Researchers from Metas AI Research (FAIR), HuggingFace, AutoGPT, and GenAI present the GAIA (General AI Assistants) AI benchmark, which measures AI performance on tasks that are easy for humans to solve.
The benchmark is based on the hypothesis that a potential General Artificial Intelligence (AGI) must outperform humans even on tasks that are easy for the average person to solve.
In contrast, the trend in benchmarks is to have AI solve tasks that are difficult for humans or require a high level of technical skill and knowledge, the researchers write.
GAIA aims to accelerate the development of AI systems with human-like capabilities across a broader range of tasks. The research team expects that solving the GAIA benchmark will mark a milestone in the development of AI.
466 everyday tasks
GAIA consists of 466 questions that require basic skills such as reasoning, dealing with different modalities, navigating the Internet, and using tools (e.g., Internet search). The questions are designed to be challenging for AI systems, but conceptually simple for humans.
Questions are divided into three levels of difficulty based on the number of steps required to solve the question and the number of different tools required.
Level 1 questions usually require no tools or at most one tool, but no more than five steps. Level 2 questions usually have more steps, about five to ten, and require a combination of tools. Level 3 questions require a system that can perform sequences of actions of any length, use any number of tools, and have general access to the world.
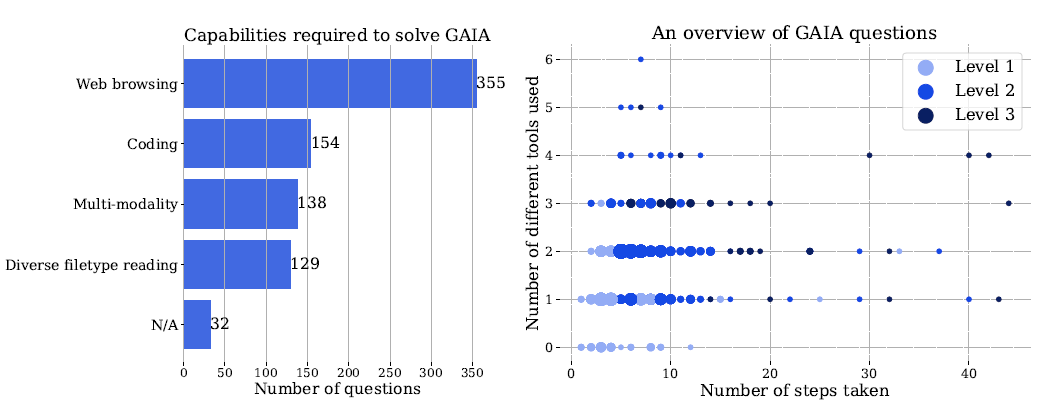
GAIA attempts to avoid the pitfalls of current AI assessment methods by being easy to interpret, non-manipulable, and simple to use. The answers are fact-based, concise and unambiguous, allowing for easy, quick and objective assessment. The questions are designed to be answered using the zero-shot method, which simplifies the evaluation.
GPT-4 fails at simple tasks
In the first evaluation, even advanced AI systems such as GPT-4 with plugins struggled with the GAIA benchmark.
For the simplest tasks (Level 1), GPT-4 with plugins achieved a success rate of only about 30 percent; for the most difficult tasks (Level 3), the success rate was 0 percent.
In contrast, human test subjects achieved an average success rate of 92 percent across all difficulty levels, while GPT-4 achieved an average of only 15 percent.
