OpenAI is expanding its custom AI training offerings with a new method called Reinforcement Fine-Tuning (RFT). The technique aims to create specialized o1 models that can perform complex technical tasks with minimal training examples.
The new approach works differently from traditional supervised fine-tuning. Instead of just learning to copy the style and tone of training data, models can develop new ways of "thinking" through problems, according to OpenAI. When given a problem, the model gets time to work out a solution o1 style. An evaluation system then rates the answer - strengthening successful reasoning patterns while weakening incorrect ones.
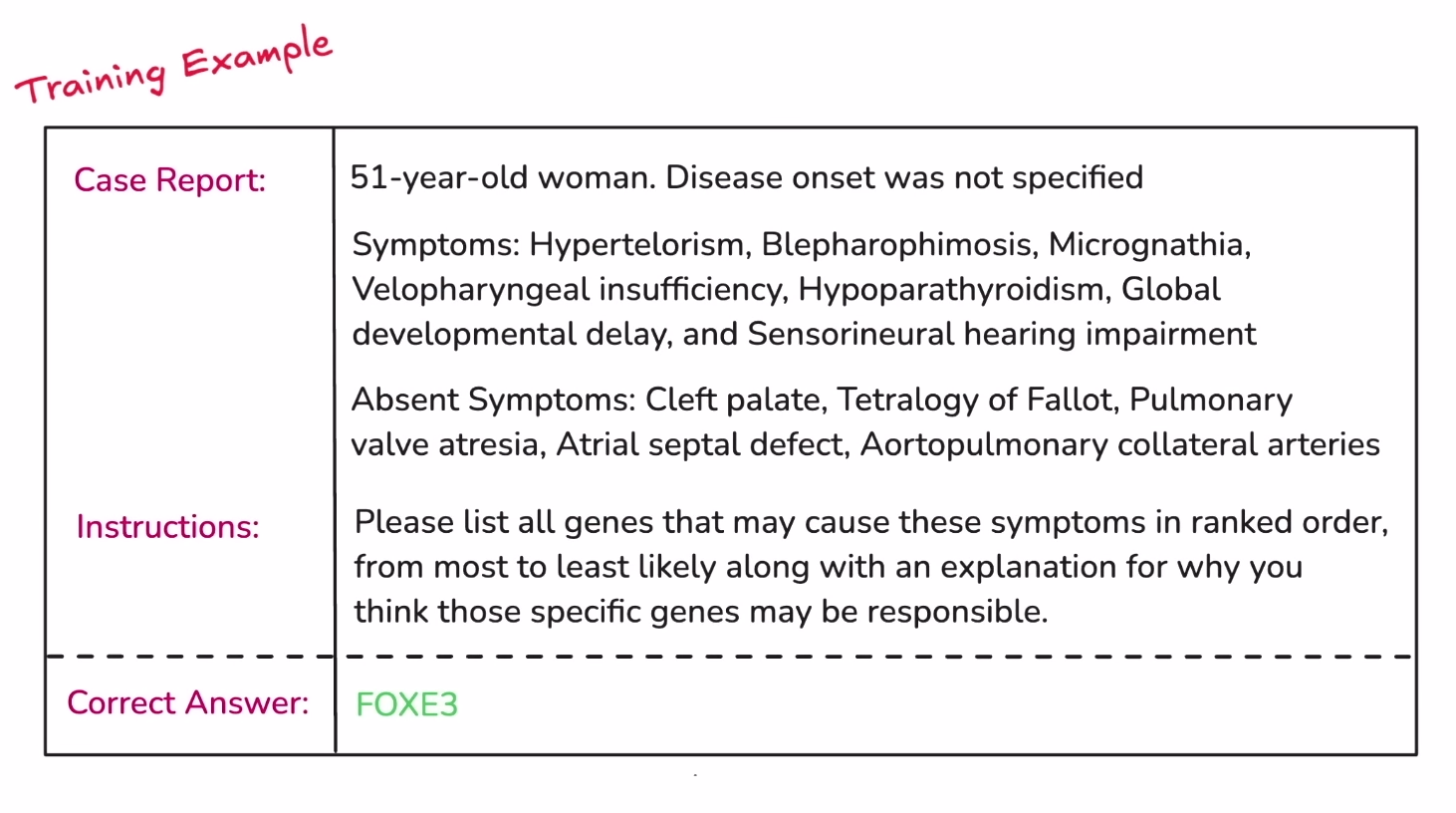
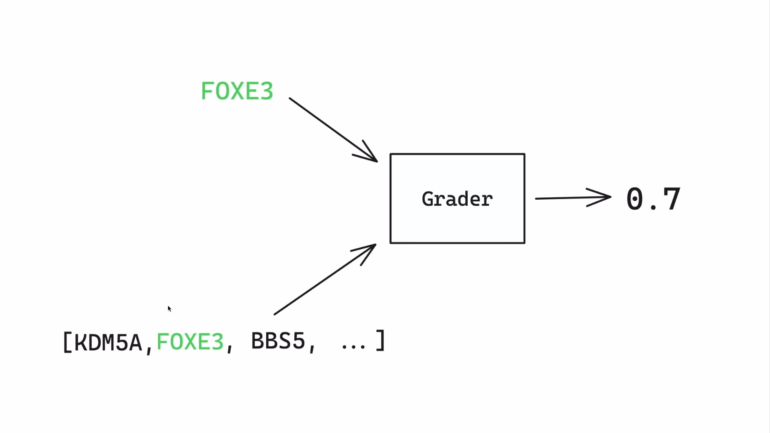
OpenAI says this approach works especially well for specialized fields like law, finance, engineering, and insurance that need deep technical knowledge. As an example, the company highlights its collaboration with Thomson Reuters, where they trained the compact o1 Mini model to work as a legal assistant.
