Scientists at Alibaba Group have introduced VACE, a general-purpose AI model designed to handle a broad range of video generation and editing tasks within a single system.
The model’s backbone is an enhanced diffusion transformer architecture, but the headline here is the new input format: the "Video Condition Unit" (VCU). The VCU is Alibaba’s answer to the perennial mess of multimodal inputs—it takes everything from text prompts to sequences of reference images or videos, plus spatial masks, and distills them into a unified representation. The team engineered dedicated mechanisms to get these disparate inputs working together, rather than clashing with each other.
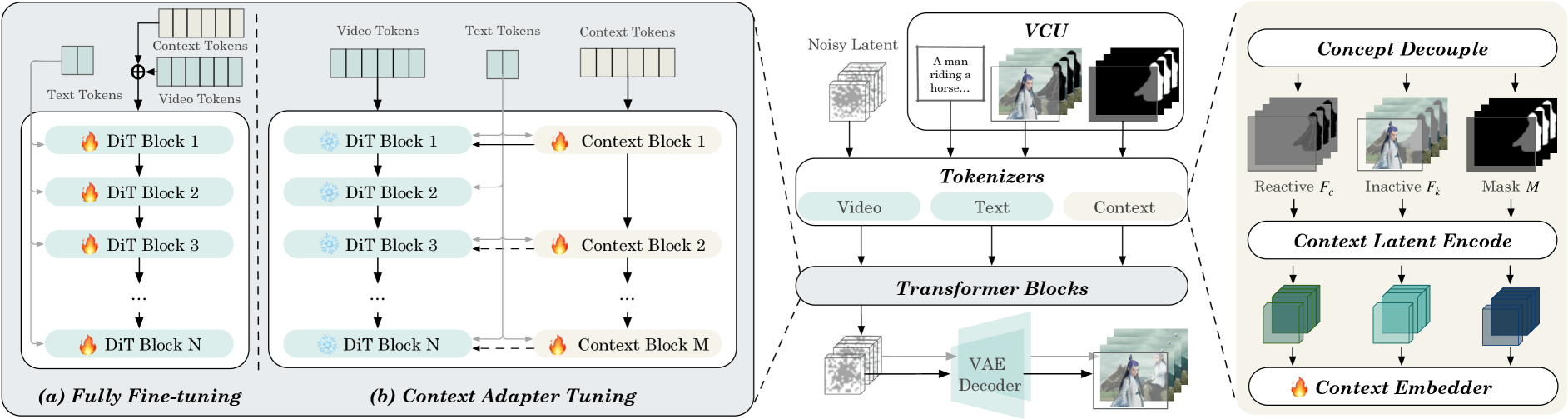
The process starts with masks dividing the image into "reactive" areas—targets for modification—and "inactive" zones that are left untouched. All of this visual information is embedded into a shared feature space and combined with the corresponding text input.
To keep the generated video consistent from frame to frame, VACE maps these features into a latent space built to match the structure of the diffusion transformer. Time-embedding layers ensure that the model’s understanding of the sequence doesn’t fall apart as it moves through each frame. An attention mechanism then ties together features from different modalities and timesteps, so the system can handle everything as a cohesive whole—whether it’s producing new video content or editing existing footage.
Text-to-video, reference-to-video and video editing
VACE’s toolkit covers four core tasks: generating videos from text prompts, synthesizing new footage based on reference images or clips, editing video-to-video, and applying masks for targeted edits. This one-model-fits-most approach unlocks a pretty broad set of use cases.
In practice, the demos are all over the map—VACE can animate a person walking out of frame, conjure up an anime character surfing, swap penguins for kittens, or expand a background to keep things visually seamless. If you want to see the breadth of what it can do, there are more examples on the project’s official website.
