The context window of large language models, represented in tokens, indicates how much information an AI model can process simultaneously. Today, this window is large enough for models to summarize entire books. A new study assesses the quality of these summaries across multiple dimensions.
The context windows of large language models have been growing steadily lately, with the largest currently being Claude 3 with 200,000 tokens and Google Gemini 1.5 Pro with one million tokens.
In theory, they should be able to summarize long documents like entire novels. However, the quality of these summaries can only be judged by people who are very familiar with the extensive source material, which requires a great deal of effort.
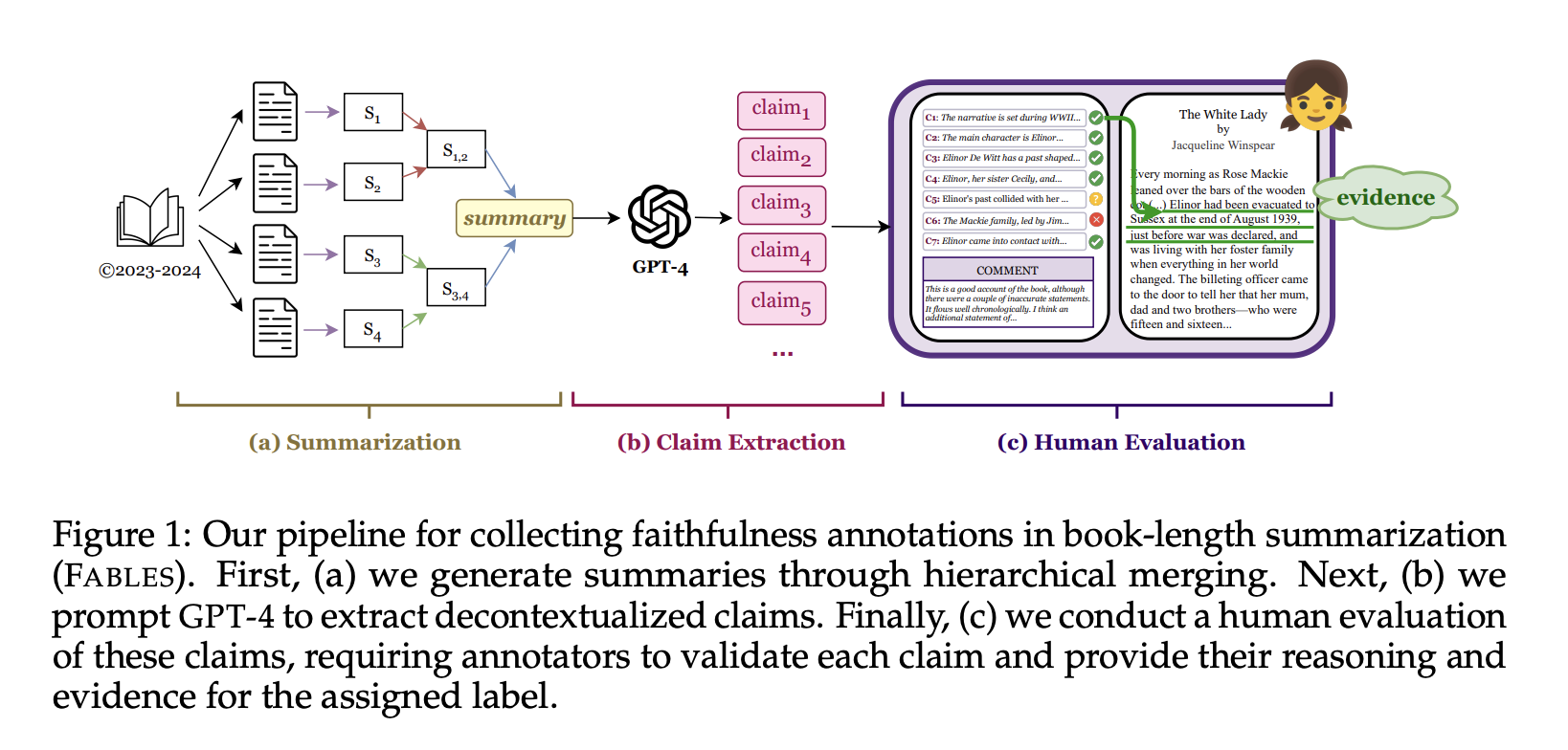
Researchers from UMass Amherst, Adobe, the Allen Institute for AI, and Princeton University have published a new dataset called FABLES (Faithfulness Annotations for Book-Length Summarization) to advance research on evaluating the reliability and accuracy of AI-generated summaries for entire books.
