulated sectors like finance. The models still struggle with complex, context-heavy tasks and cannot currently serve as the sole basis for decision-making.
While the models can extract basic data from documents, they fall short when deeper financial reasoning is required—making them ill-suited to fully replace human analysts.
"The data reveals a striking gap between investment & readiness. Today's agents can fetch numbers but stumble on the crucial financial reasoning needed to truly augment analyst work and unlock value in this space," the company writes.
The benchmark framework is available open source via GitHub, though the test dataset remains private to prevent targeted training. A full breakdown of the benchmark results is available on the Vals.ai website.