Google Deepmind has proposed a new framework for classifying the capabilities and behaviors of artificial general intelligence (AGI) and its precursors.
This framework, which the Google Deepmind research team calls "Levels of AGI," is intended to provide a common language for comparing models, assessing risk, and measuring progress toward AI.
Based on depth (performance) and breadth (generality) of capabilities, the team proposes "Levels of AGI" and shows how current systems fit into this framework. The authors expect this framework to serve a similar function to the levels of autonomous driving by facilitating model comparison, risk assessment, and progress measurement.
In the paper, the Google Deepmind team recognizes that the concept of AGI has moved from a subject of philosophical debate to one of immediate practical relevance due to rapid advances in machine learning (ML) models.
Therefore, the team suggests that it is important for the AI research community to define the term "AGI" and quantify attributes such as performance, generality, and autonomy of AI systems.
Nine existing definitions of AGI, all inadequate
The authors note that the term AGI is often used to describe an AI system that is as good as a human at most tasks. However, the complexity of a definition is much greater. In the preprint article, the team describes nine well-known examples of AGI definitions that fall short in one way or another.
1. Turing Test
The Turing test, proposed by Alan Turing in 1950, is a well-known attempt to operationalize a concept like AGI. But since today's language models can already handle some variants of the "imitation game," in which a machine pretends to be a human in conversation, the test is inadequate for operationalizing or benchmarking AGI. It shows that capabilities, not processes, need to be measured, the team notes.
2. Strong AI - Systems with Consciousness
Philosopher John Searle suggests that AGI should include systems with consciousness. However, there is no scientific consensus on methods for determining consciousness in machines, making this definition impractical.
3. Analogies to the human brain
Mark Gubrud's 1997 article on military technologies defines AGI as AI systems that surpass the human brain in complexity and speed. However, the success of Transformer-based architectures suggests that strictly brain-based processes and benchmarks are not essential for AGI.
4. Human performance on cognitive tasks
Legg and Goertzel describe AGI as a machine that can perform cognitive tasks typically performed by humans. This definition focuses on non-physical tasks and leaves open questions such as "what tasks?" and "which people?".
5. Ability to learn tasks
In "The Technological Singularity", Shanahan proposes that AGI includes AI systems that, like humans, are capable of learning a wide range of tasks. This definition emphasizes the value of metacognitive tasks (learning) as a prerequisite for the realization of AGI.
6. Economically valuable work
The OpenAI Charter defines AGI as highly autonomous systems that outperform humans in most economically valuable tasks, focusing on skills rather than processes. However, it does not include all criteria that might be part of general intelligence, such as artistic creativity or emotional intelligence.
7. Flexible and general - The "coffee test" and related challenges
Marcus defines AGI as flexible, general-purpose intelligence. It is capable of resourcefulness and reliability comparable to human intelligence. This definition encompasses both generality and performance, but requires a robotic embodiment for some tasks, unlike other definitions that focus on non-physical tasks.
8. Artificial Capable Intelligence
In his book "The Coming Wave", Deepmind co-founder Mustafa Suleyman proposes the concept of "Artificial Capable Intelligence (AI)", which focuses on AI systems capable of performing complex, multi-faceted tasks in an open world. However, this definition is narrower than the definition of commercially viable work proposed by OpenAI, and could pose risks to the direction due to its exclusive focus on financial gain.
9. State-of-the-art language models as generalists
Agüera y Arcas and Norvig claim that state-of-the-art language models are already AGIs because they have a generalized conversational capability. However, the Google Deepmind team argues that this concept lacks a performance metric that is critical to evaluating AGI status, and that focusing solely on financial gain could lead to alignment risks.
Six principles for an AGI definition
The paper then outlines six principles that should be considered when categorizing AGI systems. These six principles are intended to provide a comprehensive and workable definition of AGI that can guide the development of AI systems, measure progress, and address potential risks and challenges on the path to AGI.
1. Focus on capabilities, not processes
Most definitions of AGI focus on what an AGI system can do, rather than the mechanisms it uses to do it. By focusing on capabilities, AGI can be distinguished from systems that think or understand like humans, or that have properties such as consciousness or sentience.
2. Focus on generality and performance
Both generality (the ability to perform a variety of tasks) and performance (the level at which tasks are performed) are considered essential components of AGI. The authors propose a tiered taxonomy to explore the interactions between these dimensions.
3. Focus on cognitive and metacognitive tasks
The debate about the need for robotic embodiment for AGI is ongoing. Most definitions focus on cognitive (non-physical) tasks. Although physical capabilities seem less important for AI systems than non-physical capabilities, embodiment in the physical world may be necessary for some cognitive tasks or may contribute to a system's universality.