The researchers found that Anthropic's latest model, Claude 3 Opus, significantly outperformed all of OpenAI's closed-source LLMs, with 90 percent of assertions rated as reliable, followed by GPT-4 and GPT-4 Turbo at 78 percent, GPT-3.5 Turbo at 72 percent, and Mixtral, the only open-source model tested, just behind at 70 percent.
Analysis of the reviewers' comments showed that most of the unreliable statements related to events, characters, and relationships. Verifying the statements usually required indirect, multi-level reasoning, which the researchers said made the task even more complex.
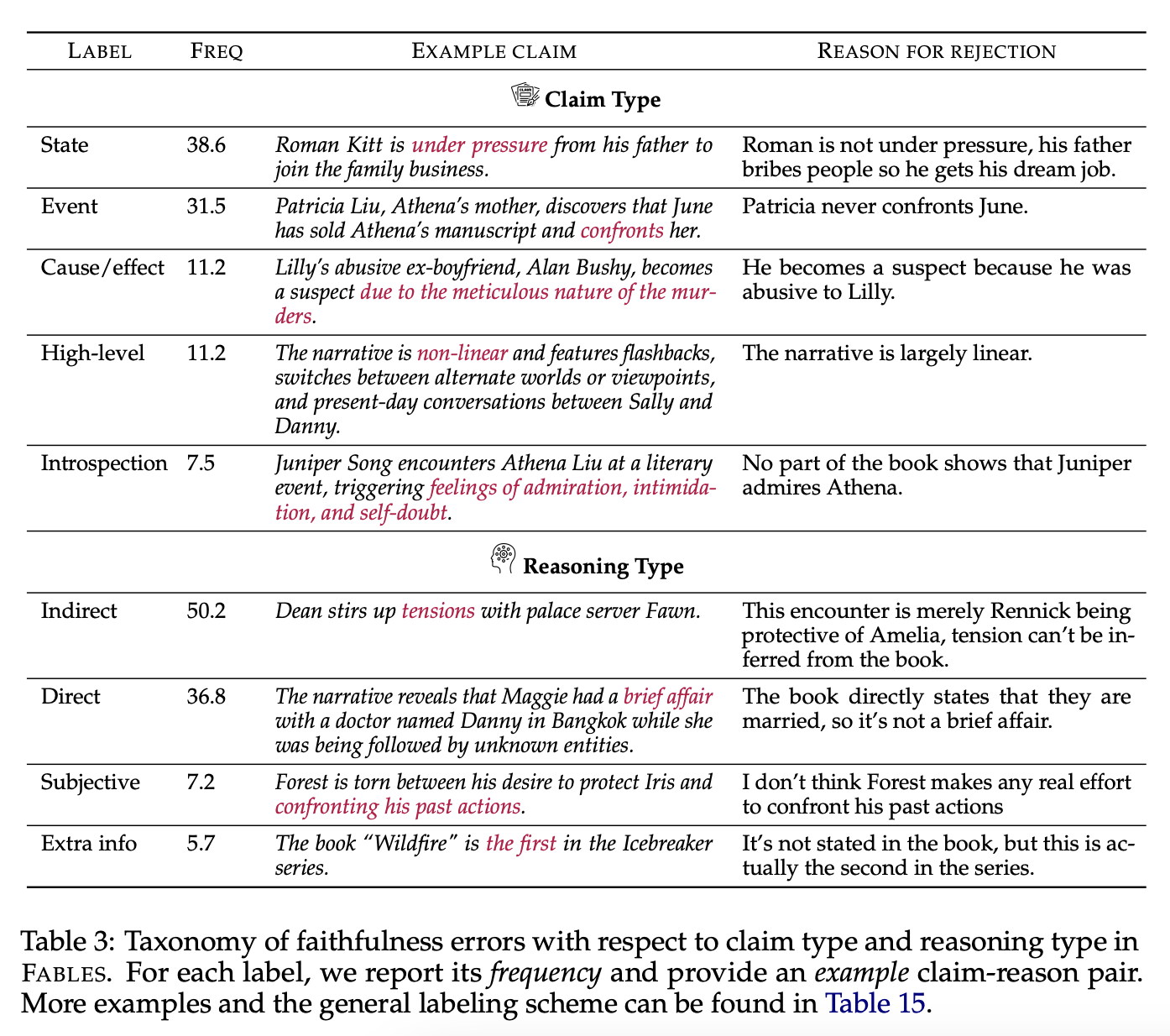
Good but difficult to scale method
The study focused on books published in 2023 and 2024 to avoid them being included in the training material and potentially skewing the results. To keep costs and cognitive load to a minimum, the annotators were asked to read the books in advance on their own time.
