With Med-PaLM M, Google Deepmind introduces a multimodal variant of its Med-PaLM medical AI model series that can process text, medical images, or even genomes for diagnosis.
Med-PaLM M (MPM) is based on PaLM-E, Google's robot model that combines language and vision, and is not a multimodal evolution of Med-PaLM 2, Google's large language model that has been refined for medical tasks. This makes sense since Med-PaLM M is supposed to be able to make diagnoses from visual data.
Just as Med-PaLM 2 is a variant of the foundational language model PaLM 2 refined with medical data, MPM is a variant of PaLM-E refined with medical data.
All-purpose AI doctor
Google Deepmind calls MPM a step toward a general-purpose biomedical model. Think of it as a kind of universal doctor that has an appropriate diagnosis or answer ready for all medical topics and images.
Med-PaLM M processes a variety of medical information. Like Med-PaLM 2, it can simply answer questions and comes close to the level of Med-PaLM 2. It can also examine X-ray images or even scan DNA sequences for mutations.
In almost all disciplines, Med-PaLM M matches the current state-of-the-art performance of specialized systems and even sets new standards in some areas, such as x-ray diagnostics or answering visual questions.
To test the capabilities of the AI model, the research team created MultiMedBench, a multimodal benchmark with 14 different tasks from seven multimedical disciplines. MultiMedBench includes more than one million examples and is designed to advance the development of biomedical AI.
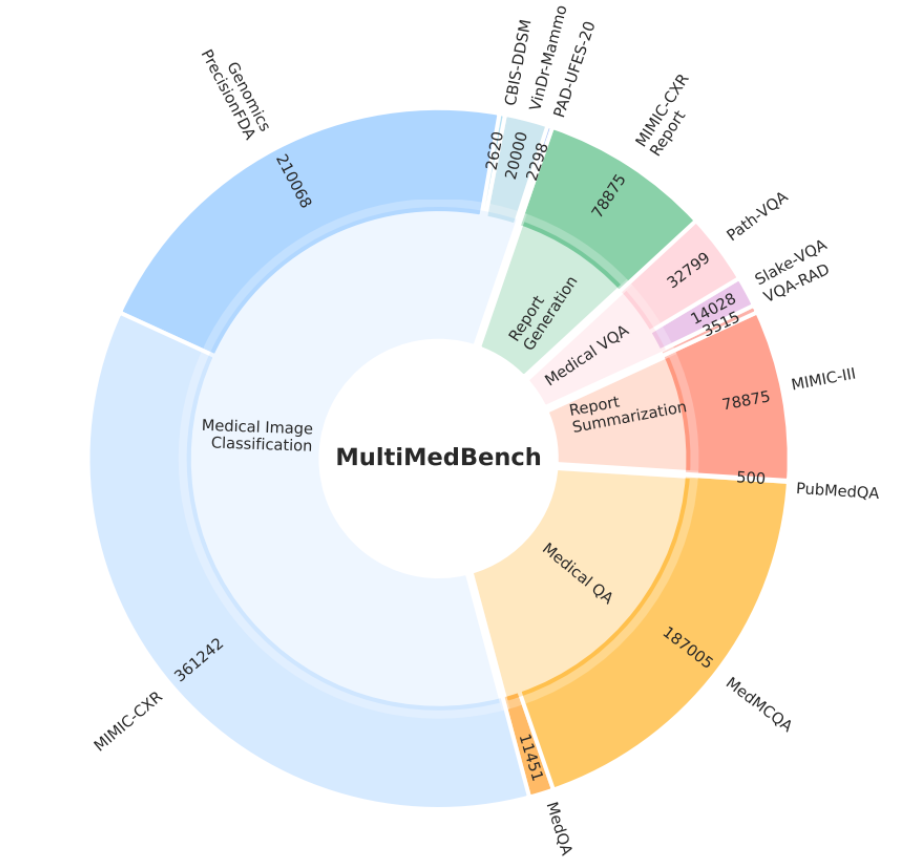
MPM shows potential for medical generalization
The research team extensively tested Med-PaLM M's ability to diagnose human chest X-rays. In approximately 40 percent of cases, clinicians preferred the AI-generated X-ray reports in a blinded test.
MPM produced 0.25 clinically significant errors per report, which is on par with human experts and should allow for clinical use.