Reinforcement learning for expert systems
Justin Ree, a bioinformatician at Berkeley Lab, used RFT to study rare genetic diseases. He trained the system using data extracted from hundreds of scientific papers that included symptoms and their associated genes.
Ree reports that the RFT-trained o1 Mini outperformed the standard o1 model at this task, despite being smaller and less expensive. He notes that the model's ability to explain its predictions makes it particularly useful.
Testing shows the fine-tuned mini model achieves the highest precision in gene identification, reaching up to 45 percent accuracy at maximum range.
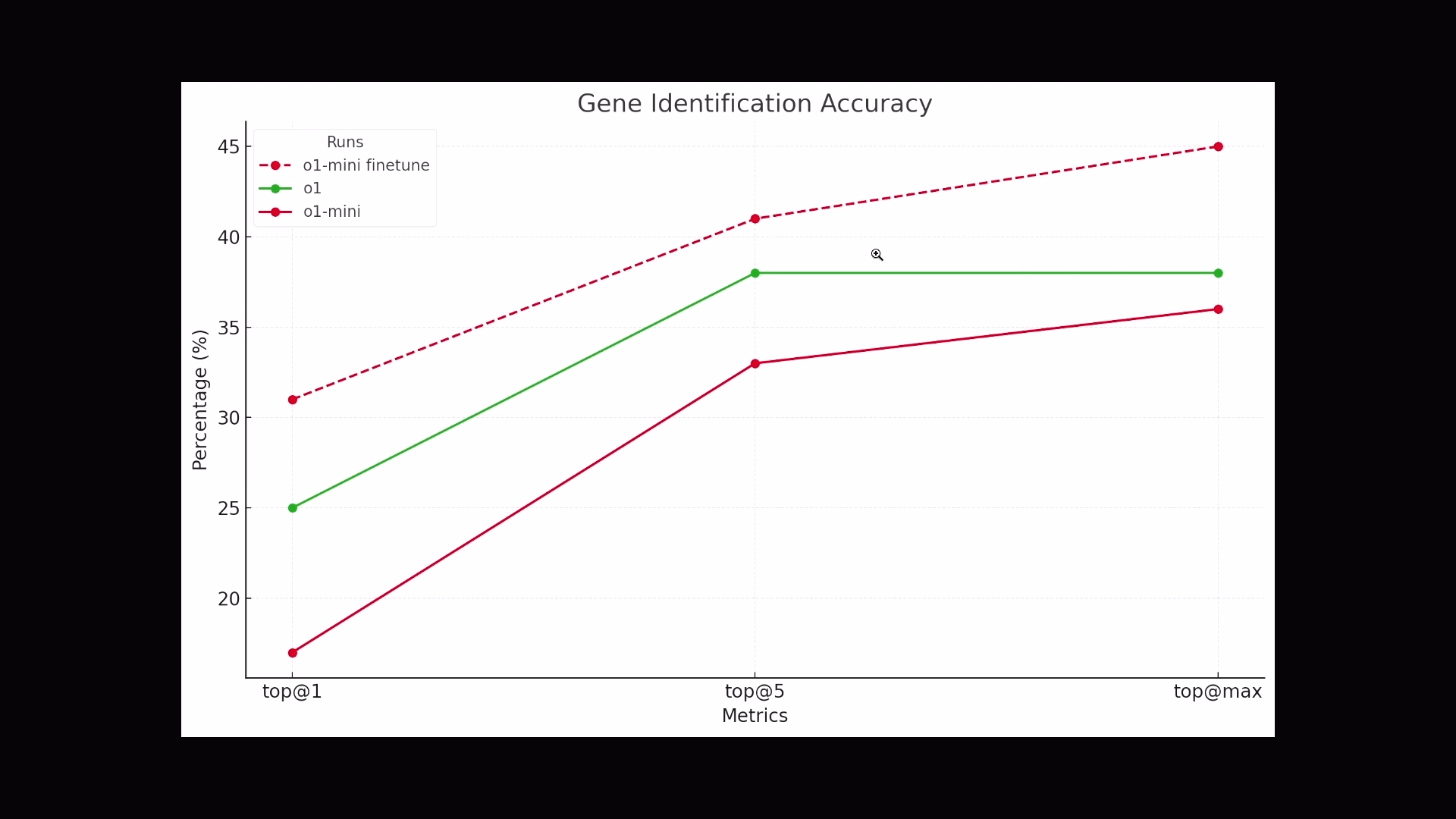
Early access program
OpenAI is now accepting organizations into its Reinforcement Fine-Tuning Research Program. The program is designed for organizations working on complex tasks that could benefit from AI assistance.
Participants will receive access to the RFT API and can help improve it through feedback before its public release. OpenAI plans to make RFT more widely available in early 2025.