The researchers note that their approach is not easily scalable to new books and datasets, as the 14 human helpers recruited through Upwork cost a total of $5,200. Expanding and constantly updating the training set would therefore be very time-consuming and costly.
The researchers also experimented with using LLMs to automatically verify claims, but even their best method struggled to detect false claims reliably.
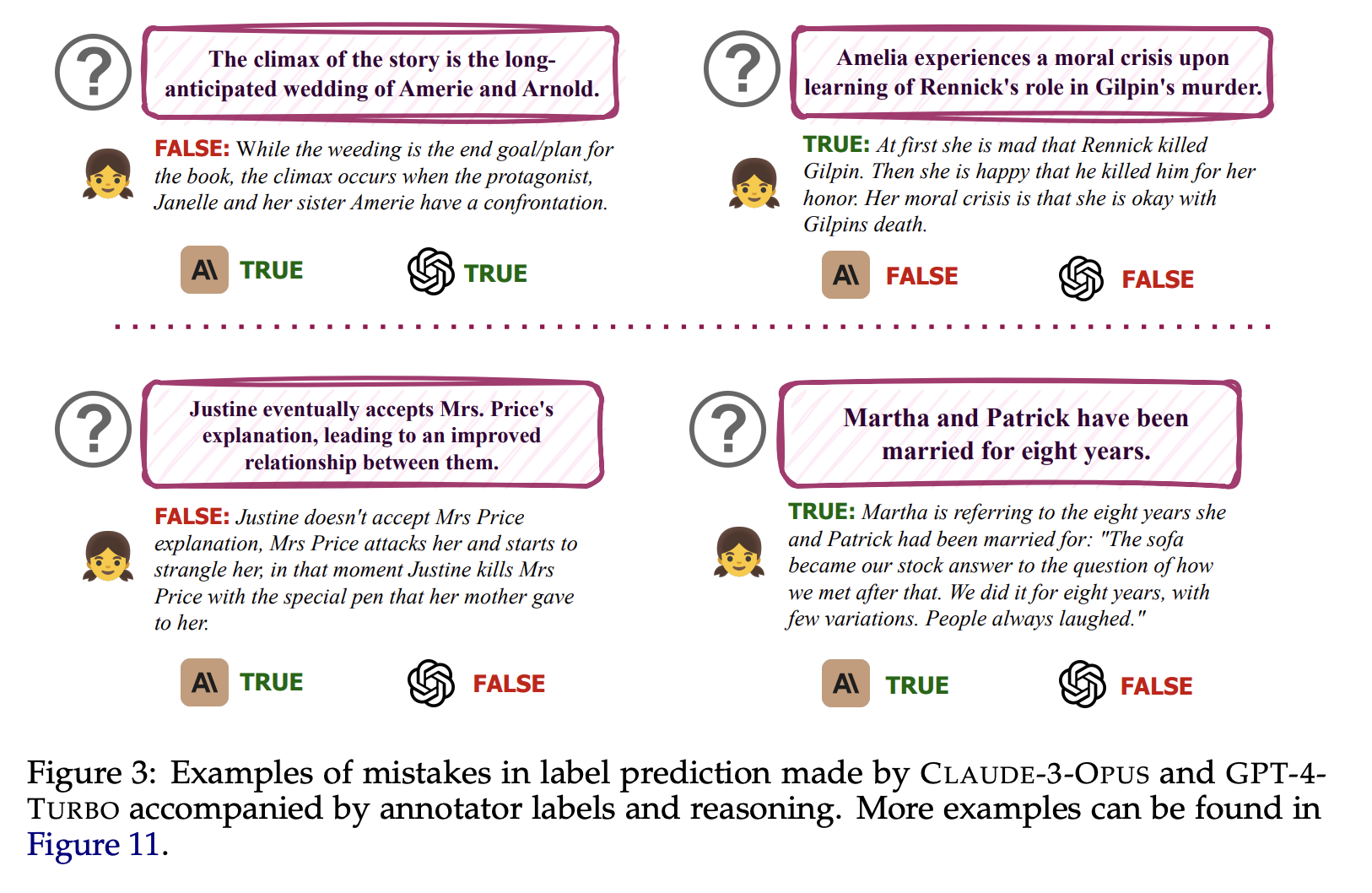
Beyond the correctness of the assertions, the researchers made other hypotheses based on the annotators' comments. In general, all language models made chronological errors, although the models with a larger context window were less affected.