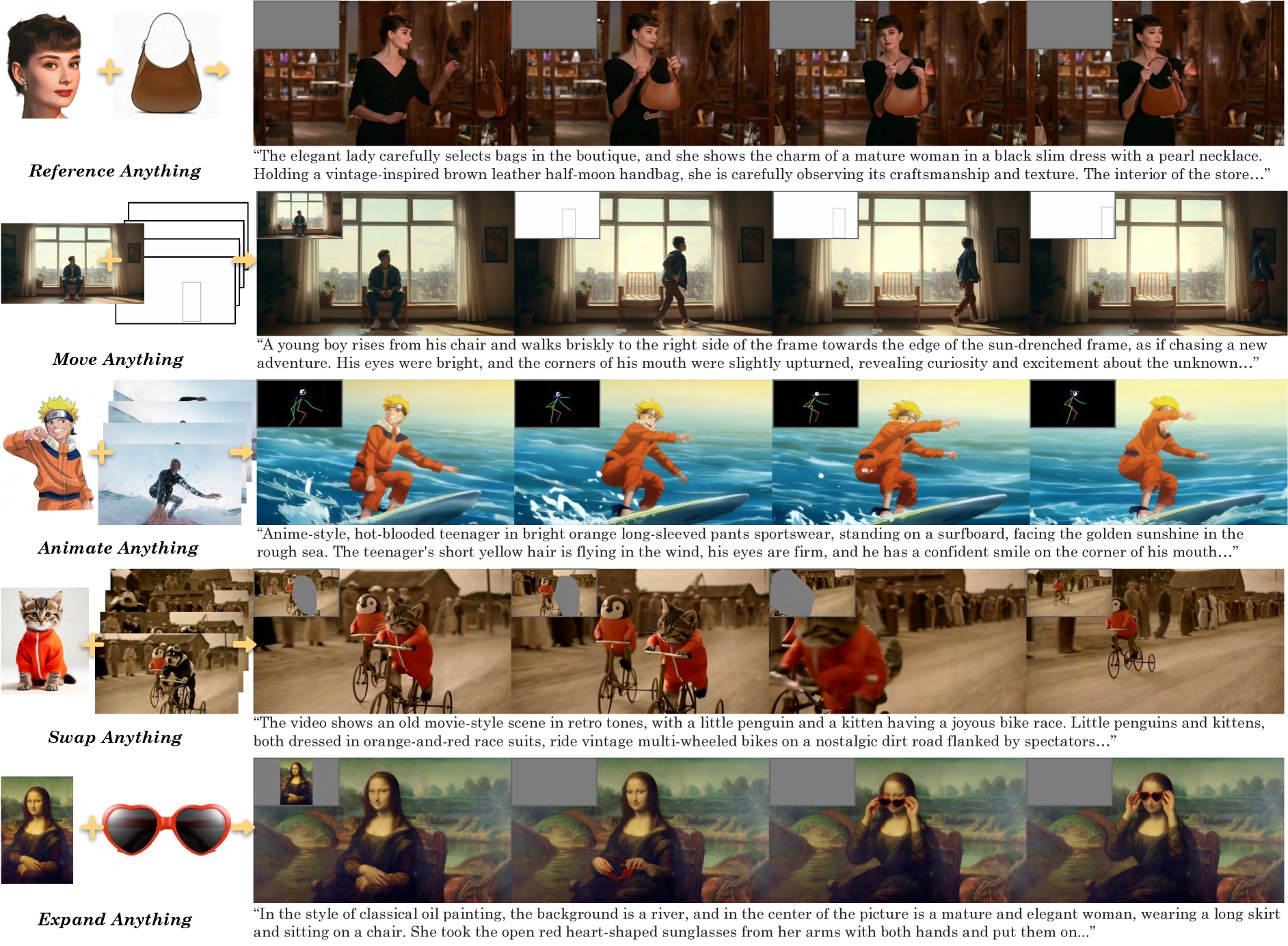
Training started with the basics: the team focused first on inpainting and outpainting to shore up the text-to-video pipeline, then layered in reference images and moved on to more advanced editing tasks. For data, they pulled from internet videos—automatically filtering, segmenting, and enriching them with depth and pose annotations.
Benchmarking VACE across twelve video editing tasks
To actually measure how VACE stacks up, the researchers put together a dedicated benchmark: 480 examples covering a dozen video editing tasks, including inpainting, outpainting, stylization, depth control, and reference-guided generation. According to their results, VACE outperforms specialized open-source models across the board in both quantitative metrics and user studies. That said, there’s still a gap on reference-to-video generation, where commercial models like Vidu and Kling have the edge.
Alibaba’s researchers pitch VACE as an important step towards universal, multimodal video models, and the next move is pretty predictable—scaling up with bigger datasets and more compute. Some parts of the model are set to land as an open-source release on GitHub.
VACE fits into the bigger picture of Alibaba’s AI ambitions, alongside a string of recent large language model releases—especially the Qwen series. Other Chinese tech giants like ByteDance are pushing hard on video AI as well, sometimes matching or beating Western offerings like OpenAI’s Sora or Google's Veo 2.