A new simulator from Epoch AI shows that training models on the scale of GPT-4 would have been possible with older hardware, but at a significantly higher cost.
The simulator analyzes how efficiently a model uses FLOP (Floating Point Operations Per Second) compared to the computing power needed for training. Epoch AI's research shows that efficiency on the same hardware tends to decrease as models grow larger.
The company's data also reveals different patterns of efficiency across GPU generations. While newer architectures like the H100 can maintain higher efficiency rates for longer periods of time, older GPUs like the V100 show a steeper decline in efficiency as training size increases.
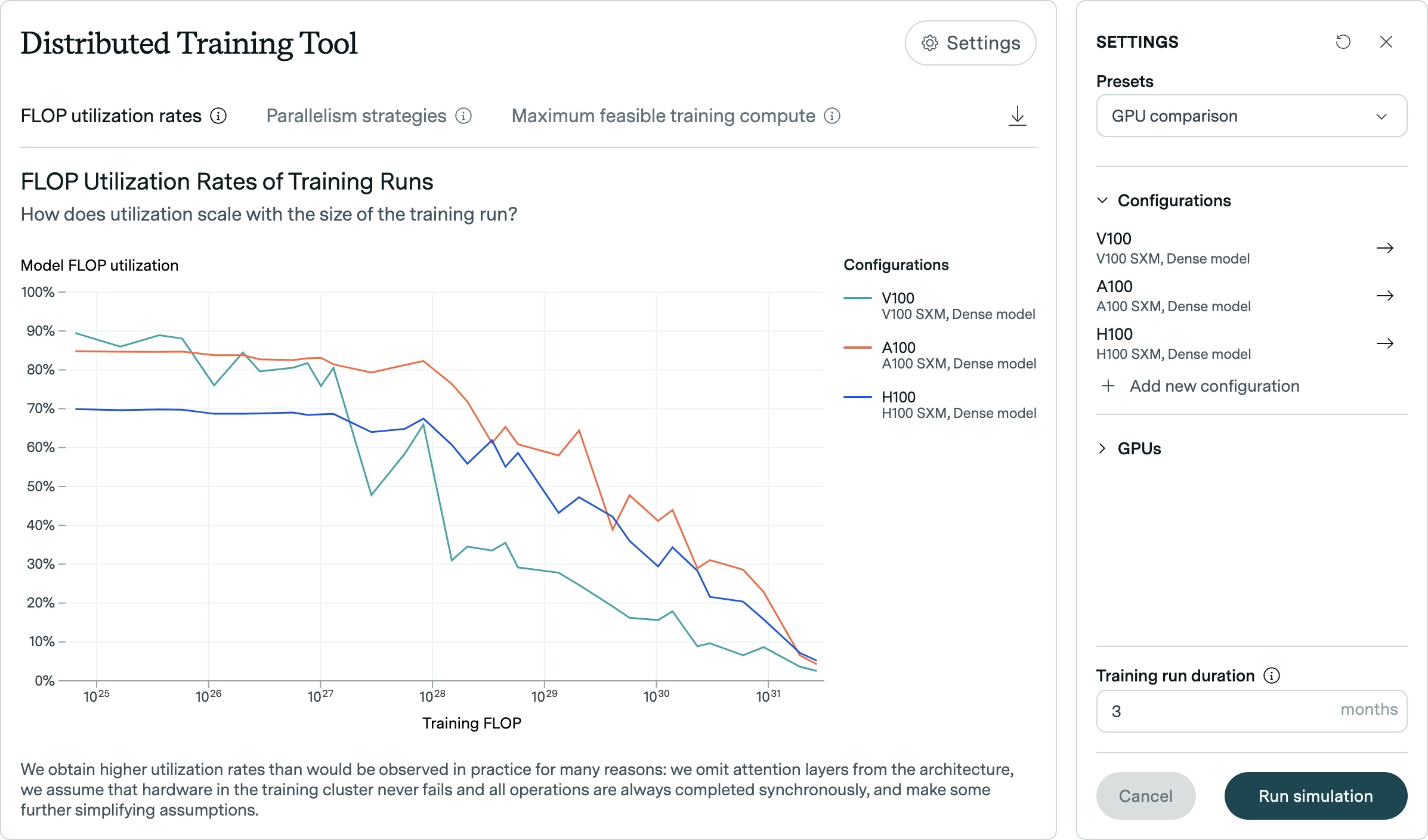
2012 tech could handle GPT-4
Epoch AI conducted an experiment simulating training on a GTX 580 GPU with 3 GB of memory. This was the same graphics card researchers used to train the groundbreaking AlexNet model in 2012.
The researchers estimate that GPT-4's training requires between 1e25 and 1e26 floating-point operations (FLOP). The simulation indicates this scale of training could have been achieved with 2012 technology, though at approximately ten times the cost of using modern hardware.
Understanding future hardware needs
The simulator enables complex simulations for training across multiple data centers. Users can specify the size of the data centers, the latency, and the bandwidth of the connections between the data centers. These parameters allow researchers to simulate how training runs could be distributed across multiple locations.
The tool also allows users to analyze performance differences between modern GPUs, such as the H100 and A100, as well as the effects of different batch sizes and training across multiple GPUs. The system generates detailed log files showing the output of the model.
Epoch AI says it developed the simulator to improve understanding of advances in hardware efficiency and to assess the impact of chip export controls. The company's goal is to increase understanding of the hardware requirements needed for large-scale training runs expected this decade.