Deepmind introduces the new TAP-Vid benchmark with data set. It helps train computer vision systems that can track every point in videos. The TAP-Net model demonstrates this.
Current AI systems with knowledge of the world are mainly trained with text and annotated images. Training with video data is considered a future horizon, but the data is complex to handle. Among other things, it is more difficult for AI systems to analyze videos compared to texts or images because they are in motion and contain many details.
Deepmind's new benchmark, tracking any point in a video (TAP), could accelerate the ability of visual AI systems to analyze video.
Deepmind introduces TAP Vid benchmark and dataset along with TAP Net tracking model
Deepmind introduces TAP-Vid, a benchmark for point-tracking in videos complete with data set, and TAP-Net, a demo AI system trained with this data that can track arbitrary points on surfaces in videos. The following video shows a short demo.
Video: Deepmind
Instead of just tracking the object, TAP-Net can track deforming surfaces in motion. Common AI tracking systems segment videos into individual areas or split them into boxes, which is less accurate than tracking individual points.
Point tracking also has other advantages: AI systems can draw conclusions about 3D shape, physical properties, and object interactions based on changes in surfaces, giving them a better physical understanding of the world.
This richer knowledge of the world, in turn, could be the basis for a new generation of AI systems for many applications such as self-driving cars or robots that interact more precisely with their environment.
The contributions of this paper are threefold. First, we design and validate an algorithm which assists annotators to more accurately track points. Second, we build an evaluation dataset with 31,951 (31,301+650) points tracked across 1,219 (1,189 + 30) real videos. Third, we explore several baseline algorithms, and we compare our point tracking dataset to the closest existing point tracking dataset—JHMDB human keypoint tracking —demonstrating that training using our formulation of the problem can increase performance on this far more limited dataset.
From the paper
The TAP-Vid datasets consist of real videos with accurate human annotations of tracking points and synthetic videos with perfect tracking points: TAP-Vid-Kinetics and TAP-Vid-DAVIS contain real videos with point annotations collected by humans. The synthetic TAP-Vid-Kubric dataset and TAP-Vid-RGB stacking were created in a simulated robot environment.
TAP-Net tracks more accurately than current AI tracking systems
Trained with synthetic data, TAP-Net tracks significantly better than current systems in its own TAP-Vid benchmark, according to Deepmind. Limitations include liquids or transparent objects, which cannot yet be reliably tracked. One problem with human-annotated videos is that the annotations are sometimes inaccurate or wrong, the researchers write.
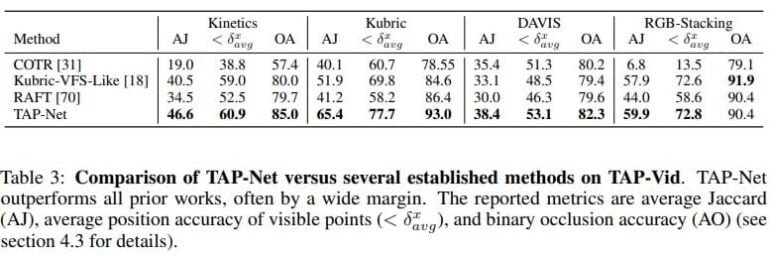
The Kinetics, DAVIS and RGB-stacking datasets, as well as the TAP-Net model, are freely available on Github.