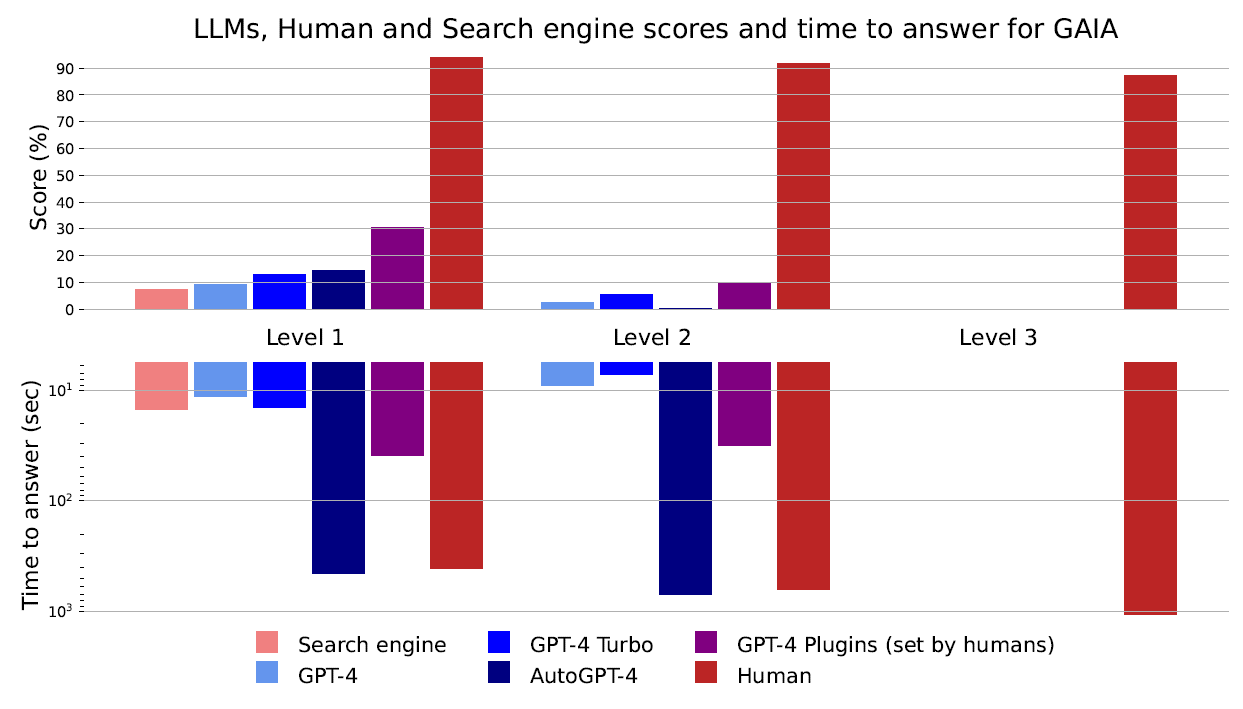
In general, GPT-4 performed best with plugins defined by humans for the task. Plugins are tools that LLMs can use when they cannot solve a task with their inherent capabilities.
The researchers see this as confirmation of the "great potential" of plugins and tool research. In February 2023, Meta AI introduced Toolformer, a large language model that automatically learns and autonomously decides how to select the best tools, such as a calculator, a question-and-answer system, or an Internet search, to solve a given task.
A study published by OpenAI in March on the impact of LLMs on the job market also made specific reference to language models that rely on tools.
However, this first evaluation has a flaw: all human testers had an academic background (Bachelor's: 61%, Master's: 26%, PhD: 17%). By contrast, only 37.7% of people over the age of 25 in the U.S. had a bachelor's degree in 2022. Whether having an academic degree has a significant impact on a person's ability to perform the tasks in the benchmark is an open question.
Standard search engine falls behind in GAIA benchmark
The research team also sees potential for using LLMs as a search engine replacement: the study notes that while human web searches can provide direct text results for Level 1 questions, from which the correct answer can be inferred, they are less effective for more complex Level 2 and Level 3 queries.
In this case, a human web search would be slower than a typical LLM assistant because the user would have to sift through the initial search results. However, this assessment does not take into account the reliability and accuracy of the search result, which is the real problem with LLMs as a search engine replacement.
Back in September 2023, a study showed that language models cannot generalize the simple logical conclusion "A is B" to "B is A," demonstrating a "fundamental failure in logical reasoning."