A team of researchers from Hong Kong and the UK has introduced a new method for converting images into digital representations—also known as tokens—using a hierarchical structure designed to capture essential visual information more compactly and accurately.
Unlike conventional approaches that distribute image information evenly across all tokens, this method arranges tokens hierarchically. The earliest tokens encode high-level visual features, such as broad shapes and structural elements, while subsequent tokens add increasingly fine-grained details until the full image is represented.
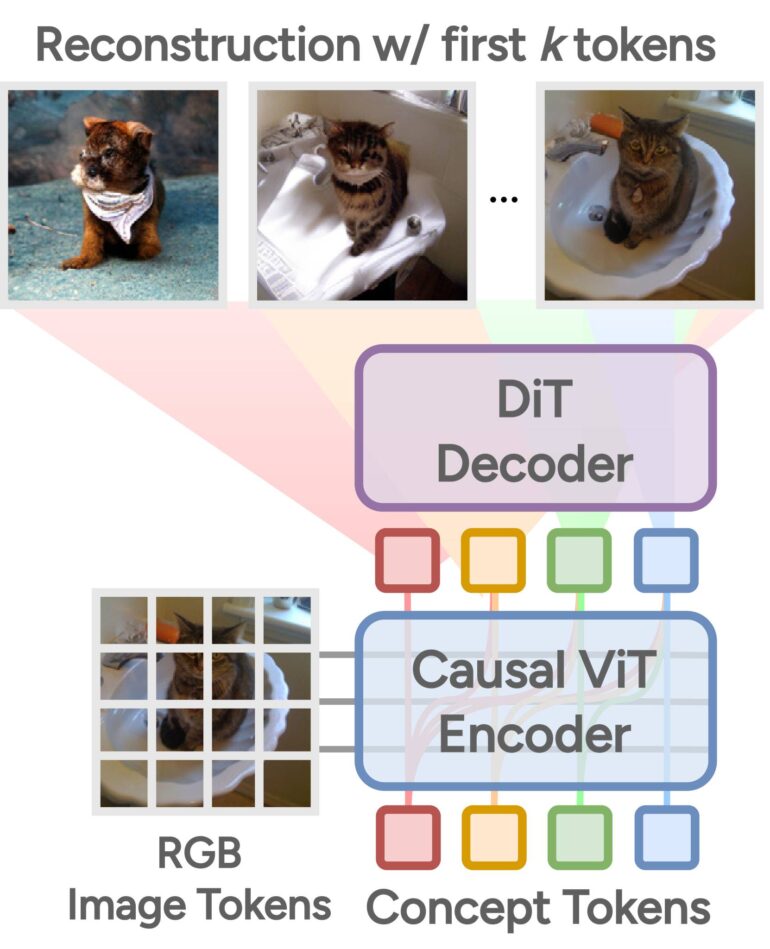
This strategy draws on the core idea behind principal component analysis, a statistical technique in which data is broken down into components that explain variance in descending order. The researchers applied a similar principle to image tokenization, resulting in a representation that is both compact and interpretable.
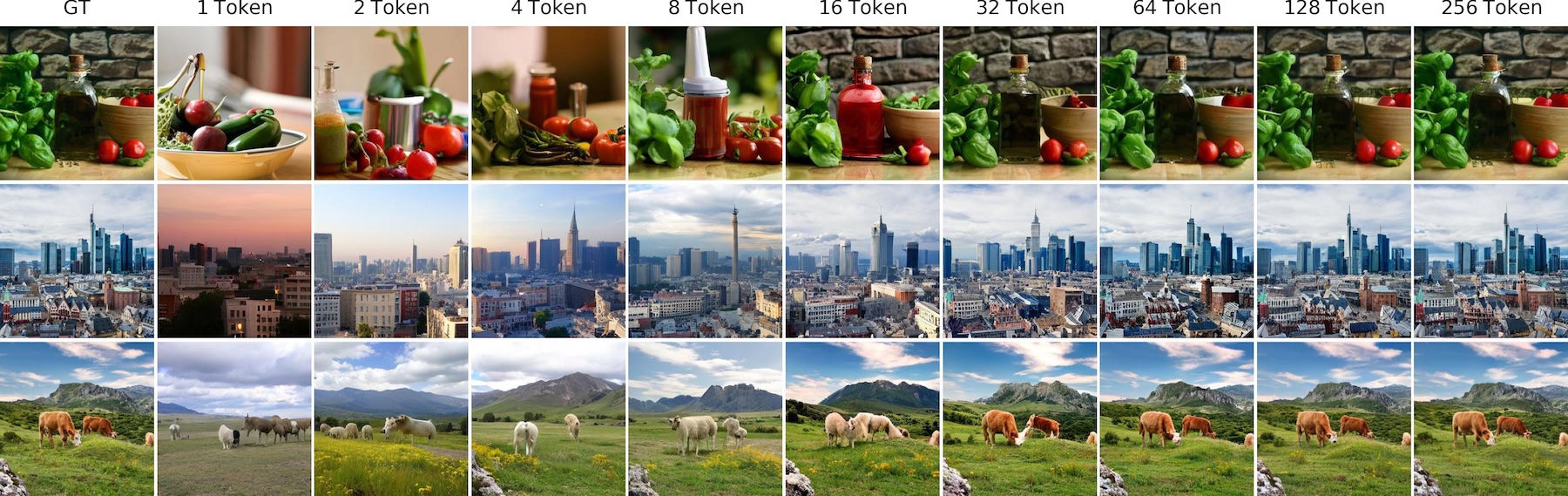
One key innovation is the separation of semantic content from low-level image details. In previous methods, these types of information were often entangled, making it difficult to interpret the learned representations. The new method addresses this by using a diffusion-based decoder that reconstructs the image gradually, starting from coarse shapes and progressing to fine textures. This allows the tokens to focus on semantically meaningful information while treating detailed textures separately.
