Update November 1, 2024: Correction
I misinterpreted an important methodological aspect of the OpenAI study. SimpleQA's test questions were chosen specifically because they cause problems for AI models - every question included was one that tripped up at least one of the models used during data creation (GPT-4 models of various release dates).
This selective approach means that low accuracy scores reflect performance on particularly challenging questions, not the AI models' overall capabilities. By focusing only on questions where the models sometimes failed, the benchmark naturally produced lower scores.
I apologize for my mistake and the misleading interpretation. The article has been updated to reflect this change.
Article dated October 30, 2024:
The SimpleQA test includes 4,326 questions across science, politics, and art. Each question was designed to have only one clearly correct answer, verified by two independent reviewers.
The low percentage of correct answers must be understood in the specific context of SimpleQA's methodology: The researchers only included questions where at least one of the GPT-4s used to generate most of the data gave an incorrect answer. This also means that the reported percentages reflect the performance of the models on particularly difficult questions, not their general ability to give correct answers to factual questions.
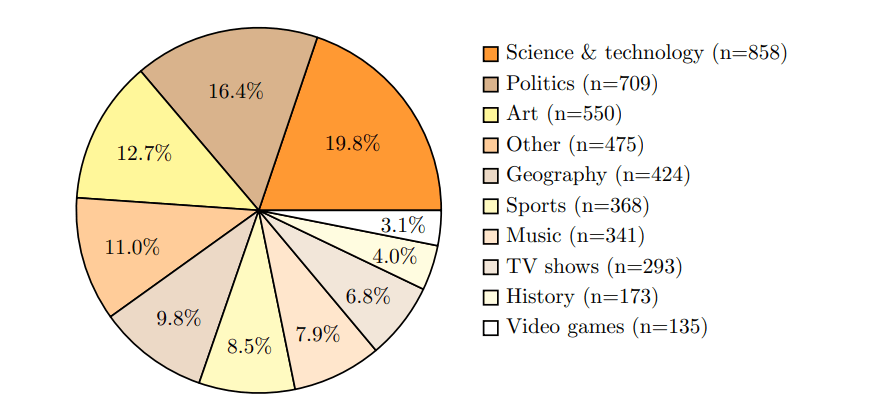
OpenAI's best model, o1-preview, achieved a 42.7 percent success rate. GPT-4o followed with 38.2 percent correct answers, while the smaller GPT-4o-mini managed just 8.6 percent accuracy.
Anthropic's Claude models performed worse. Their top model, Claude-3.5-sonnet, got 28.9 percent right and 36.1 percent wrong. However, smaller Claude models more often declined to answer when uncertain – a desirable response that shows they recognize their knowledge limitations.
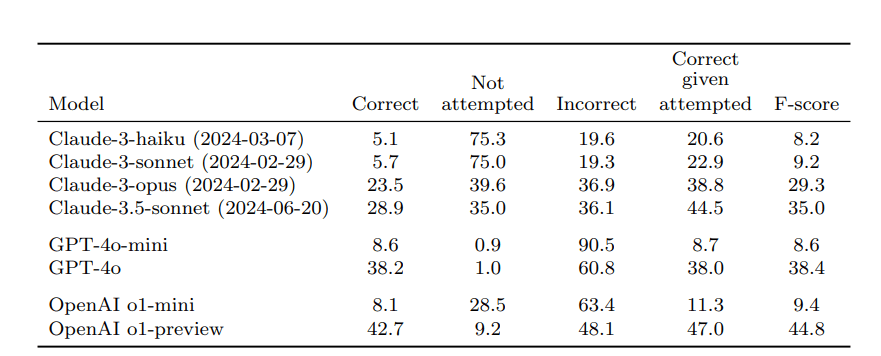
Note that the test specifically measures knowledge acquired during training. It does not assess the models' general ability to provide correct answers when given additional context, Internet access, or database connections.
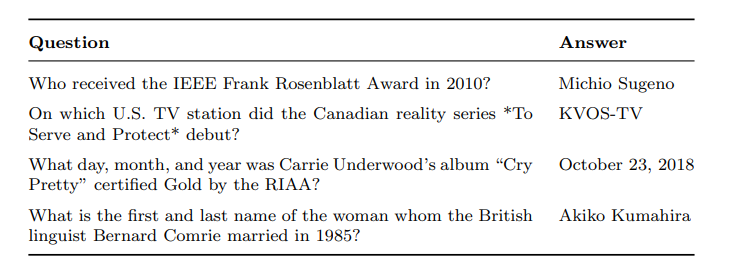
AI models overestimate themselves
The study shows also shows that AI language models significantly overestimate their own capabilities when answering questions. When researchers asked the models to rate their confidence in their answers, the AIs consistently gave inflated scores about their own accuracy.
To measure this overconfidence systematically, researchers had the models answer identical questions 100 times each. They found that when a model gave the same answer repeatedly, it was more likely to be correct - but even then, actual success rates remained lower than what the models predicted about their own performance. This finding fits with the common criticism that language models can answer complete nonsense while acting like they know it's right.
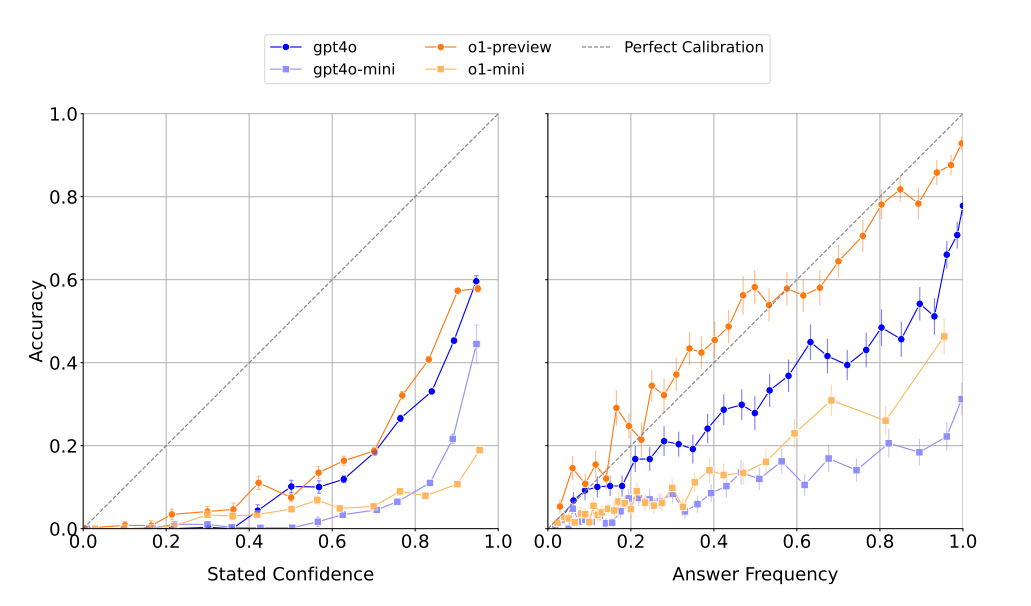
The researchers note significant gaps in current AI systems' factual accuracy that need addressing. They also point out an open research question: whether an AI's performance on short factual answers predicts how well it handles longer, more detailed responses containing multiple facts.
OpenAI has released its SimpleQA benchmark on Github to help researchers develop more reliable language models.