In Drone Racing, human pilots control miniature aircraft at breathtaking speeds through challenging courses from a first-person perspective. Now, AI is in pole position - with a clear lead and few limitations.
With Swift, researchers from the University of Zurich and Intel present an autonomous racing drone that has beaten human professionals in drone races. Swift, an AI drone equipped with cameras and other sensors, won five of nine races against Alex Vanover, four of seven against Thomas Bitmatta, and six of nine against Marvin Schaepper. Overall, Swift won 60 percent of the races.
Not all races ended in an AI victory: 40 percent of its defeats were due to collisions with opponents or gates, and 20 percent were due to slower speeds than the human pilots.
Pushing the limits, thinking ahead
Swift's victories are still remarkable. The AI drone posted the fastest overall race time, even if it wasn't faster in every section of the course, beating the next best human (A. Vanover) by half a second. The human pros had a week to practice on the track.
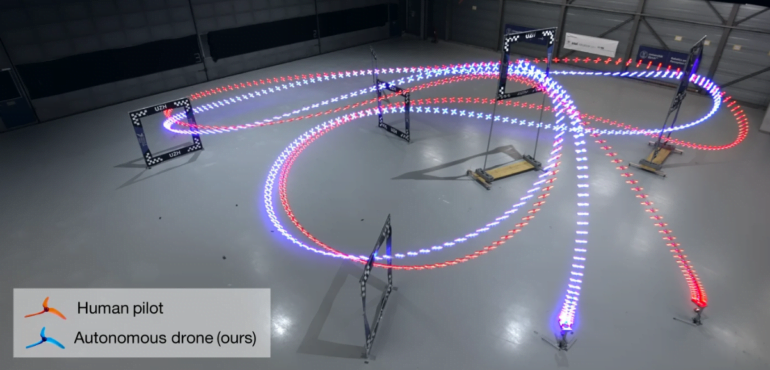
How does Swift outperform humans? For one, the drone has a faster reaction time during takeoff. It also maintains a higher speed in the first turn and flies tighter through the turns, a successful strategy that likely reflects Swift's longer-term planning.
Humans are still more robust
Swift also excels in head-to-head time trials. The AI drone is consistently trimmed for top speed. This reduces the likelihood of lap time fluctuations.
Human pilots, on the other hand, vary their lap times more because they adjust their flight strategy based on their position in the race. For example, if they are in the lead, human pilots may slow down to avoid a crash.
Swift doesn't have that luxury: The AI drone chases the best time at the highest speed, whether it's leading or trailing in the race.
Despite Swift's success, the robustness of humans is remarkable, the research team notes. Human pilots can handle an accident at full speed and continue the race as long as the drone is still intact.
They are also better able to adapt to changes in their environment, such as changes in lighting, which can confuse Swift's perception system.
AI training in a reality enhanced simulation
Swift is based on a perception system that interprets what the drone sees and "feels" through its sensors, and a control strategy that uses this information to determine the drone's next move.
The perception system is like the eyes and senses of the drone. It uses a Visual Inertial Odometry (VIO) module that combines data collected by the drone's camera with the drone's motion and inertial characteristics.
This allows it to determine where it is in the real world. In parallel, a special neural network detects the corners of the race gates based on the camera images.
It then maps these detections in 3D space and combines this information with VIO's estimates of the drone's position using a mathematical Kalman filter.
Once the drone knows where it is in the world and where the race gates are, the control strategy comes into play.
Just as a human pilot would decide whether to fly toward a goal or increase speed, the control strategy decides what the drone should do next.
The strategy, trained with deep reinforcement learning, rewards Swift for moving toward the center of the next gate while ensuring that the next gate remains in the drone's field of view.
Notwithstanding the remaining limitations and the work ahead, the attainment by an autonomous mobile robot of world-champion-level performance in a popular physical sport is a milestone for robotics and machine intelligence. This work may inspire the deployment of hybrid learning-based solutions in other physical systems, such as autonomous ground vehicles, aircraft and personal robots, across a broad range of applications.
From the paper
One of the greatest challenges for the research team was figuring out how to transfer control decisions from the initial virtual training simulation to the real world.
To address this "reality gap," the simulation was enhanced with data-driven models that take into account how the drone perceives its environment and moves in the real world. This additional fine-tuning was based on real-world data collected with the drone on the track itself. This allowed Swift to learn in simulation and from reality.