Apple MM1 is a capable multimodal AI model that can compete with GPT-4V and Google Gemini in visual tasks thanks to its intelligent architecture and sophisticated training.
Like GPT-4V and Gemini, MM1 is based on the Large Language Model (LLM) architecture and was trained on a mixture of image-text pairs, interleaved image-text documents, and text-only data (45% image-text pairs, 45% interleaved image-text documents, 10% text-only data).
This training regimen has enabled MM1 to develop capabilities similar to its rivals, including image description, question answering, and even basic mathematical problem-solving.
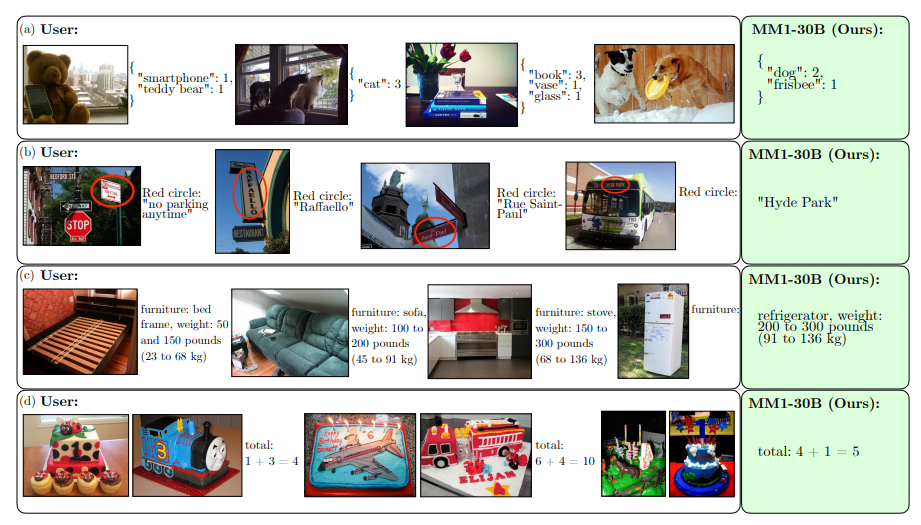
Apple's researchers conducted in-depth investigations to identify the factors that most significantly influence MM1's performance, such as architectural components and training data.
They discovered that high image resolution, the performance of the image processing component (known as the "visual encoder"), and the volume of training data are particularly crucial. Interestingly, the link between image and language was found to be less critical.
The visual encoder is tasked with converting image information into a format that the AI system can process. The more advanced this encoder is, the better MM1 can understand and interpret image content.
The study also highlights the importance of the right mix of training data. Image-text pairs, interleaved image-text data, and text-only data were essential for achieving strong results with limited examples in the input prompt. However, when MM1 had to generate outputs without examples in the prompt, image-text pairs in the training data played a more significant role.
Image-text pairs or image-caption pairs are data in which each image is directly paired with an associated text. This text is usually a description or explanation of the image content.
An example would be an image of a dog with the caption "A brown dog playing with a ball in the park". Such paired data is often used to train models for tasks such as automatic image labeling.
Interleaved image-text data, on the other hand, is data in which images and text appear in a mixed order, without each image necessarily being directly associated with a specific text.
An example would be a news article that consists of a mixture of images and text sections that relate to the same topic, but are not necessarily in a 1:1 relationship. Such data tends to reflect the way visual and textual information often occur together in natural contexts.
In the context of the paper, it has been shown that a mixture of both types of data - i.e. both image-text pairs and mixed image-text data - together with text-only data is beneficial for training multimodal AI models, especially when it comes to achieving good results with few examples (Few-Shot Learning).
30 billion parameters can be enough for state-of-the-art results
By scaling up to 30 billion parameters and using Mixture-of-Experts (MoE) models, a special technique in which multiple specialized AI models work together, MM1 has achieved state-of-the-art results, outperforming most published models in few-shot learning for image captioning and visual question answering.
MM1 also excels in more complex scenarios, such as multi-image reasoning, where it can combine information from multiple images to answer complex questions or draw conclusions that cannot be inferred from a single image. This could help MM1 understand and interpret the real world in a way that closely resembles human perception and reasoning.
