The progress of open-source language models is undisputed. But can they really compete with the much pricier, heavily trained language models from OpenAI, Google, and others?
Sounds too good to be true: With little training effort and almost no money, open-source language models trained using the Alpaca Formula have set new benchmarks recently, reaching ChatGPT-like levels.
The Alpaca Formula means that developers use training data generated with ChatGPT to fine-tune the half-leaked and half-published language model LLaMA by Meta. Using this data, the LLaMA model learns to produce output similar to ChatGPT in a very short time and with little computational effort.
One Google engineer even sounded the alarm internally about the growing capabilities of open-source models. He suggested that the open-source scene could overtake commercial modelers like Google.
But researchers at the University of Berkeley came to a different conclusion in a recent study: They applied the Alpaca formula to some base models from LLaMA and GPT-2, then had those results evaluated by humans and automated by GPT-4.
Initially, they came to the same conclusion as developers before them: The performance of the models refined with instructions, which the researchers call "imitation models," far exceeded that of the base model and was on par with ChatGPT.
We were initially surprised by how much imitation models improve over their base models: they are far better at following instructions, and their outputs appear similar to ChatGPT’s. This was further supported by both human and GPT-4 evaluations, where the outputs of our best imitation model were rated as competitive with ChatGPT.
From the paper
The "false promise" of model imitation or: there are no shortcuts
However, "more targeted automatic evaluations" showed that the imitation models actually performed well only in the tasks for which they had seen imitation data. In all other areas, a clear performance gap remained with GPT-4 and co. because these base models acquire much of their capabilities during extensive pre-training, not during fine-tuning.
Human evaluators may overlook this discrepancy, as imitation models continue to copy ChatGPT's confident style, but not its factuality and plausibility. Simply put, imitation models learn more style than content, and humans unfamiliar with the content do not notice.
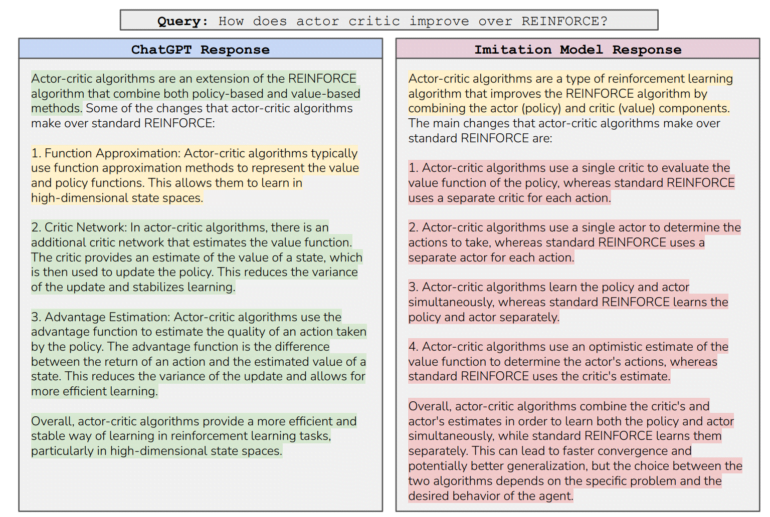
These so-called crowd workers, who often evaluate AI content without the expertise and in a short amount of time, are easily fooled, the researchers suggest. "The future of human evaluation remains unclear," they write. GPT-4 may be able to emulate human reviewers in some areas, but it showed human-like cognitive biases. More research is needed, they say.
The researchers' conclusion: An "unwieldy amount of imitation data" of imitation data - or better baseline models - would be needed to compensate for the imitation models' weaknesses.
AI model moat is real
According to the study, the best-positioned companies will be those that can teach their models capabilities with massive amounts of data, computing power, and algorithmic edge.
In contrast, companies that refine "off-the-shelf" language models with their data sets would have a hard time building a moat. Moreover, they would have no input into the design decisions of the teacher model and would be limited upward by its capabilities.
A positive aspect of imitation learning, they said, is that when the style is adopted, the behavior associated with safe and toxic responses is also adopted by the instructor model. As a result, imitation learning would be particularly effective if you had a powerful base model and needed cheap data to fine-tune it.
OpenAI researcher John Schulman also recently criticized using ChatGPT data to fine-tune open-source base language models, saying that they could produce more incorrect content if the fine-tuning data sets contain knowledge that is not present in the original model.
The open-source OpenAssistant project stands out here, where the datasets are collected by human volunteers rather than generated by AI, which Meta's AI chief Yann LeCun believes is the way to go, saying that "human feedback *must be crowd-sourced, Wikipeda-style" so that open-source base models can become something like the "common repository of human knowledge."