A new study of how language models evaluate each other has uncovered a troubling pattern: as these systems become more sophisticated, they're increasingly likely to share the same blind spots.
Researchers from institutions in Tübingen, Hyderabad, and Stanford have developed a new measurement tool called CAPA (Chance Adjusted Probabilistic Agreement) to track how language models overlap in their errors beyond what you'd expect from their accuracy rates alone. Their findings suggest language models tend to favor other LLMs that make mistakes similar to their own.
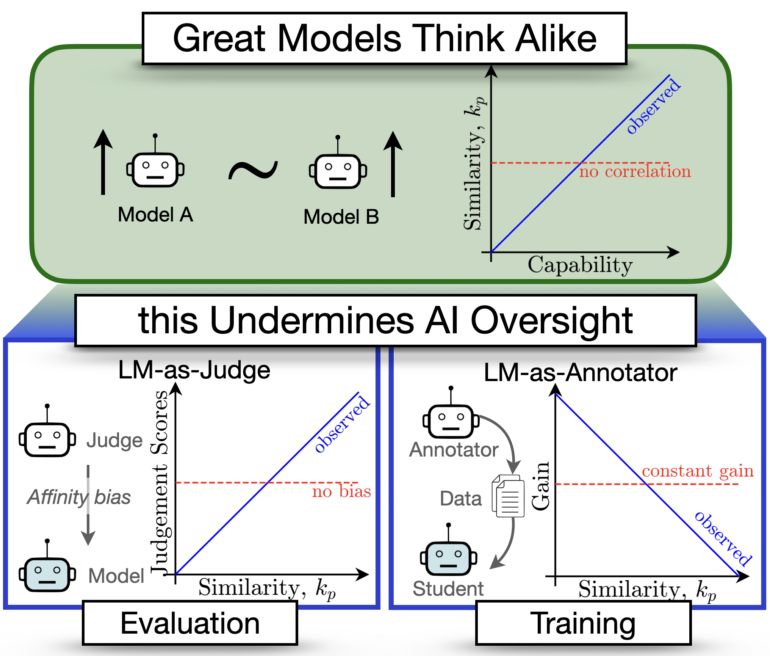
When language models were tasked with judging other models' output, they consistently gave better scores to systems that shared their error patterns, even after accounting for actual performance differences. The researchers compare this behavior to "affinity bias" in human hiring, where interviewers unconsciously favor candidates who remind them of themselves.
The team also explored what happens when stronger models learn from content generated by weaker ones. They discovered that greater differences between models led to better learning outcomes, likely because dissimilar models possess complementary knowledge. This finding helps explain why performance gains in "weak-to-strong" training approaches vary across different tasks.
Common blind spots and failure modes
After analyzing more than 130 language models, the researchers identified a concerning pattern: as models become more capable, their errors grow increasingly alike. This trend raises safety concerns, especially as AI systems take on more responsibility for evaluating and controlling other AI systems.
"Our results indicate a risk of common blind-spots and failure modes when using AI oversight, which is concerning for safety," the researchers write.
The research team emphasizes the importance of paying attention to both model similarity and error diversity, noting that further research is needed to extend their metric to evaluate free-text responses and the reasoning abilities of large language models.