A recent analysis by the ARC Prize Foundation finds that OpenAI’s o3 model delivers significantly weaker results on standardized reasoning benchmarks than its previously tested o3 preview version.
The ARC Prize Foundation, a nonprofit group focused on AI evaluation, uses open benchmarks like ARC-AGI to highlight the gap between human reasoning and current artificial intelligence systems. Each evaluation aims to clarify the current state of the field.
The ARC-AGI benchmark is structured to test symbolic reasoning, multistep composition, and context-dependent rule application—skills that humans often demonstrate without special training, but which AI models only perform to a limited extent.
The analysis assessed performance at "low," "medium," and "high" reasoning levels, which vary the depth of model reasoning. "Low" prioritizes speed and minimal token usage, while "high" is intended to encourage more comprehensive problem-solving. For this study, two models—o3 and o4-mini—were tested at all three reasoning levels on 740 tasks from ARC-AGI-1 and ARC-AGI-2, producing 4,400 data points.
Cost efficiency and performance: o3 outpaces o1
According to the ARC Prize Foundation, o3 achieved 41 percent accuracy (low compute) and 53 percent (medium compute) on ARC-AGI-1. The smaller o4-mini model reached 21 percent (low compute) and 42 percent (medium compute). On the more challenging ARC-AGI-2 benchmark, both current models struggled considerably, scoring below three percent accuracy.
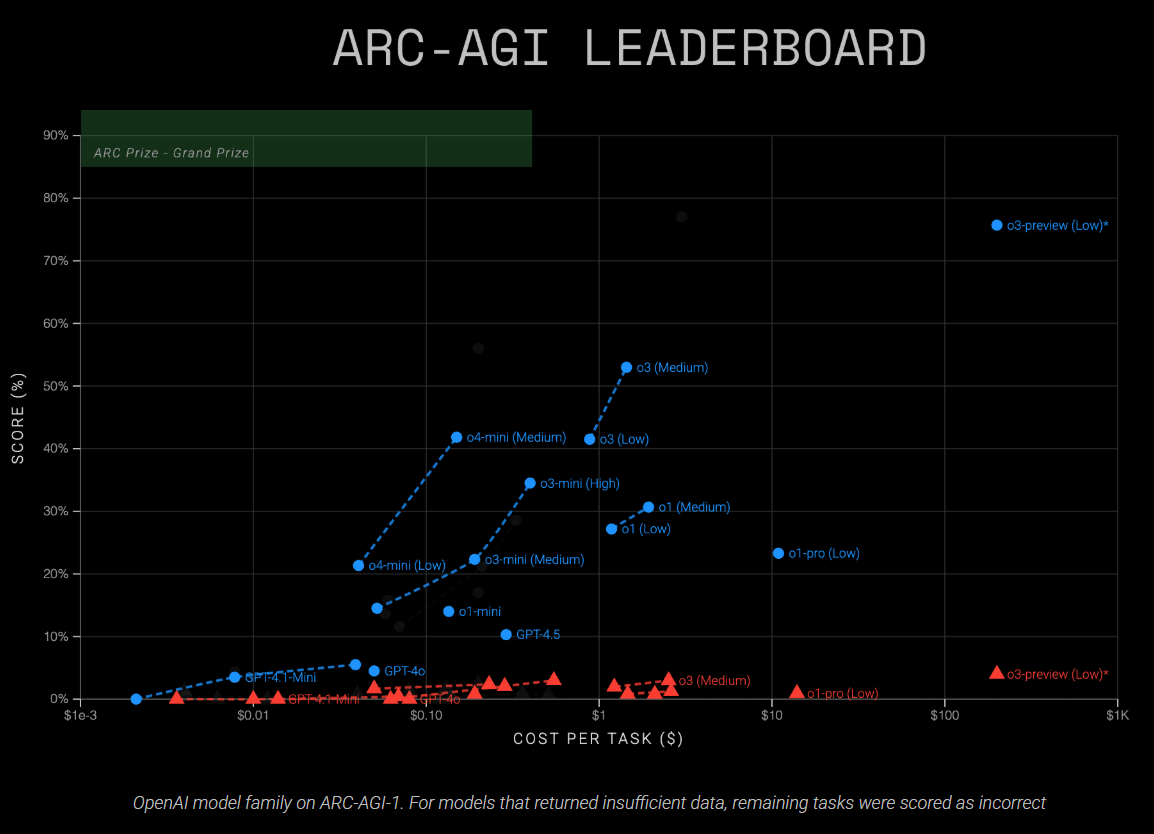
At higher reasoning levels ("high" compute), both models failed to complete many tasks. The analysis also observed that models tended to answer tasks they could solve more easily, while leaving more difficult tasks unanswered. Assessing only the successful responses would distort the actual performance, so these partial results were excluded from official leaderboards.
Model | Reasoning setting | Semi Private Eval V1 | Semi Private Eval V2 | Cost per task (V2) |
---|---|---|---|---|
o3 | Low | 41% | 1,9% | 1.22 US dollars |
o3 | Medium | 53% | 2,9% | 2.52 US dollars |
o3 | High | - | - | - |
o4-mini | Low | 21% | 1,6% | 0.05 US dollar |
o4-mini | Medium | 42% | 2,3% | 0.23 US dollar |
o4-mini | High | - | - | - |
The data shows that higher reasoning effort does not guarantee better results, but often results only in higher costs. In particular, o3-high consumes significantly more tokens without achieving a corresponding gain in accuracy for simpler tasks. This raises questions about the scalability of the current approach to chain-of-thought reasoning.
