Researchers from Shanghai Jiao Tong University, Fudan University, the Shanghai AI Laboratory, and the Generative AI Research Lab have developed a method that allows stronger AI models to improve their reasoning abilities through guidance from weaker models.
Developing AI systems that surpass human cognitive abilities, known as superintelligence, remains a key goal in artificial intelligence. However, as AI models improve, standard feedback methods and AI training have limitations because humans still play a critical role in developing the models.
To solve this, the research team introduced the "weak-to-strong" learning approach. It helps strong models reach their full potential without relying on human input or even stronger models.
The two-step training method allows the strong model to refine its training data on its own.
First, the researchers combine data from the weak model (Llama2-7b, Gemma-2b, and Mistral-7b) with data from the strong model (Llama2-70b, Llama3-70b) through "in-context learning" (generating with examples). This lets them carefully select data sets for later supervised fine-tuning. Second, the strong model is improved further through preference optimization by learning from the weak model's mistakes.
AI optimizes training data better than humans
Initial experiments show the new approach works much better than simple fine-tuning on weak data. In the GSM8K benchmark for math tasks, the strong model's performance, only supervised by the weak Gemma 2b model, increased by up to 26.99 points (Stage I).
Preference optimization (Stage II) achieved a further 8.49-point improvement. According to the researchers, this means that the method outperforms even the fine-tuning on gold standard solutions.
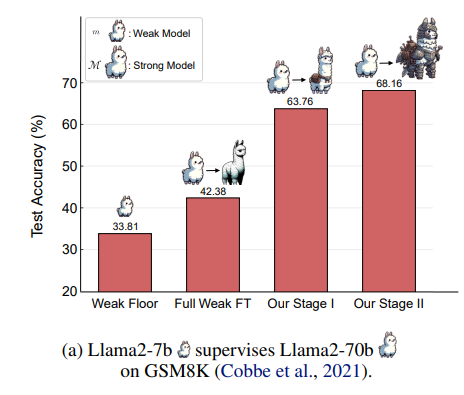
The researchers point out that their method allows the strong model to continually improve its mathematical skills by refining its training data on its own. It shows how AI can use self-refinement of training data to take on tasks that don't yet have predefined human or AI solutions, and where standard fine-tuning methods fail, to improve where human oversight reaches its limits.
Former OpenAI researcher Andreji Karpathy also sees using AI models to optimize training data as a potential next driver of AI progress. It could be AI that develops the "perfect data set" for AI.