Independent testing has found that OpenAI's o1 model solves just 30 percent of programming tasks in benchmark tests - not the 48.9 percent the company claimed. The findings add to a growing debate about how to measure AI capabilities.
In his new study using OpenAI's coding benchmark "SWE-Bench Verified," AI researcher Alejandro Cuadron found what he calls a "surprising" gap. While OpenAI reported their model could handle nearly half of the real-world programming tasks from Github, Cuadron's testing shows it managing less than a third.
Anthropic's Sonnet 3.5 came out ahead, solving 53 percent of the tasks - though this might be because the model helped develop the test procedure itself. Notably, the less expensive Deepseek v3 model performed about as well as OpenAI's o1 in Cuadron's testing.
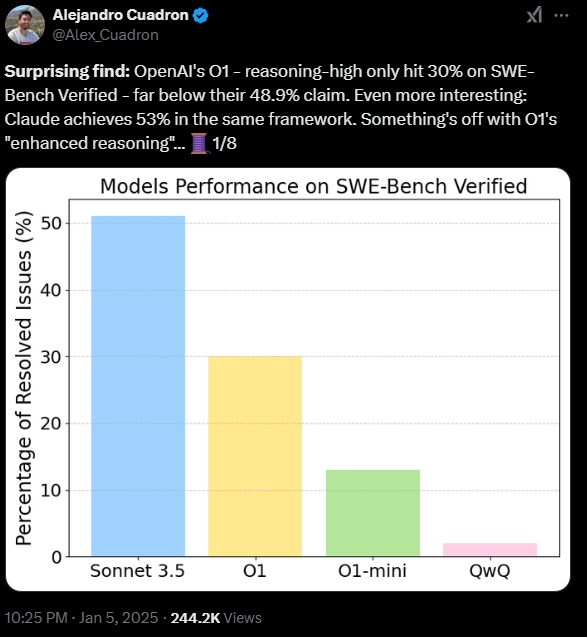
Why the big difference?
The gap between OpenAI's claims and Cuadron's findings comes down to testing methods. OpenAI used "Agentless," a framework that gives AI very specific instructions for solving programming tasks. Cuadron, on the other hand, used "OpenHands," which gives the AI more freedom in how it approaches problems.
Cuadron says that OpenHands was considered the gold standard when OpenAI ran their tests, but they chose not to use it. He suspects OpenAI's more rigid testing method might favor models that simply memorize solutions rather than truly solving new problems independently.
This isn't just academic nitpicking. OpenAI has touted o1's supposed strength in reasoning and ability to tackle novel problems. "But why does O1 [sic] struggle with true open-ended planning despite its reasoning capabilities?" Cuadron writes.
Other research has cast doubt on these claims before. A recent travel planning benchmark showed o1-preview struggling with planning tasks, and an Apple study found that slightly varied math problems led to much worse performance - suggesting the model isn't good at generalizing knowledge, which undermines claims about its logical capabilities.
The bigger picture
This situation highlights a persistent problem in AI evaluation: benchmark results depend heavily on testing methods. When companies can optimize their models for specific test procedures, it becomes nearly impossible for outsiders to gauge an AI's true capabilities. This matters because these benchmark results drive PR campaigns and marketing efforts, which in turn influence investor funding.
Logan Kilpatrick, who recently moved from OpenAI to become Head of Product at Google AI Studio, emphasizes the need for better verification and openness in testing procedures. While he doesn't suspect OpenAI of any deliberate deception, he sees solving these evaluation problems as crucial for developing more advanced AI systems.
"The world needs [more, better, harder, etc] evals for AI," Kilpatrick argues. "This is one of the most important problems of our lifetime, and critical for continual progress."