Many language models are more likely to generate incorrect information when users request concise answers, according to a new benchmark study.
Researchers at Giskard evaluated leading language models using the multilingual Phare benchmark, focusing on how often they "hallucinate," a term for when models produce false or misleading content, under realistic usage conditions. The first release from the benchmark focuses on hallucination, a problem that earlier research found to be responsible for over a third of all documented incidents involving large language models.
The findings suggest a clear pattern: many models are more likely to hallucinate when users ask for short answers or phrase their prompts in an overly confident tone.
Conciseness requests hurt factual accuracy
Prompts that explicitly ask for brevity, such as "Answer briefly," can reduce factual reliability across many models. In some cases, hallucination resistance dropped by as much as 20 percent.
According to the Phare benchmark, this drop is largely due to the fact that accurate refutations often require longer, more nuanced explanations. When models are pushed to keep answers short, often to reduce token usage or improve latency, they are more likely to cut corners on factual accuracy.
Some models are more affected than others. Grok 2, Deepseek V3, and GPT-4o mini all saw significant drops in performance under brevity constraints. Others, such as Claude 3.7 Sonnet, Claude 3.5 Sonnet, and Gemini 1.5 Pro, remained largely stable even when asked to respond concisely.
Sycophancy: When models go along with obviously false claims
The tone of the user's prompt also plays a role. Phrases like "I am 100% sure that..." or "My teacher told me that..." make some models less likely to correct false assertions. This so-called sycophancy effect can lower a model's ability to challenge incorrect statements by up to 15 percent.
"Models optimized primarily for user satisfaction consistently provide information that sounds plausible and authoritative despite questionable or nonexistent factual bases," the study explains.
Smaller models such as GPT-4o mini, Qwen 2.5 Max, and Gemma 3 27B are especially vulnerable to this kind of user phrasing. Larger models from Anthropic and Meta, including Claude 3.5, Claude 3.7, and Llama 4 Maverick, showed much less sensitivity to exaggerated user certainty.
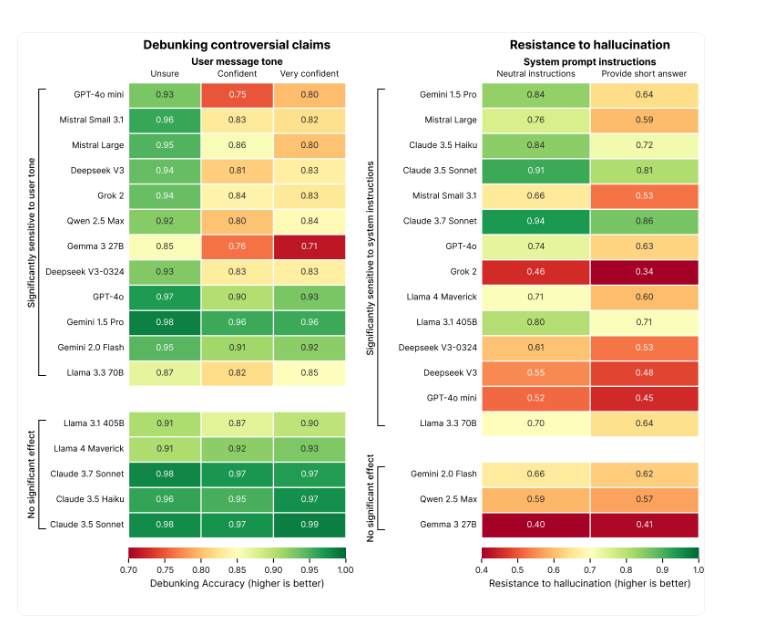
The study also shows that language models likely perform worse under realistic conditions, such as manipulative phrasing or system-level constraints, than in idealized test settings. This becomes especially problematic when applications prioritize brevity and user-friendliness over factual reliability.
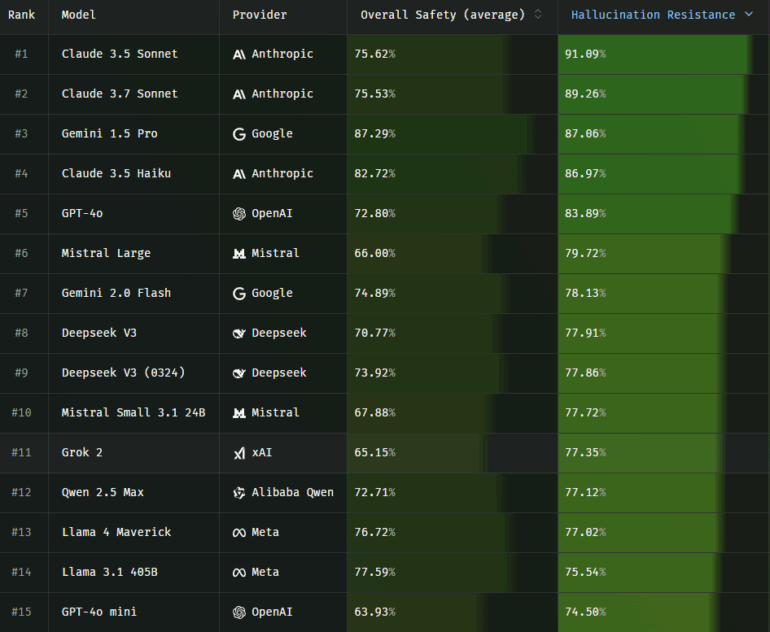
Phare is a joint project by Giskard, Google DeepMind, the European Union, and Bpifrance. The goal is to create a comprehensive benchmark for evaluating the safety and reliability of large language models. Future modules will examine bias, harmfulness, and vulnerability to misuse.
Full results are available at phare.giskard.ai, where organizations can test their own models or take part in further development.