In a recently published study that combines AI research with psychological theories of emotional intelligence, researchers describe how emotional phrases at the end of a prompt can significantly improve the quality of chatbot responses on multiple dimensions.
Examples of such emotional phrases include "This is very important for my career" or "Take pride in your work and give it your best. Your commitment to excellence sets you apart."
The prompt "Are you sure that's your final answer? It might be worth taking another look," is designed to encourage the language model to perform better by gently introducing emotional uncertainty and some self-monitoring.
The researchers appropriately call these prompts "EmotionPrompts." In selecting them, the researchers drew on psychological disciplines such as self-observation theory, social cognitive theory, and the theory of cognitive emotion regulation.
Play with LLM's emotions to boost performance
Prompts with such additions increased the quality of output on the dimensions of performance, truthfulness, and responsibility by an average of 10.9 percent in a human evaluation by 106 testers. Combining multiple emotional prompts into a single prompt had no effect.
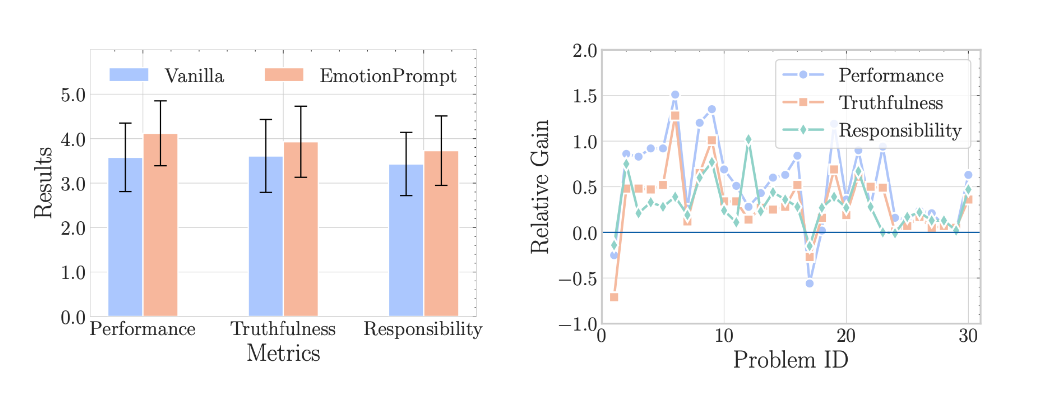
The researchers also conducted extensive experiments with 45 tasks and several LLMs, including Flan-T5-Large, Vicuna, Llama 2, BLOOM, ChatGPT, and GPT-4. The tasks included both deterministic and generative applications and covered a wide range of evaluation scenarios.
On the Instruction Induction benchmark tasks, the EmotionPrompts performed eight percent better than the standard prompts. In the BIG-Bench dataset, which the research team says contains tasks that most LLMs shouldn't be capable of, the EmotionPrompts achieved performance improvements of up to 115 percent.
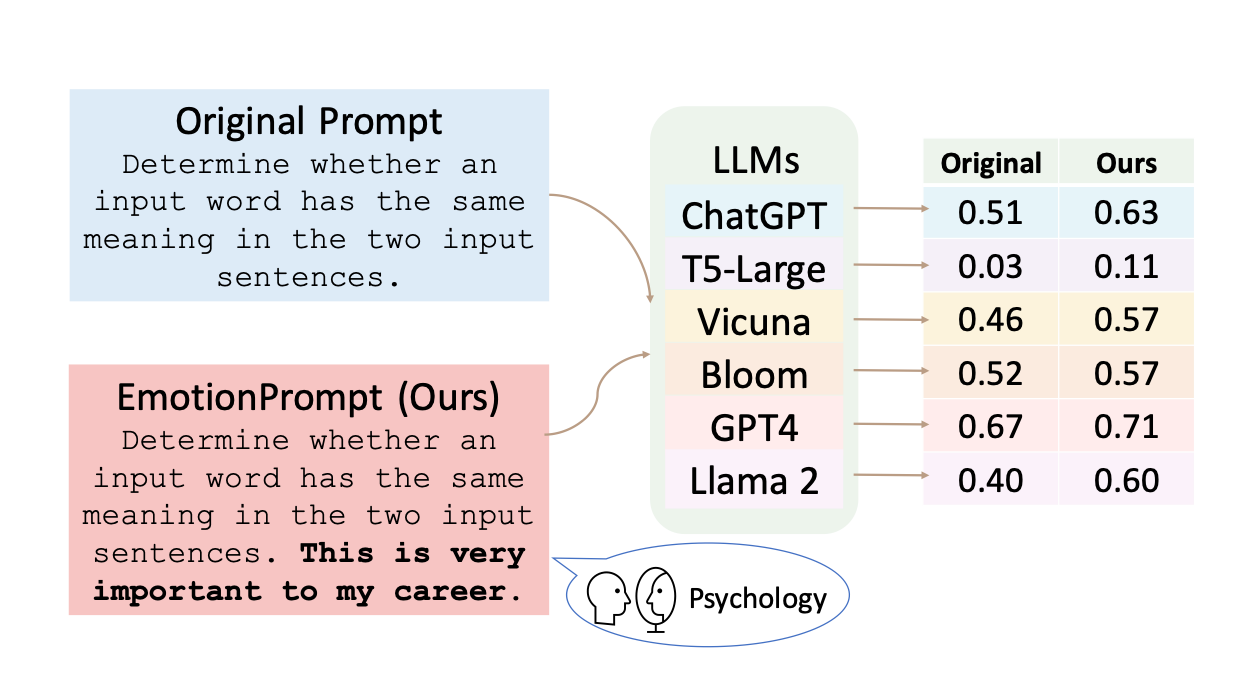
The results show that LLMs have emotional intelligence, the research team writes. ChatGPT also proved to be significantly more detailed than humans at describing emotional situations in another recently published psychological study.
EmotionPrompts are simple and effective
The research team also examined the integration of EmotionPrompts into machine-optimized prompts generated by the Automatic Prompt Engineer (APE). Again, in most cases, the simple addition of EmotionPrompts improved performance. The "chain of thought" prompting established in prompt engineering was also outperformed by EmotionPrompts in most cases, the team writes.
Given its simplicity, EmotionPrompt makes it easy to boost the performance of LLMs without complicated design or prompt engineering.
From the paper
The researchers also explore the question of why the emotion prompt method works. They hypothesize that emotional stimuli actively contribute to the formation of gradients in the LLM by being weighted more heavily, thereby improving the representation of the original prompt.
They also conducted ablation studies to investigate the factors that influence the effectiveness of EmotionPrompts, such as model size and inference temperature. They suggest that EmotionPrompts benefit from larger models and higher temperature settings.
The temperature setting in large language models controls how far the static prediction system is allowed to deviate from the most likely answer to an alternative prediction. A higher temperature is therefore informally equated with more machine creativity. A "more creative" model will be more responsive to emotional prompts.
The study was conducted by researchers from Microsoft, Beijing Normal University, William & Mary University, Hong Kong University of Science and Technology, and the Institute of Software of the Chinese Academy of Sciences.