A new study from Germany's TU Darmstadt shows that even the most sophisticated AI image models fail at simple visual reasoning tasks.
The researchers tested various vision language models (VLMs) using Bongard problems—simple visual puzzles that most people can solve intuitively. These puzzles, created by Russian scientist Michail Bongard, present twelve simple images that are divided into two groups. The challenge is to identify the rule that separates these groups, a task that tests abstract reasoning skills.
Even GPT-4o falls short
The study's findings are striking: The models struggled with basic tasks that most people find simple.
For example, they had trouble distinguishing between vertical and horizontal lines, or determining the direction of rotation of a spiral. These basic visual concepts proved challenging for even the most sophisticated AI models.
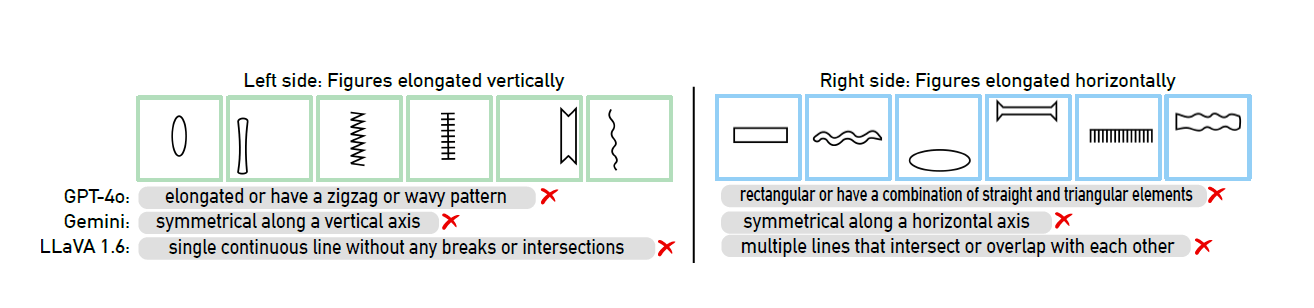
GPT-4o, currently considered the most advanced multimodal model, could only solve 21 out of 100 visual puzzles. Other well-known AI models, including Claude, Gemini, and LLaVA, performed even worse.
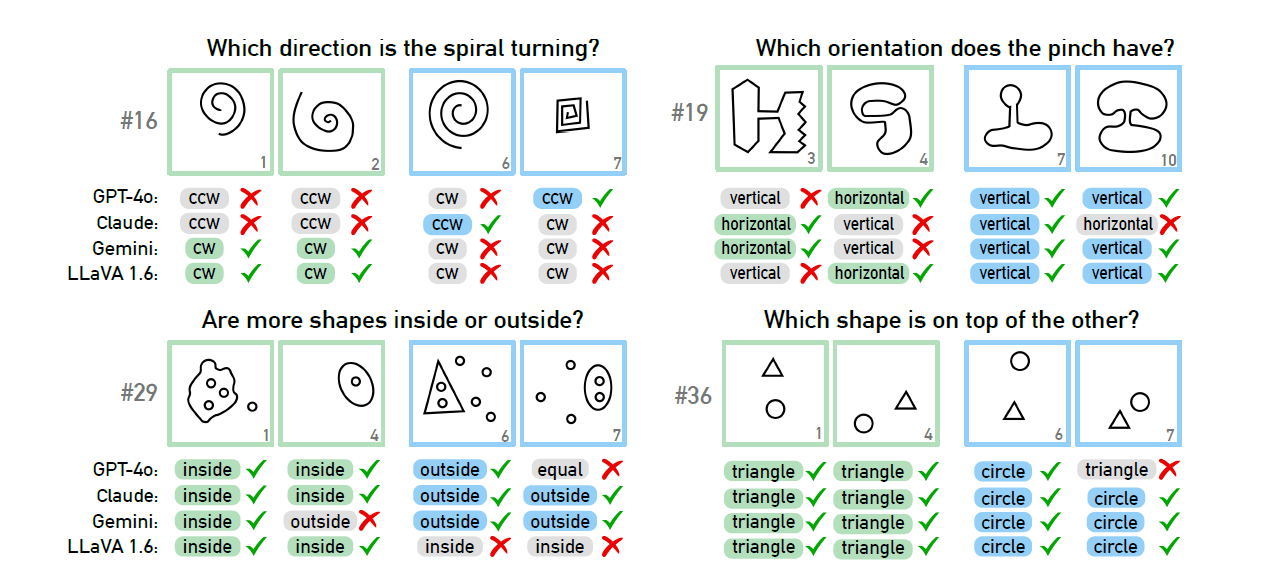
When researchers provided multiple-choice options, the results improved only marginally. The AI models only showed significant improvement when the number of possible answers was severely restricted—under these conditions, GPT-4 and Claude managed to solve 68 and 69 out of 100 puzzles respectively.

The researchers studied the reasons for the models' failures in detail in four selected problems. They found that AI systems sometimes fail at the basic level of visual perception, even before reaching the actual "thinking" and "reasoning" stages. But they couldn't find a single clear cause.
Rethinking AI evaluation benchmarks
The study raises questions about the evaluation of AI systems and suggests that existing benchmarks may not accurately measure the true reasoning abilities of models. The research team recommends rethinking these benchmarks to better assess the visual reasoning abilities of AI.
"Our findings raise several critical questions: Why do VLMs encounter difficulties with seemingly simple Bongard Problems, despite performing impressively across various established VLM benchmarks? How meaningful are these benchmarks in assessing true reasoning capabilities?" the researchers write.
The study was conducted by Technische Universität Darmstadt in collaboration with Eindhoven University of Technology and the German Research Center for Artificial Intelligence (DFKI), with funding from the German Federal Ministry of Education and Research and the European Union.