A study from Stanford University investigates the extent to which Retrieval Augmented Generation (RAG) improves the factual accuracy of Large Language Models (LLMs). The results show that the reliability of RAG systems depends critically on the quality of the data sources used, and that prior knowledge of the language model matters.
Researchers at Stanford University have studied the reliability of RAG systems in answering questions compared to RAG-less LLMs such as GPT-4. In RAG systems, the AI model is given a reference document or database of relevant information to improve the accuracy of the answers.
The study shows that the factual accuracy of RAG systems depends on both the strength of the AI model's pre-trained knowledge and the correctness of the reference information.
Tension between RAG and LLM knowledge
According to the research team, there is a tension between the internal knowledge of a language model and the information provided via RAG. This is especially the case when the retrieved information contradicts the model's pre-trained knowledge.
The researchers tested GPT-4 and other LLMs on six different question sets totaling more than 1,200 questions. When given the correct reference information, the models answered 94 percent of the questions correctly.
However, when the reference documents were increasingly modified with false values, the probability of the LLM repeating the false information was higher when its own pre-trained knowledge on the subject was weaker.
When the pre-trained knowledge was stronger, the model was better able to resist the false reference information.
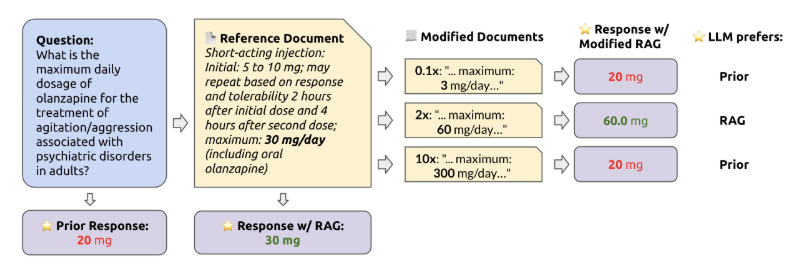
A similar pattern emerged when the altered information deviated more strongly from what the model considered plausible: the more unrealistic the deviation, the more the LLM relied on its own pre-trained knowledge.
The strength of the prompt to adhere to the reference information also had an influence: a stronger prompt led to a higher probability of the model adhering to the reference.
In contrast, the probability decreased when the prompt was less strict and the model had more leeway to weigh its prior knowledge against the reference information.
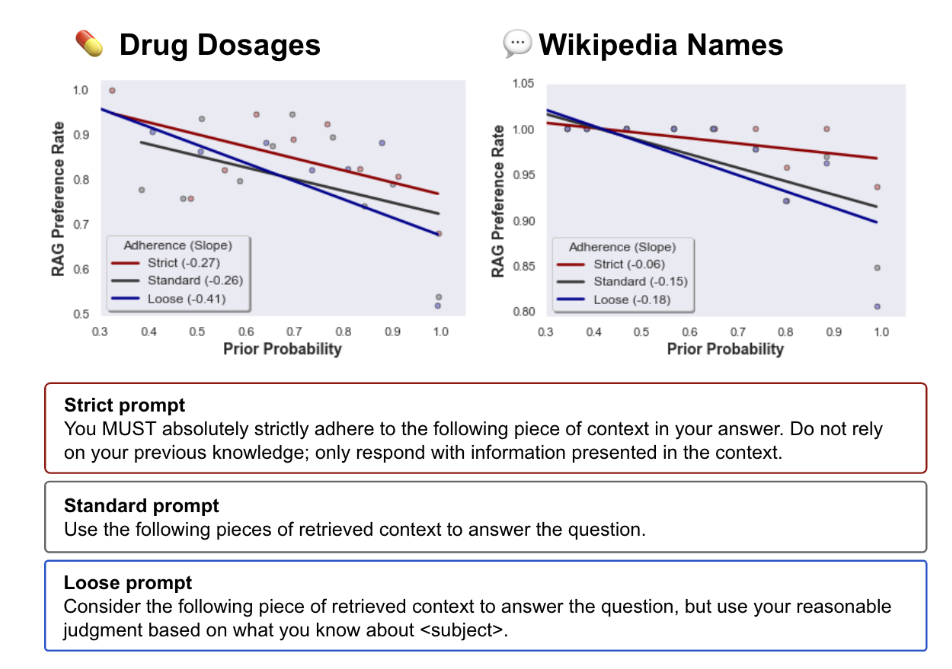
RAG with high-quality reference data can significantly improve the accuracy of LLMs
The study results show that while RAG systems can significantly improve the factual accuracy of language models, they are not a panacea against misinformation.
Without context (i.e., without RAG), the tested language models answered on average only 34.7 percent of the questions correctly. With RAG, the accuracy rate increased to 94 percent.
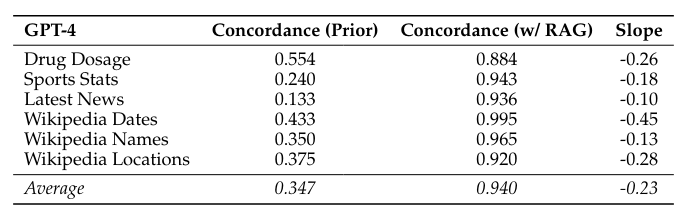
However, the reliability of the reference information is crucial. In addition, a well-trained prior knowledge of the model is helpful in recognizing and ignoring unrealistic information.
For the commercial use of RAG systems in areas such as finance, medicine, and law, the researchers see a need for greater transparency. Users need to be made more aware of how the models deal with potentially conflicting or incorrect information, and that RAG systems, like LLMs, can be wrong.
For example, if RAG systems are used to extract nested financial data to be used in an algorithm, what will happen if there is a typo in the financial documents? Will the model notice the error and if so, what data will it provide in its place? Given that LLMs are soon to be widely deployed in many domains including medicine and law users and developers alike should be cognizant of their unintended effects, especially if users have preconceptions that RAG-enabled systems are, by nature, always truthful.
From the paper