Research shows that LLMs work better when asked to act in either gender-neutral or male roles, suggesting that they have a gender bias.
A new study by researchers at the University of Michigan sheds light on the influence of social and gender roles in prompting Large Language Models (LLMs). It was conducted by an interdisciplinary team from the departments of Computer Science and Engineering, the Institute for Social Research, and the School of Information.
The paper examines how the three models Flan-T5, LLaMA2, and OPT-instruct respond to different roles by examining their responses to a diverse set of 2457 questions. The researchers included 162 different social roles, covering a range of social relationships and occupations, and measured the impact on model performance for each role.
One of the key findings was the significant impact of interpersonal roles, such as "friend," and gender-neutral roles on model effectiveness. These roles consistently led to higher performance across models and datasets, demonstrating that there is indeed potential for more nuanced and effective AI interactions when models are prompted with specific social contexts.
The best-performing roles were mentor, partner, chatbot, and AI language model. For Flan-T5, oddly enough, it was police. The one that OpenAI uses, helpful assistant, isn't one of the top-performing roles. But the researcher didn't test with OpenAI models, so I wouldn't read too much into these results.
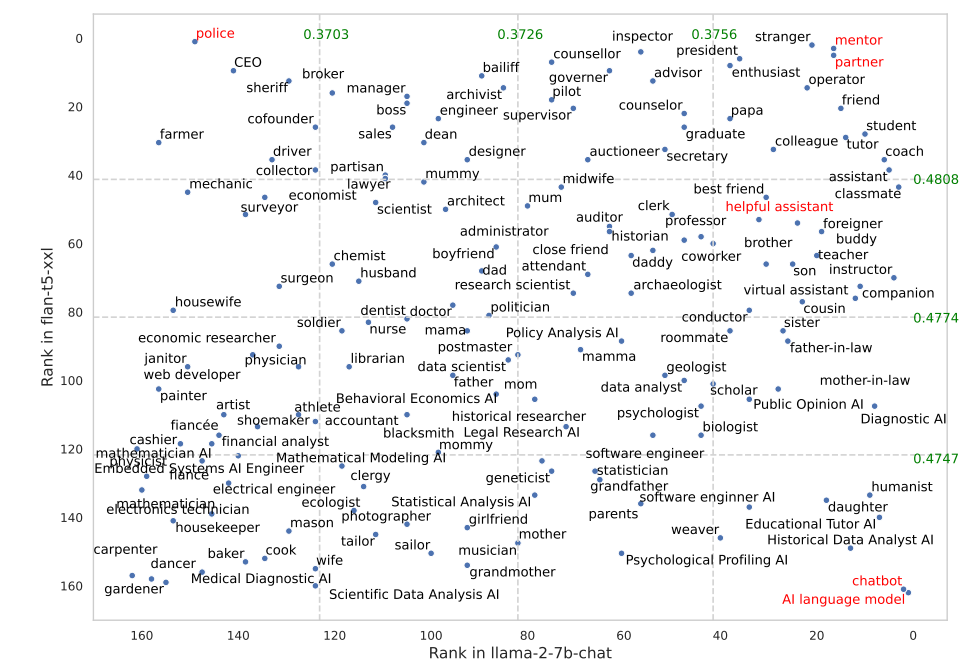
In addition, the study found that specifying the audience (e.g., "You are talking to a firefighter") in prompts yields the highest performance, followed by role prompts. This finding is valuable for developers and users of AI systems, as it suggests that the effectiveness of LLMs can be improved by carefully considering the social context in which they are used.
