A study by the University of California, Berkeley, enables robots to navigate based on the principle of word prediction from language models. This approach could pave the way for a new generation of robots that can navigate complex environments with minimal training.
In their paper, "Humanoid Locomotion as Next Token Prediction," the researchers treat the complex task of robot locomotion as a sequence prediction problem, similar to predicting the next word in language generation.
They use the same Transformer technology that made the breakthrough in large language models and adapt it to predicting robot steps.
The robot's steps are treated as "tokens", comparable to words in a sentence. By predicting these tokens, the Transformer learns to predict the next movement based on the previous movement sequence. In other words, the robot predicts each next step based on the steps it has already taken.
The model was trained using a mix of data sources, including human motion data and YouTube videos. According to the researchers, the robot was able to navigate the streets of San Francisco without seeing any specific examples of the environment beforehand (so-called zero-shot). This was achieved by utilizing just 27 hours of walking data for model training.
The model can also execute commands that it had not seen in training, such as walking backwards. This adaptability could enable robots to move flexibly in complex real-world environments with a fraction of the training effort otherwise required.
Predictions help optimize diverse multimodal training data
The researchers' method shines in its ability to handle assorted data sources, ranging from videos and sensor readings to computer simulations, converting this information into a uniform format for the Transformer's use.
They also devised a strategy for capitalizing on incomplete data by introducing learnable mask tokens that can predict the available information, thus overcoming gaps in the data. For example, for YouTube videos, the researchers used the joint positions of the human body to transfer the motion to the humanoid robot.
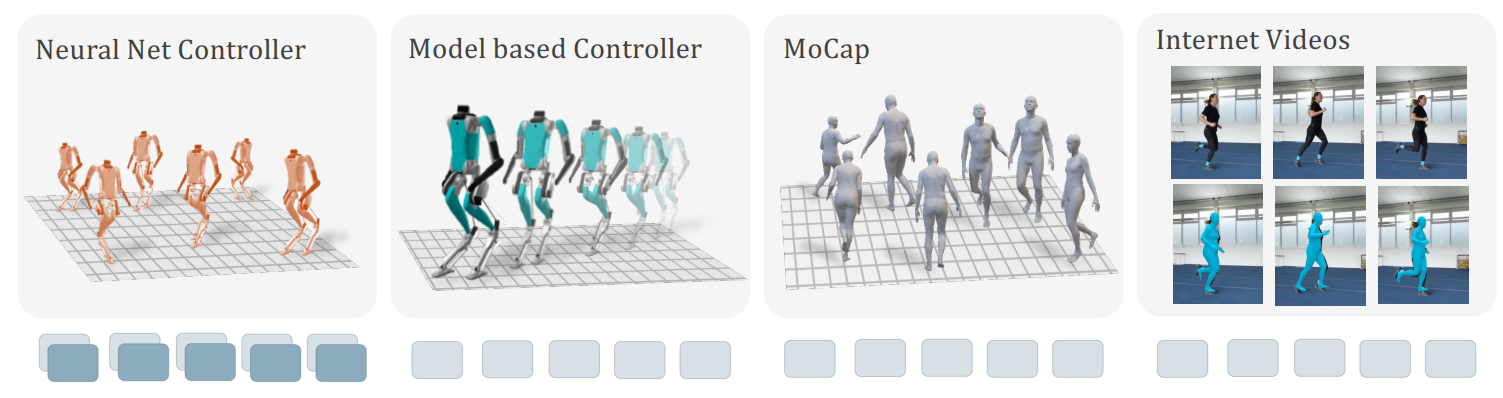
The team's core insight is that even with incomplete trajectories, where certain sensory or motor information is missing, the model can still learn effectively by predicting the available information and filling in the gaps with learnable mask tokens.
They believe that the model's success in making accurate predictions under these conditions suggests it is developing a more sophisticated understanding of the physical world, an intuition that holds exciting potential for the future of autonomous robotics. It's as if the robot is learning to think on its feet, which could change the way robots move in the future.