Researchers have developed a new AI model called MedSAM-2 that significantly improves the segmentation of 2D and 3D medical images. With just a single manual annotation, it can process entire series of images.
Scientists from the University of Oxford have introduced a powerful AI model for medical image segmentation. MedSAM-2 builds on the recently released Segment Anything Model 2 (SAM 2) from Meta and treats medical images similarly to video sequences. According to the study, MedSAM-2 outperforms previous state-of-the-art models in segmenting various organs and tissues in 2D and 3D images.
To achieve these capabilities, MedSAM-2 treats medical image series like video sequences. The model also uses a "Confidence Memory Bank" that stores the most reliable predictions. When analyzing new images, it refers back to this information and weights it based on similarity to the current image.
A key feature of MedSAM-2 is its ability to perform "one-prompt segmentation." This means the model can recognize and segment similar structures in additional images with just a single annotation in an example image. This technique also works for images without temporal relationships and significantly reduces the workload for medical personnel.
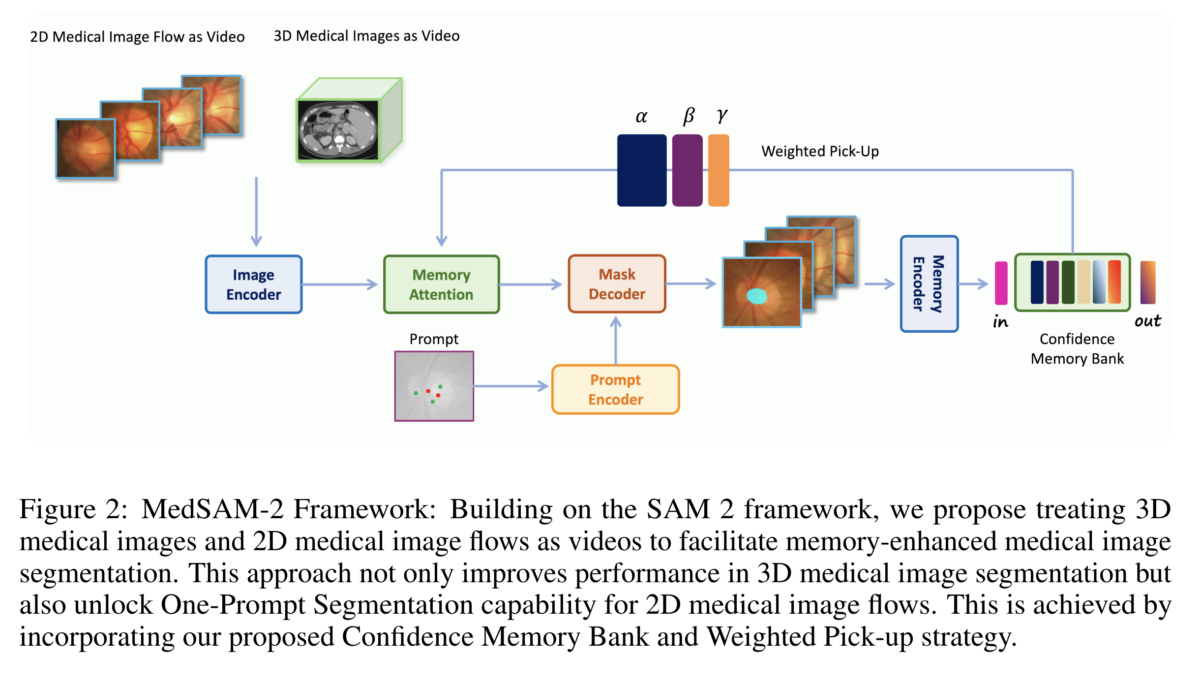
MedSAM-2 sets new standards in performance and user-friendliness
The researchers tested MedSAM-2 on 15 different medical datasets, including scans of abdominal organs, optic nerves, brain tumors, and skin lesions. In almost all cases, the new model achieved better results than specialized predecessor models.
For segmenting abdominal organs in 3D images, MedSAM-2 achieved an average Dice score of 88.6 percent. This value surpasses the previous top model, MedSegDiff, by 0.7 percentage points. The Dice score is a measure of the agreement between the AI prediction and the manual segmentation by experts.
MedSAM-2 also performed better than previous models on 2D images of various body regions. For optic nerve segmentation, it improved the Dice score by 2 percentage points, for brain tumors by 1.6, and for thyroid nodules by 2.8 percentage points.
The developers of MedSAM-2 see the model as an important step towards improving medical image analysis and have released the model and code on GitHub. This is intended to encourage further development and use in clinical practice.