A new survey paper provides an in-depth look at the methods, datasets, and applications of how artificial intelligence could fundamentally change 3D development.
3D modeling has gained many new capabilities through the use of neural representations and generative AI models.
A new survey paper provides a structured insight into the underlying methods, datasets, and applications in the field of AI for 3D content generation and editing.
What's striking is the enormous variety and complexity of methods and techniques that have emerged in a very short time. The authors speak of "explosive growth," with new developments occurring on a weekly or even daily basis.
They divide the field into four main areas:
- 3D representations,
- generation methods,
- datasets
- and various applications.
According to the authors, 3D generation can be useful in nearly all areas, in obvious applications such as games and virtual reality, but also in film or robotics.
Applications range from the generation of virtual people and realistic faces to individual 3D objects or entire 3D scenes.
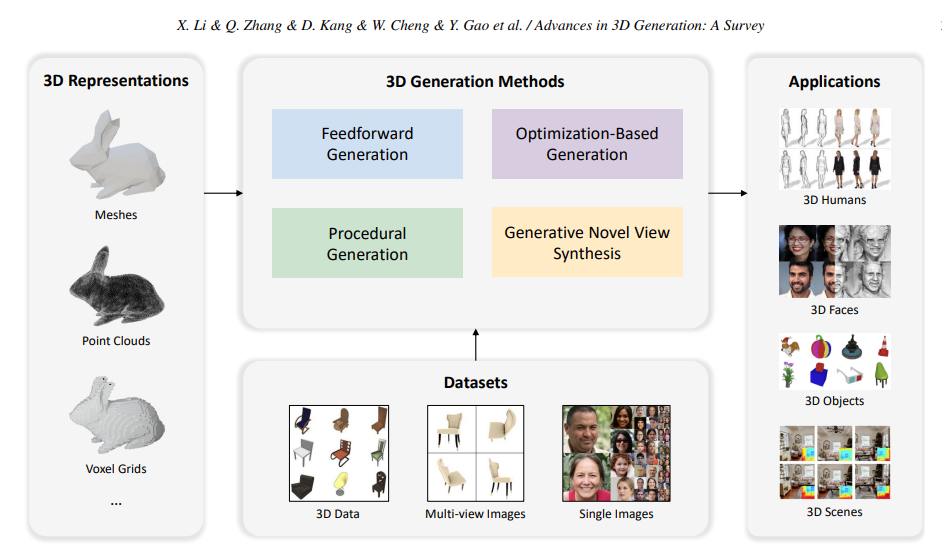
In addition to 3D generation, the paper also discusses 3D editing tasks, which can be divided into global and local editing.
Global editing aims to change the appearance or geometry of the entire 3D scene. Local editing focuses on changing a specific area of a scene or object.
Examples of global editing tasks include stylizing and manipulating individual objects, while local editing tasks include manipulating appearance, deforming geometry, and duplicating/deleting objects.
3D data is diverse and sparse, slowing generative AI
The study also discusses the datasets available for 3D generation. In particular, the authors emphasize the importance of large and diverse datasets for the development of AI-based 3D generation models.
Although numerous datasets already exist that can be used to train 3D generation models, they are often limited to specific applications or domains, or are simply small.
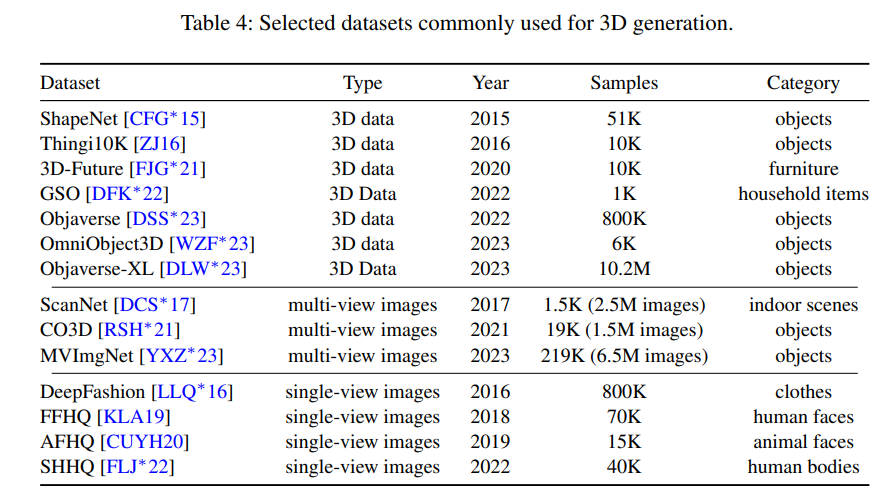
One reason is that 3D data is not as easy to capture as text or images. 3D artists and designers spend a lot of time creating high-quality 3D content, the researchers write.
In addition, 3D data can vary widely in scale, quality, and style, adding to the complexity of data capture. Standardizing disparate 3D data would require a new set of rules.
In addition to creating large, high-quality 3D datasets, other challenges include developing better metrics to objectively evaluate the quality and variety of models generated, and using 2D data for 3D generation.