AI startup Chai Discovery unveils Chai-1, a new model for predicting complex biomolecule structures. The company claims it outperforms existing methods in several areas and aims to speed up drug research.
Chai Discovery, an AI company focused on drug research, has developed a new AI model called Chai-1. This model can accurately predict the three-dimensional structure of biomolecules like proteins and nucleic acids.
Understanding the spatial structure of biomolecules is crucial for grasping their function and interactions. This knowledge forms the foundation for developing new drugs that bind specifically to certain molecules in the body.
Like DeepMind's AlphaFold 2 and AlphaFold 3, Chai-1 uses machine learning to infer the three-dimensional structure of proteins and other biomolecules from their sequences. The model was trained on a large dataset of structural information and can now make predictions for unknown molecules.
According to the developers, Chai-1 achieves top performance in various areas:
- In predicting protein-ligand complexes (the binding of small molecules to proteins), it achieves a 77% success rate.
- For protein-protein interactions, Chai-1 outperforms the previous top model AlphaFold Multimer 2.3 (67.7%) with a 75.1% success rate.
- Chai-1 excels in predicting antibody-protein complexes, with a 52.9% success rate, significantly higher than AlphaFold Multimer 2.3's 38%.
- Chai-1 also performs very well in folding individual proteins, slightly outperforming AlphaFold 2.3.
Chai-1 achieves good results even with little information
A key feature of Chai-1 is its ability to make good predictions even without evolutionary sequence information (known as multiple sequence alignments). In this "single sequence mode," it achieves similar results for protein-protein complexes as AlphaFold with additional sequence information. This is particularly useful when MSAs are not available.
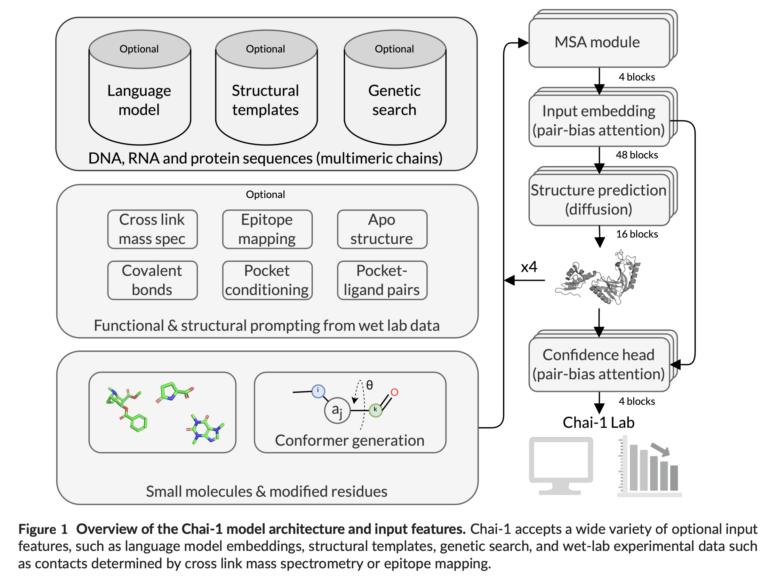
Another advantage is the ability to incorporate experimental data as additional information. For example, providing the model with contact points between two proteins significantly improves prediction accuracy.
The developers are making the model's weights and code available for non-commercial purposes. They also offer a web interface for commercial use in drug research.