Google DeepMind has demonstrated how robots can navigate complex environments using Gemini 1.5 Pro's large context window and multimodal input capabilities.
The researchers used Gemini 1.5 Pro's ability to process up to a million multimodal tokens to enable robots to navigate unfamiliar spaces using only human instructions, video guidance, and model reasoning.
In one experiment, scientists guided robots through specific areas of a real-world environment, showing them important locations such as "Lewi's desk" or the "temporary desk area. The robots were then able to find their way back to these locations on their own.
The "demonstration video" that gives the robot an overview of the environment can be easily recorded with a smartphone.
Video: Google Deepmind
This approach even works for small objects. A user could show the robot a video tour of their home and later ask via smartphone, "Where did I leave my coaster?" The robot would then navigate to the location on its own.
Thanks to the built-in language model, the robot can also make abstractions. If a user asks for a place to draw, the robot can associate that with a whiteboard and take the user there.
Video: Google Deepmind
Google DeepMind suggests that in the future, a robot could infer a user's preferences from audiovisual information and act accordingly. For example, if a user has many cans of a particular brand of soda on their desk, the robot could preferentially retrieve that drink from the fridge. Such capabilities could greatly enhance human-robot interaction.
The system architecture processes the multimodal input to create a topological graph – a simplified representation of the room. This graph is constructed from individual images of the video tours and captures the general connectivity of the environment, allowing the robot to navigate without a detailed map.
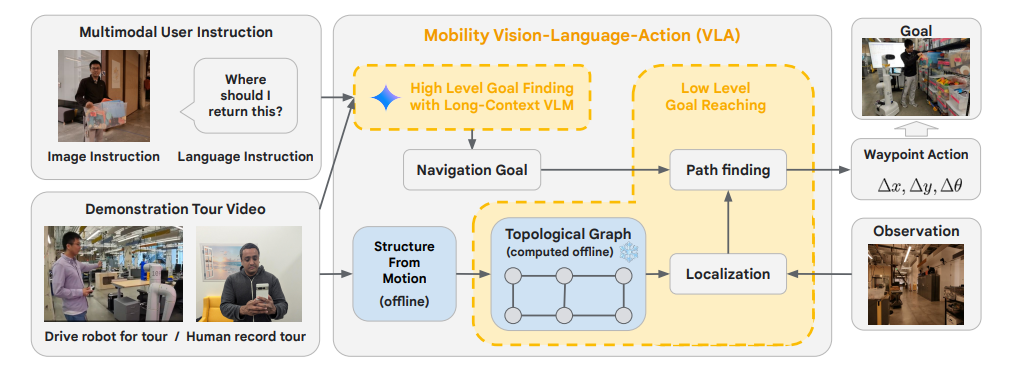
In further tests, the robots received additional multimodal instructions, such as map sketches on a whiteboard, audio prompts related to locations on the tour, and visual cues such as a toy box. With these inputs, the robots were able to perform different tasks for different people.
In 57 tests in a real office environment of 836 square meters, Mobility VLA achieved success rates of up to 90 percent in performing various multimodal navigation tasks. For complex instructions requiring reasoning, it achieved a success rate of 86 percent, compared to 60 percent for a text-based system and 33 percent for a CLIP-based approach.

Despite the promising results, the researchers point out some limitations. For example, the system currently takes 10 to 30 seconds to process a command, resulting in delays in interaction. It also cannot explore the environment on its own, relying instead on the demonstration video provided.
Google Deepmind plans to extend the Mobility VLA to other robot platforms and expand the system's capabilities beyond navigation. Preliminary tests indicate that the system could also perform more complex tasks, such as inspecting objects and reporting on the results.