Google DeepMind has developed a new approach to data collection that can be used to quickly gather large amounts of data from real-world interactions. The aim is to improve the ability of robots to successfully complete more complex tasks.
RoboVQA uses a "crowd-sourced bottom-up" approach to data collection. Specifically, data, including egocentric videos, will be collected from humans, robots, and humans controlling a robot arm for a variety of tasks.
RoboVQA learns from humans and machines
The process starts with detailed instructions for household tasks such as "make me a coffee" or "tidy up the office". Robots, humans, and humans with robotic arms then carried out the tasks in three office buildings.
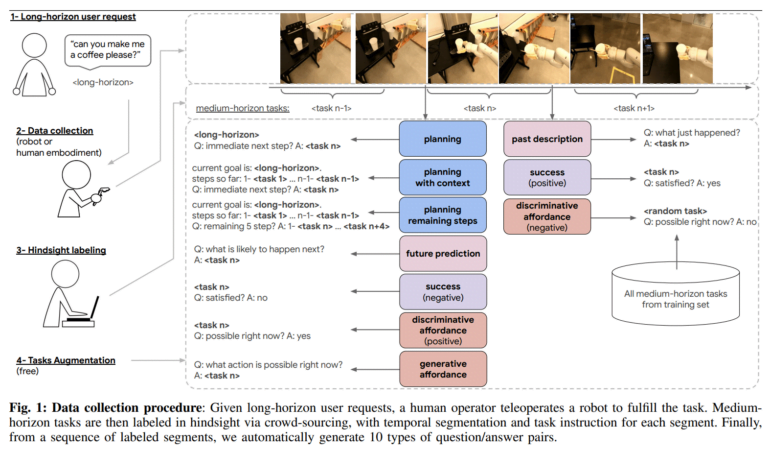
Image: Google Deepmind
Crowdworkers then used the videos to break down the lengthy tasks into shorter segments with natural language descriptions such as 'take the coffee beans' or 'turn on the coffee maker'. This resulted in more than 829,502 videos with detailed instructions. According to Deepmind, this method enables much faster data collection than other methods that do not rely on crowdsourcing.
RoboVQA-VideoCoCa outperforms other robot models
The researchers also showed that the collected data actually adds value. The team trained the RoboVQA-VideoCoCa model, which performed a variety of tasks in realistic environments significantly better than other approaches based on other vision-language models (VLMs). Compared to these, the robots required 46 percent less human intervention. According to the team, this is a significant step forward, but it also shows that there is still a lot of data to be collected.
Video: Google Deepmind
In another test, the team also showed that the model's errors can be reduced by almost 20 percent by using a video VLM instead of a VLM that only analyses individual images.
Meanwhile, another team of AI researchers presented RoboGen, a method for automatically generating training data for robots in simulations.
All information and data are available on GitHub.