All models were also criticized for omitting important information, with Claude 3 Opus performing best in this respect, while GPT-4 Turbo and Mixtral even omitted individual persons.
The researchers also confirmed the tendency previously observed in various models with very long context windows to systematically give more weight to content at the end of a book, a phenomenon known as "lost-in-the-middle".
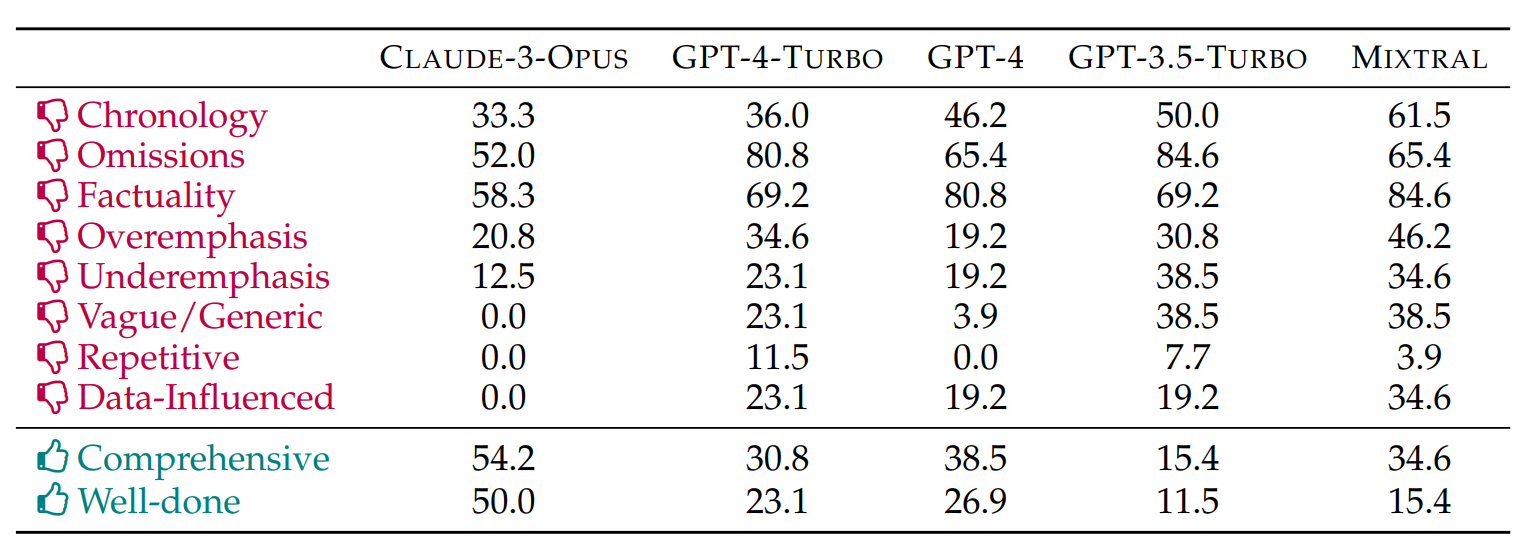
The researchers are publishing the FABLES dataset on GitHub to encourage further research of this kind.