A new benchmark called FrontierMath, created by leading mathematicians, shows that current AI systems perform poorly on complex mathematical problems, despite high scores on simpler tests.
According to AI research firm Epoch AI, top models like o1-preview, GPT-4o, Claude 3.5, and Gemini 1.5 Pro solve less than 2 percent of FrontierMath problems, even though they score above 90 percent on previous math assessments.
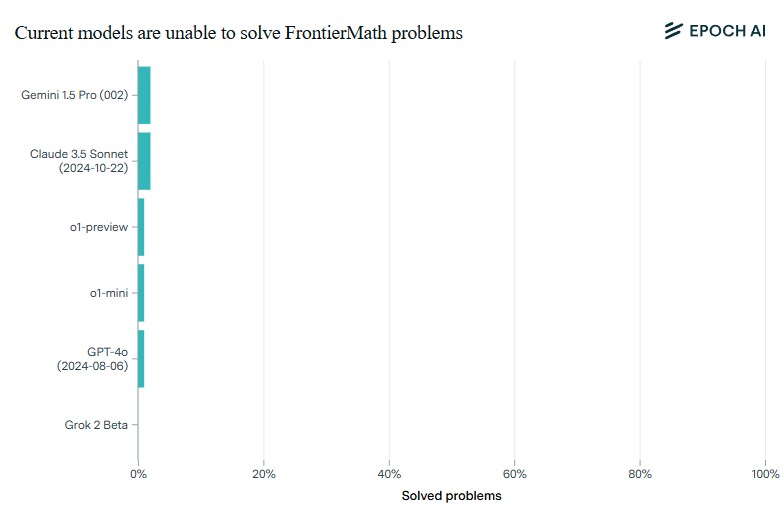
To create FrontierMath, a team of over 60 leading mathematicians put together hundreds of complex math problems. These aren't your typical math questions - they range from intensive number theory calculations to abstract problems in algebraic geometry. According to Epoch AI, even skilled mathematicians need hours or days to solve them.
Before adding any problem to the test, other experts review it to check both its accuracy and difficulty. When researchers tested random samples, they found error rates around 5 percent - about the same as other major machine learning benchmarks like ImageNet.
The benchmarking paradox
The stark difference between scores on standard tests and FrontierMath points to a core issue in AI benchmarking: Tests measure only specific, limited skills. And companies spend millions optimizing their AI models specifically for these standard benchmarks because they serve as a marketing tool.
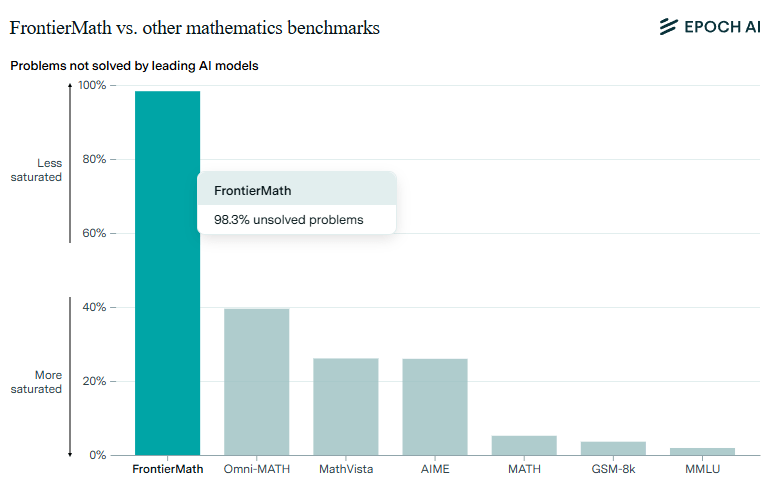
Former OpenAI developer Andrej Karpathy says these findings show a new aspect of Moravec's paradox. While AI can excel at complex tasks with clear rules - like playing high-level chess - it frequently fails at simple problems that people handle with ease. When tasks call for common sense or gut-level problem solving, AI systems fall short.
"They can solve complex closed problems if you serve them the problem description neatly on a platter in the prompt, but they struggle to coherently string together long, autonomous, problem-solving sequences in a way that a person would find very easy," Karpathy writes.
This creates an odd situation where "LLMs are inching well into top expert territory," but as Karpathy notes, "you wouldn't hire them over a person for the most menial jobs." He suggests that beyond benchmarks like FrontierMath, the field needs new tests to measure "all the 'easy' stuff that is secretly hard."
Nevertheless, the Epoch AI team sees mathematics as an ideal framework for evaluating complex reasoning. It requires both creativity and precise logical chains, while allowing for objective verification of results.
Looking ahead, the team plans to expand the benchmark and regularly test AI systems to measure their progress in mathematical reasoning. They'll also publish additional example problems over the next few months to help other researchers better understand what AI can and cannot do.