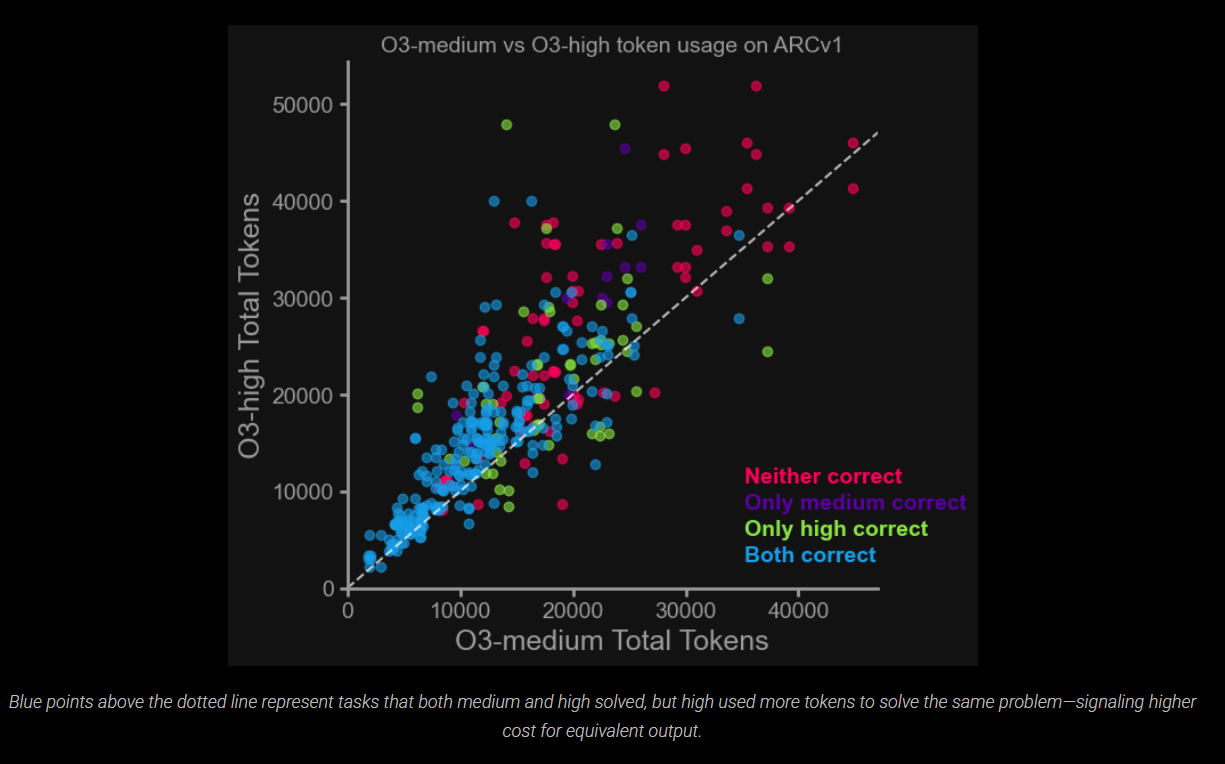
For cost-sensitive applications, the ARC Prize Foundation advises using o3-medium as the default setting. The "high-reasoning" mode is only recommended when maximum accuracy is needed and cost is less important. "There is no compelling reason to use low if you care about accuracy," says Mike Knoop, co-founder of the ARC Prize Foundation.
The Foundation also notes that as model performance advances, efficiency—how quickly, inexpensively, and with minimal token usage a model can solve problems—becomes the primary differentiator. In this regard, o4-mini is notable: it achieves 21 percent accuracy on ARC-AGI-1 at a cost of about five cents per task, while older models like o1-pro require roughly eleven dollars per task for comparable results.
OpenAI's o3 is less AGI than o3-preview
The current o3 release diverges substantially from the o3-preview version tested in December 2024. At that time, o3-preview scored 76 percent (low compute) and 88 percent (high compute) on ARC-AGI-1 in text mode, while the released o3 model now delivers only 41 percent (low) and 53 percent (medium).
OpenAI confirmed to ARC that the production o3 model differs from the preview version in several key ways. The company explained that the released model has a different architecture, is an overall smaller model, operates multimodally (handling both text and image inputs), and uses fewer computational resources than the preview version.
Regarding training data, OpenAI states that the training of o3-preview covered 75 percent of the ARC-AGI-1 dataset. For the released o3 model, OpenAI says it was not trained directly on ARC-AGI data, not even on the training dataset. However, it is possible the model was indirectly exposed to the benchmark through its public availability.
The released o3 model has also been refined for chat and product use cases, which—according to ARC Prize—results in both advantages and disadvantages on the ARC-AGI benchmark. These differences underscore that benchmark results, especially for unreleased AI models, should be viewed with caution.
Ongoing progress and persistent limitations
The o3-medium model currently delivers the highest performance among publicly tested ARC Prize Foundation models on ARC-AGI-1, doubling the results of earlier chain-of-thought approaches.
Despite this improvement, the newly introduced ARC-AGI-2 benchmark remains largely unsolved by both new models. While humans solve an average of 60 percent of ARC-AGI-2 tasks even without special training, OpenAI's strongest reasoning model currently achieves only about three percent.
"ARC v2 has a long way to go still, even with the great reasoning efficiency of o3. New ideas are still needed," Knoop writes.
This highlights a persistent gap in problem-solving ability between humans and machines, despite recent advances and what Microsoft CEO Satya Nadella has described as "nonsensical benchmark hacking."
A recent analysis also suggests that so-called reasoning models such as o3 probably do not have any new capabilities beyond those of their foundational language models. Instead, these models are optimized to arrive at correct solutions more quickly for certain tasks—particularly those for which they have been trained through targeted reinforcement learning.