A team of researchers from the University of the Republic in Uruguay has developed an AI-based system that can intercept and reconstruct unintended electromagnetic emissions from HDMI cables. The method significantly outperforms previous approaches.
Scientists from the University of the Republic in Uruguay have unveiled a new AI system capable of intercepting electromagnetic emissions from HDMI cables and reconstructing the screen content. The method, dubbed "Deep-TEMPEST," uses deep learning to convert intercepted signals into readable images. The phenomenon of cables and connectors unintentionally emitting electromagnetic waves is known as "TEMPEST."
According to the researchers, their approach significantly outperforms previous implementations. When reconstructing text, they were able to reduce the average character error rate by over 60 percentage points.
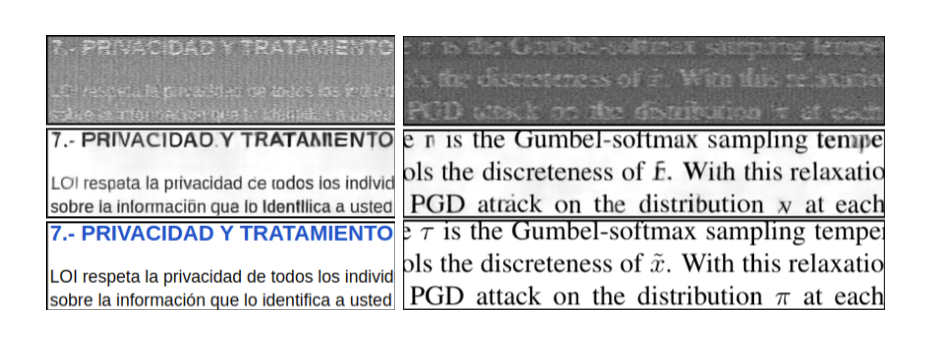
Simple countermeasures help to protect against attacks
Compared to analog interfaces like VGA, HDMI poses a greater challenge due to its 10-bit encoding and higher bandwidth. The proposed method treats the problem as an inverse problem and trains a deep neural network to translate the electromagnetic signal back into the displayed image.
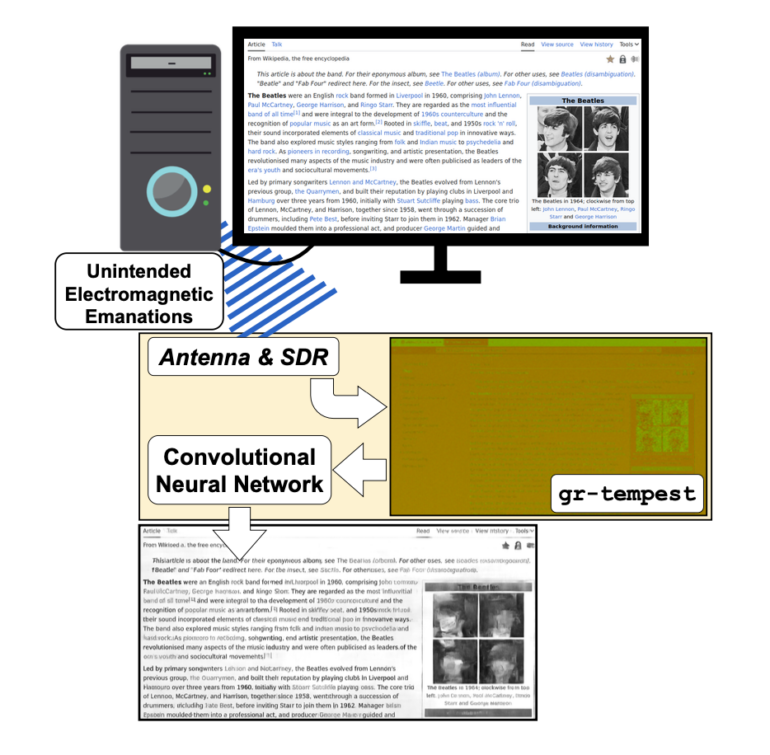
This method avoids conventional AM demodulation (a technique for extracting information from the received electromagnetic signal by analyzing its amplitude), which causes information loss, and instead uses the complex signal directly. By combining real and simulated training data, image recognition accuracy is significantly improved. The system is based on freely available Software Defined Radio (SDR) hardware and an open implementation in the GNU Radio framework.
In experiments, the system proved robust to changes in reception frequency. However, when changing screen resolution, the system needs to be retrained to achieve good performance. The researchers suggest training separate models for different configurations.
As possible countermeasures, the scientists mention adding noise to the screen content or using color gradients in the background. Both can make reconstruction significantly more difficult.
The research team sees potential for improvements, such as using multiple consecutive signal samples or a faster implementation for real-time applications. They hope their work will advance research in this field.