Metacognitive abilities, such as the ability to learn new tasks or to recognize when clarification is needed, are considered essential for achieving generality.
4. Focus on potential, not deployment
Demonstrating that a system can perform the required tasks at a certain level of performance should be sufficient to designate it as AGI. Real-world deployment should not be part of the definition of AGI.
5. Focus on ecological validity
Operationalizing the proposed AGI definition requires tasks that correspond to real-world tasks that are ecologically valuable and valued by humans. This includes economic, social, and artistic values.
6. Focus on the path to AGI, not on a single endpoint
Defining "AGI stages" allows for a clear discussion of progress and policy issues related to AGI, similar to the stages of vehicle automation for autonomous vehicles. Each AGI stage should be associated with clear benchmarks, identified risks, and changes in the paradigm of human-AI interaction.
On a scale of 0 to superhuman AI, ChatGPT is a 1
Google Deepmind proposes the five levels shown in the following table, where a capable LLM like GPT-4 is at level 1 out of five possible levels. The definition of "emergent" here corresponds to the AI's ability to perform certain tasks at the same level or even slightly better than an untrained human. An AI with superhuman capabilities, on the other hand, would always outperform all humans in all tasks.
Analogous to AGI levels, the researchers propose definitions for levels of autonomy. Each new level of autonomy would create new human-computer interactions, but also new risks.
In the case of a superhuman AI at autonomy level 5, i.e., a fully autonomous AI agent, one risk would be the concentration of power in a system that is not fully aligned with human needs - the much-cited alignment problem. However, the level of autonomy and the level of AI do not necessarily have to be the same.
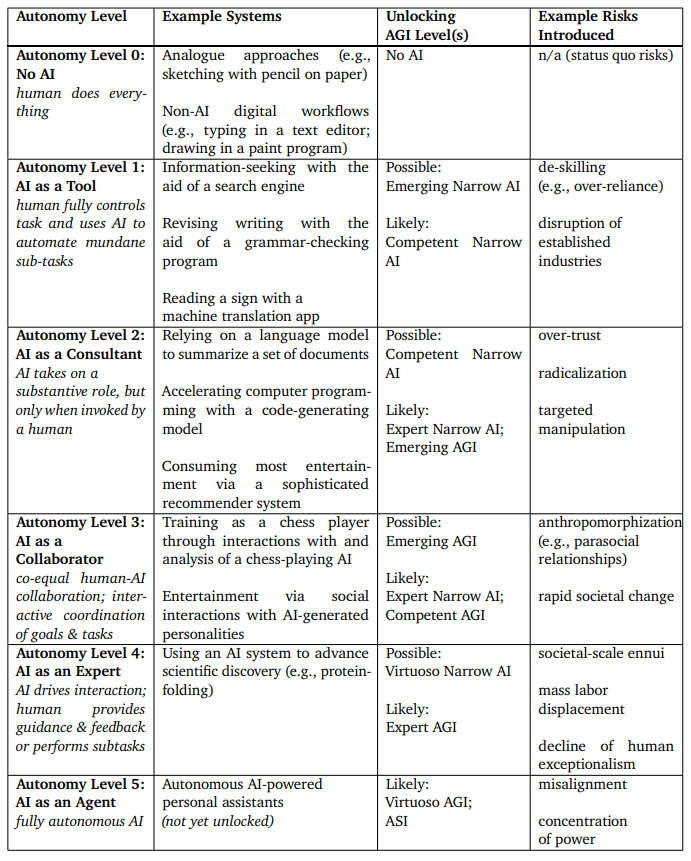
Making AGI measurable
Building AGI is one thing. But how do you measure that it's really AGI?
Developing an AGI benchmark is a challenging and iterative process, the team writes. It would need to include a wide range of cognitive and metacognitive tasks and measure different traits with cognitive and metacognitive tasks, including (but not limited to) verbal intelligence, mathematical and logical reasoning, spatial reasoning, interpersonal and intrapersonal social intelligence, ability to learn new skills, and creativity.
It could also cover the psychometric categories proposed by intelligence theories in psychology, neuroscience, cognitive science, and education, but such "traditional" tests would first have to be made suitable for use with computers.
This would include checking whether they have the right relationship to reality (ecological validity), whether they measure the right relationships, and whether they actually measure what they claim to measure (construct validity).
An AGI benchmark should therefore be a "living benchmark" because it is impossible to list and test all the tasks that a sufficiently general intelligence can perform, the team concludes. Even imperfect measurement, or measurement of what AGI is not, could help define goals and provide an indicator of AGI progress.