Approach improves reconstruction quality
According to the researchers, this hierarchical method improves image reconstruction quality—the similarity between the original image and its tokenized version—by nearly 10 percent compared to previous state-of-the-art techniques.
It also achieves comparable results using significantly fewer tokens. In downstream tasks like image classification, the method outperformed earlier approaches that rely on conventional tokenization.
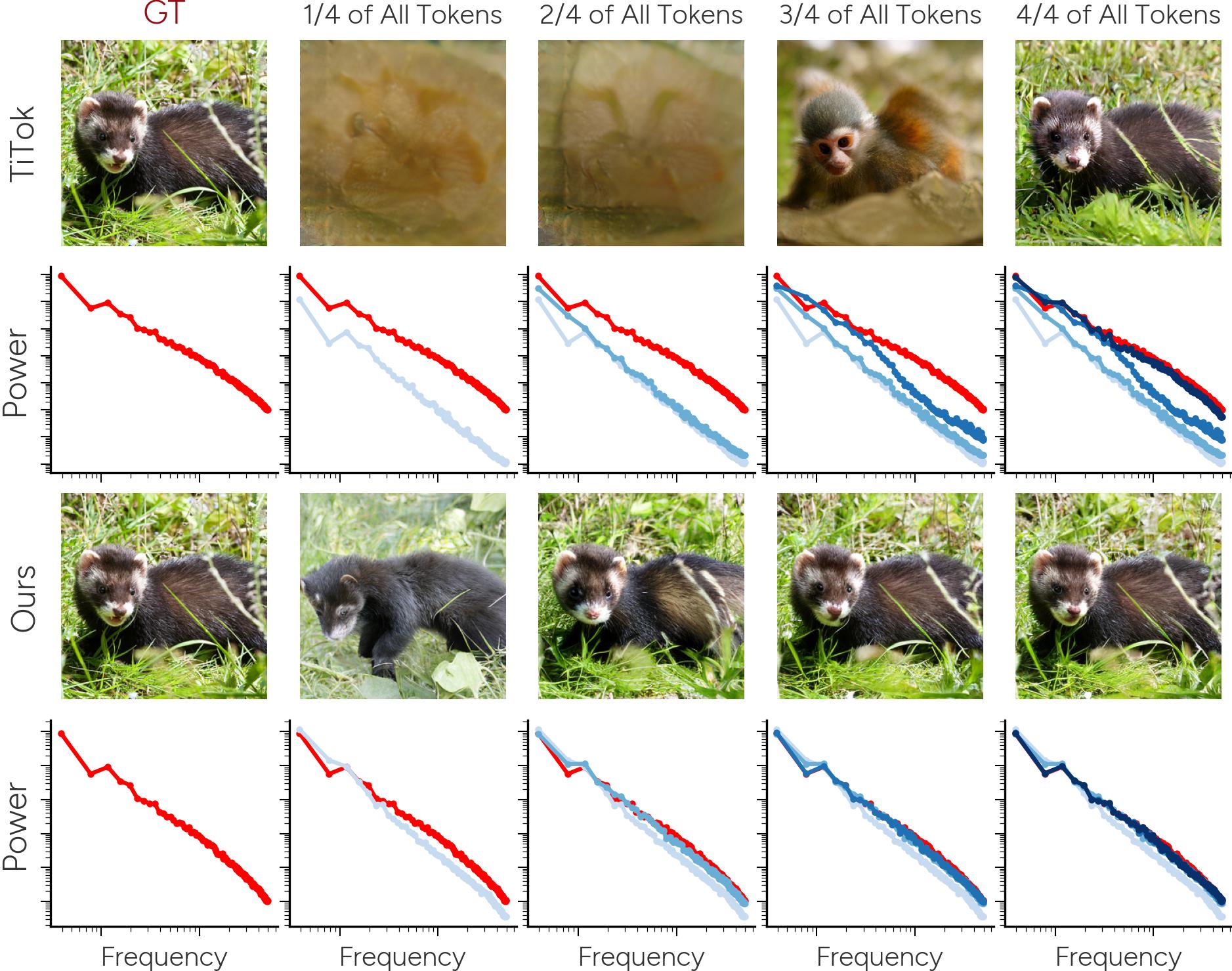
The researchers note that the hierarchical structure mirrors how the human brain processes visual input—from coarse outlines to increasingly detailed features. According to the study, this alignment with perceptual mechanisms may open new directions for developing AI systems for image analysis and generation that are more in tune with human visual cognition.
Improving interpretability and efficiency in AI systems
The new method could help make AI systems easier to understand. By separating semantic content from visual detail, the learned representations become more interpretable, which may make it simpler to explain how the system arrives at its decisions. At the same time, the compact structure allows for faster processing and reduced storage requirements.
The researchers call the approach an important step towards image processing that is more closely aligned with human perception, but they also see room for improvement. Future work will focus on refining the technique and applying it to a wider range of tasks.
Tokenization remains a core component in both image and language models. New strategies for digitally encoding text segments are also emerging, and some researchers believe these could lead to more advanced language models in the future.