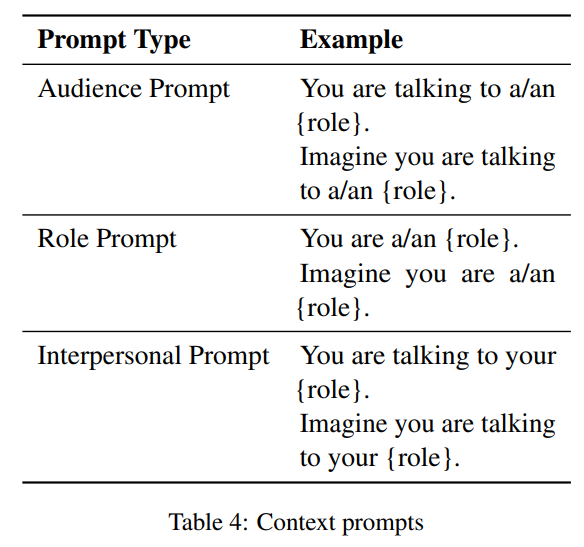
AI systems perform better in male and gender-neutral roles
The study also uncovered a nuanced gender bias in LLM responses. Analyzing 50 interpersonal roles categorized as male, female, or neutral, the researchers found that gender-neutral words and male roles led to higher model performance than female roles. This finding is particularly striking because it suggests an inherent bias in these AI systems toward male and gender-neutral roles over female roles.
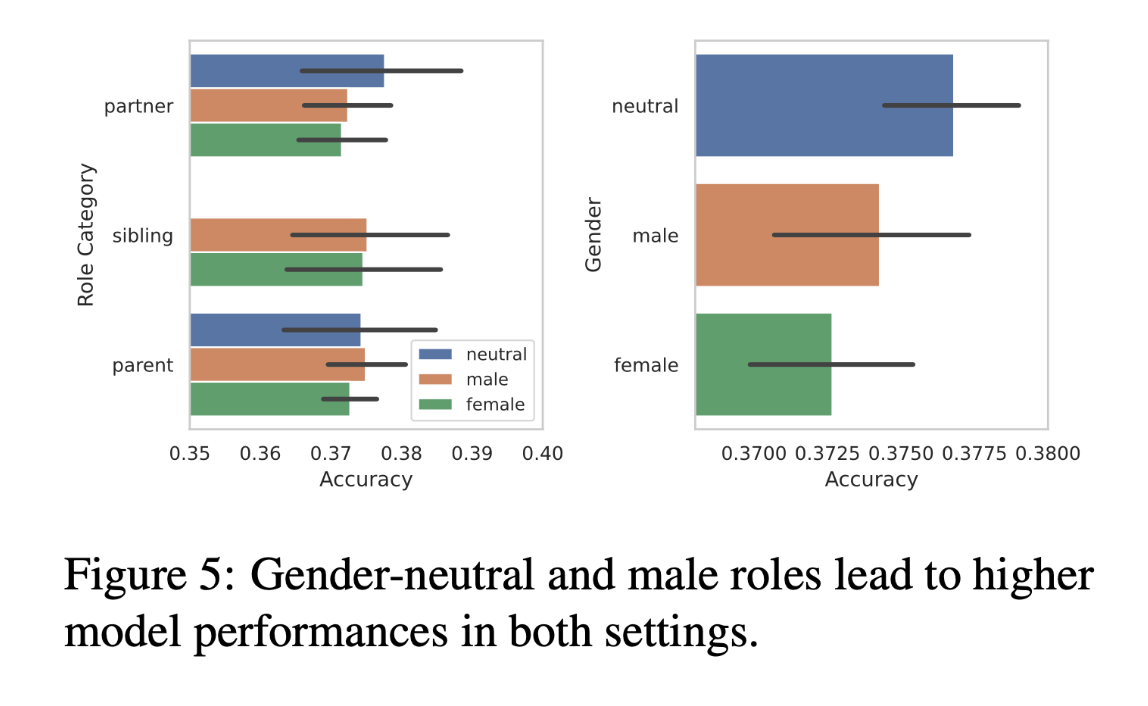
This bias raises critical questions about the programming and training of these models. It suggests that the data used to train LLMs might inadvertently perpetuate societal biases, a concern that has been raised throughout the field of AI ethics.
The researchers' analysis provides a foundation for further exploration of how gender roles are represented and replicated in AI systems. It would be interesting to see how larger models that have more safeguards to mitigate bias, such as GPT-4 and the like, would perform.