AI models break benchmark after benchmark in computer vision - but in the real world, they continue to show weaknesses and lag behind humans. Why is that?
MIT researchers show that current training datasets often use images that depict objects so clearly that humans - and machines - can easily recognize them. But what constitutes a "difficult" image? The researchers suggest using the time it takes a human to identify an object in an image as a measure.
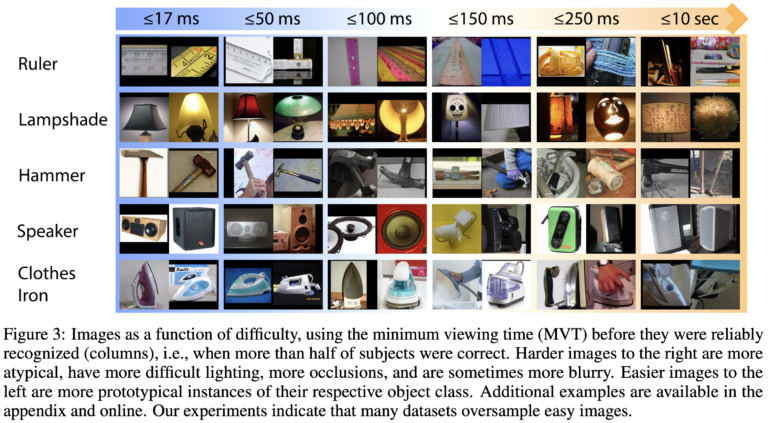
The Minimum Viewing Time (MVT) metric developed by the team is designed to quantify the difficulty of recognizing an image. The researchers used a subset of the ImageNet and ObjectNet datasets to show images for different durations, ranging from 17 milliseconds to 10 seconds, and asked participants to select the correct object from 50 options. After more than 200,000 runs, the researchers found that the test sets were biased toward simpler, shorter MVT images, so that most of the benchmark performance came from images that were easy for humans to recognize.
The team also showed that larger models like the Vision Transformer performed better on simpler images than smaller models, but made less progress on more difficult images.
"Minimum viewing time" could enable more robust AI models
Co-author Jesse Cummings emphasizes the importance of MVT for evaluating AI models: "We want models that are able to recognize any image even if — perhaps especially if — it’s hard for a human to recognize. We’re the first to quantify what this would mean."
Mayo and his team are also investigating the neurological basis of visual recognition, looking at whether the brain shows different activity when processing simple and difficult images.
"This comprehensive approach addresses the long-standing challenge of objectively assessing progress towards human-level performance in object recognition and opens new avenues for understanding and advancing the field," says co-author David Mayo. The ability to use MVT as a metric of task difficulty for many different computer vision tasks could pave the way for more robust and human-like performance in object recognition.