Alibaba's AI laboratory has introduced a new version of its Qwen language model that can process up to one million tokens of text—equivalent to about ten novels. The team also managed to increase processing speed by a factor of four.
Qwen has expanded its Qwen2.5 language model, introduced in September, from 128,000 to a context length of 1 million tokens. This allows Qwen2.5-Turbo to process ten complete novels, 150 hours of transcripts, or 30,000 lines of code.
100 percent accuracy in number retrieval
In the passkey retrieval task, which requires finding hidden numbers within 1 million tokens of irrelevant text, the model achieves 100 percent accuracy regardless of the information's position in the document. This appears to partially overcome the "lost in the middle" phenomenon, where language models primarily consider the beginning and end of a prompt.
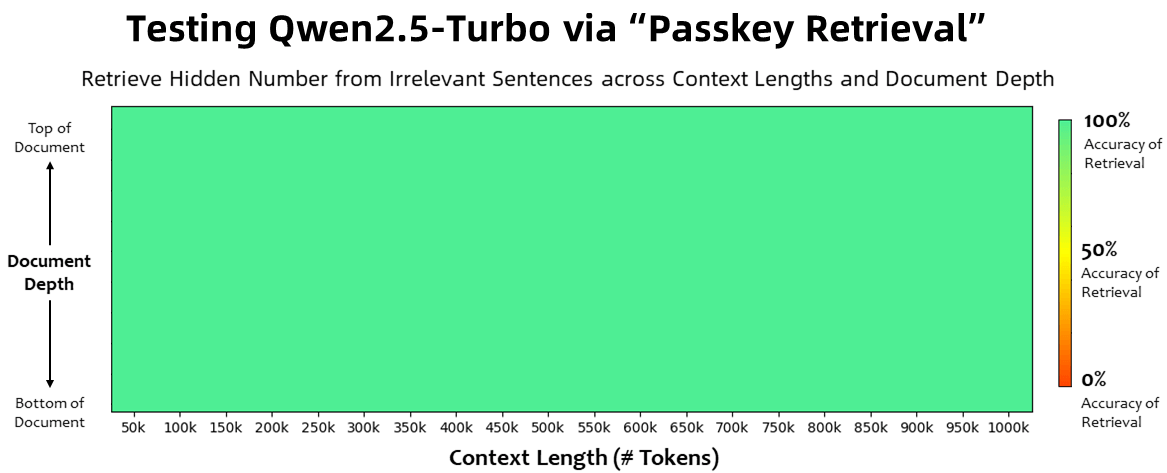
In various benchmarks for long text comprehension, Qwen2.5-Turbo outperforms competing models like GPT-4 and GLM4-9B-1M. At the same time, its performance with short sequences remains comparable to GPT-4o-mini.
In a screen recording, Qwen demonstrates its new language model's ability to quickly summarize Cixin Liu's complete "Trisolaris" trilogy, with a total length of 690,000 tokens. | Video: Qwen
Sparse attention speeds up inference by 4.3x
By using sparse attention mechanisms, Qwen reduced the time to first token when processing 1 million tokens from 4.9 minutes to 68 seconds. This represents a 4.3x speed increase.
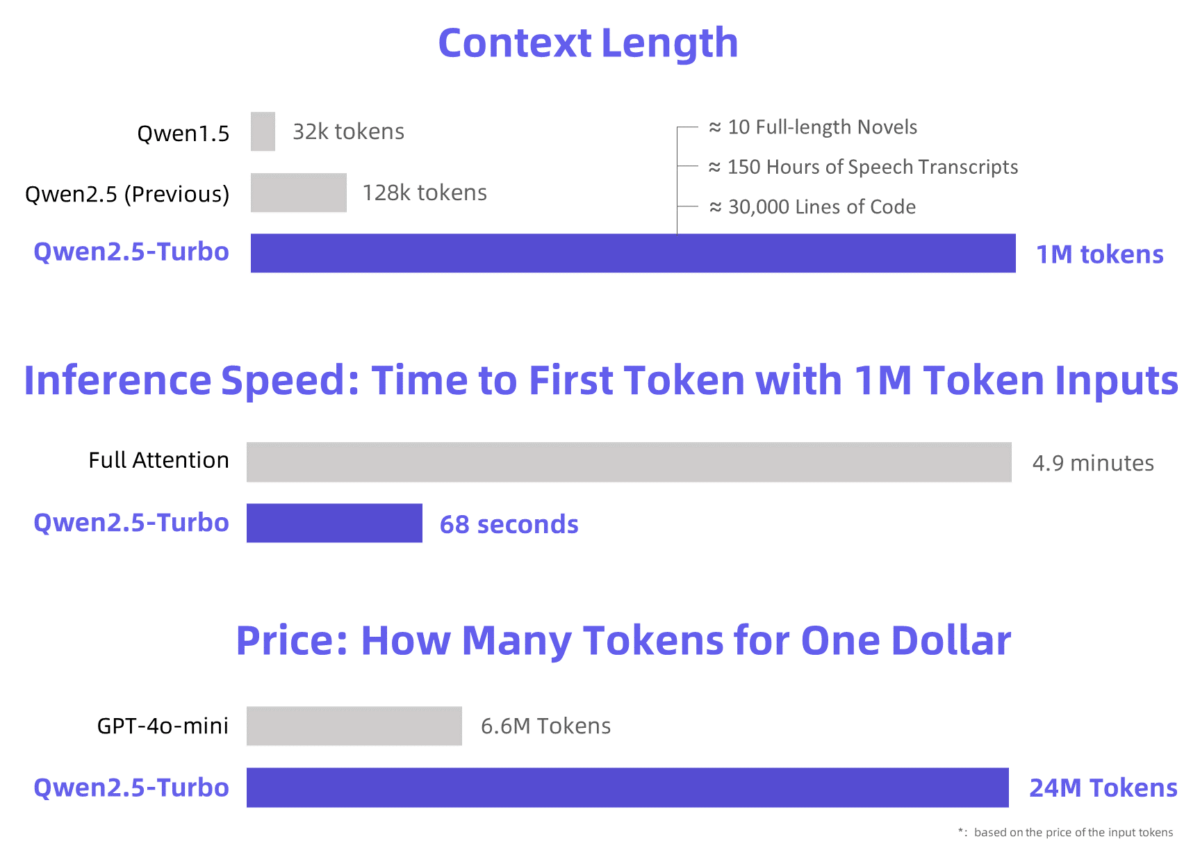
The price remains at 0.3 yuan (4 cents) per 1 million tokens. At the same cost, Qwen2.5-Turbo can process 3.6 times as many tokens as GPT-4o-mini.
Qwen2.5-Turbo is now available through Alibaba Cloud Model Studio's API and through demos on HuggingFace and ModelScope.
Qwen acknowledges room for improvement with long sequences
The company admits that the current model doesn't always perform satisfactorily when solving tasks with long sequences in real applications.
Many unsolved challenges remain, such as the model's less stable performance with long sequences and the high inference costs that make using larger models difficult.
Qwen plans to further explore human preference alignment for long sequences, optimize inference efficiency to reduce computation time, and work on bringing larger and more capable models with long context to market.
What's the use of large context windows?
The context windows of large language models have grown steadily over recent months. A practical standard has currently settled between 128,000 (GPT-4o) and 200,000 (Claude 3.5 Sonnet) tokens, though there are outliers like Gemini 1.5 Pro with up to 10 million or Magic AI's LTM-2-mini with 100 million tokens.
While these advances generally contribute to the utility of large language models, studies repeatedly cast doubt on the advantage of large context windows compared to RAG systems, where additional information is dynamically retrieved from vector databases.