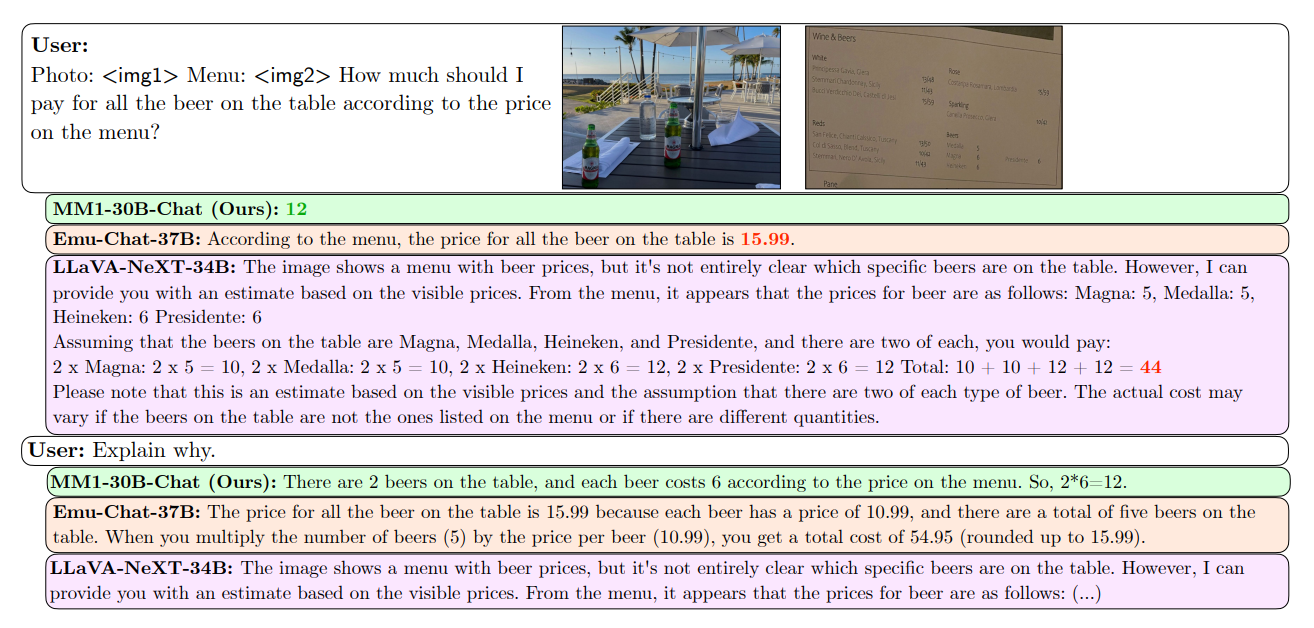
After further refining the model through supervised fine-tuning (SFT) using selected data, MM1 achieved competitive results on twelve established benchmarks. This positions MM1, or a scaled-up variant, as a potential serious competitor to other leading AI systems such as GPT-4V and Google Gemini in the near future.
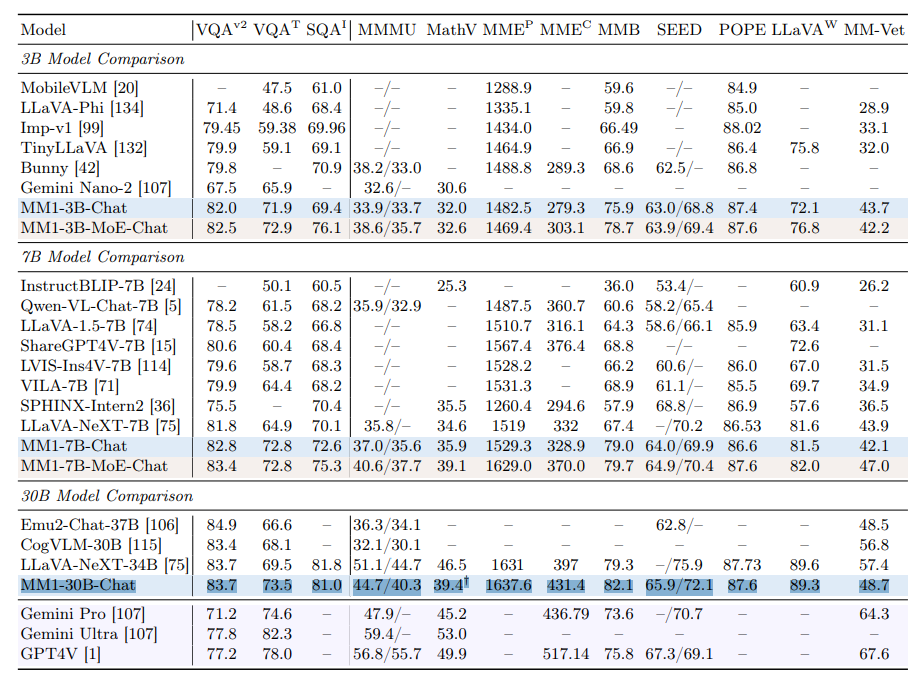