Saliency maps are supposed to shed light on the black box of AI in medicine. In a benchmark, researchers now show that the widely used method is more unreliable than assumed.
Explainability plays a crucial role in many applications of artificial intelligence, especially where the predictions of deep neural networks have a direct impact on people's lives. There are numerous methods to make the black box AI more understandable. In medical diagnostics, where much of the data is image data, there is an increased use of so-called saliency maps (in the form of heat maps), which visualize zones or points on X-ray images, for example, that have influenced an AI system's interpretation.
Saliency maps not ready for prime time according to study
Saliency methods like Grad-CAM are combined with an AI model that is used for the diagnosis itself. The visualizations should then enable treating physicians, but also patients, to understand or verify the predictions. The methods are also intended to increase the acceptance of AI systems in medicine.
In a new study, researchers now show that all widely used saliency methods lag behind the performance of human experts, regardless of the diagnostic model used. The team, therefore, concludes that these methods are not yet ready for widespread use in clinical practice.
"CheXlocalize" benchmark shows the limitations of the widely used method
In the analysis led by Pranav Rajpurkar of Harvard Medical School, Matthew Lungren of Stanford and Adriel Saporta of New York University, the authors reviewed seven widely used salience methods for their reliability and accuracy in identifying ten diseases diagnosed on X-ray images. They also compared the performance of the methods with that of human experts.
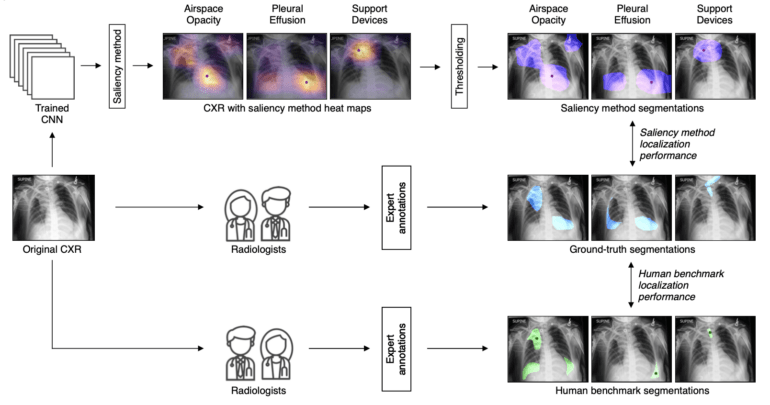
In their analysis, all saliency methods consistently performed worse than human radiologists in image assessment and pathological lesion detection. Where such methods are already in use, the authors urge caution.
"Our analysis shows that saliency maps are not yet reliable enough to validate individual clinical decisions made by an AI model," said Rajpurkar, who is an assistant professor of biomedical informatics at HMS. "We identified important limitations that raise serious safety concerns for use in current practice."
The team suspects algorithmic artifacts in the saliency methods, whose relatively small heat maps (14 x 14 pixels) are interpolated to the original image dimensions (typically 2000 x 2000 pixels), as a possible cause of the poor results.
The team's code, data, and analysis are available on GitHub.