The research team also highlights MPM's zero-shot capability, the ability to generalize to new tasks without explicit examples, using only natural language instructions.
For example, Med-PaLM M can accurately recognize and describe new medical concepts, such as tuberculosis on chest X-rays, even though it has never been trained on such examples. MPM could therefore be useful in cases where there is little medical example data.
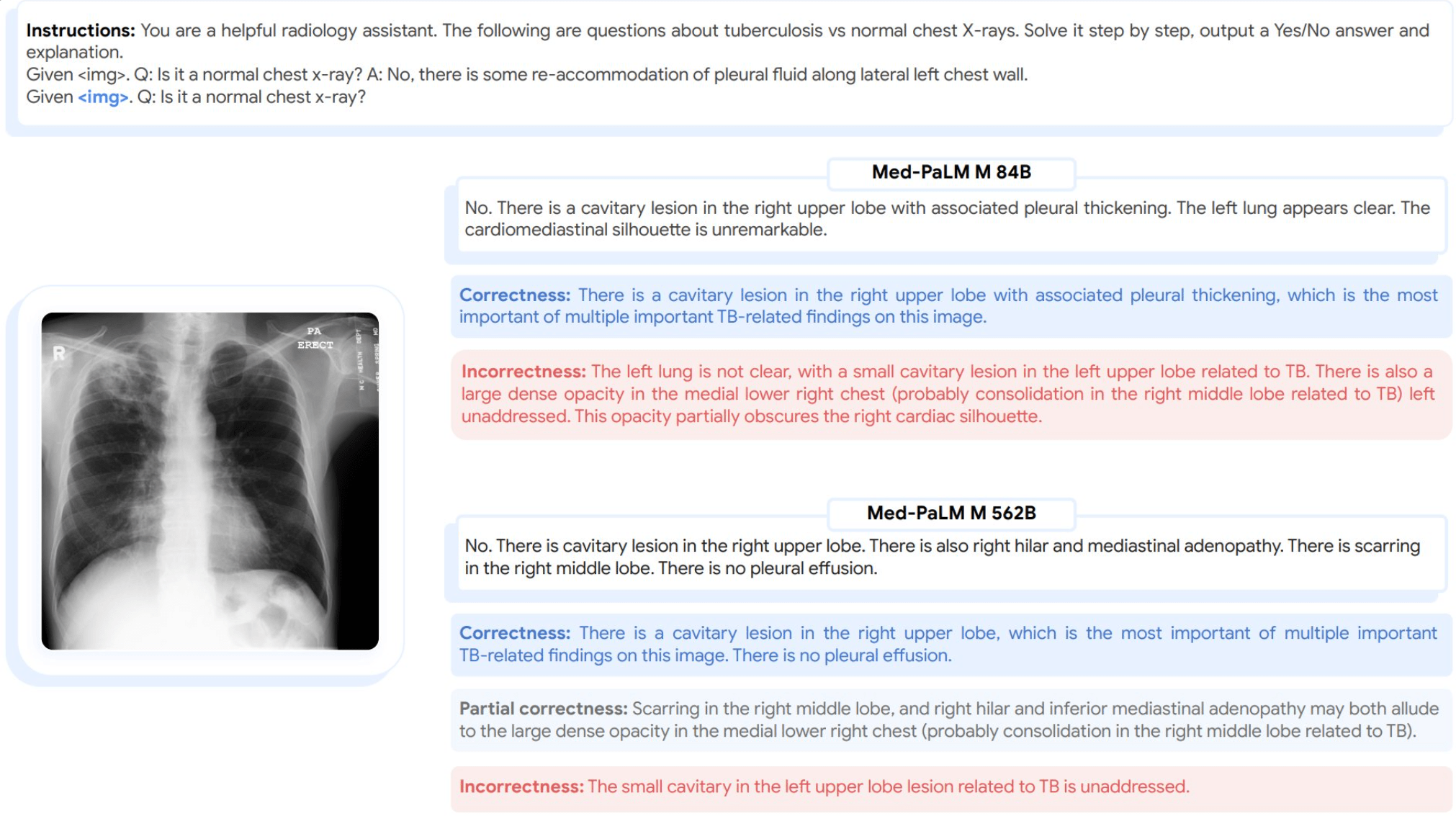
Further development and "rigorous validation" are needed, the team writes, but they see MPM as an "important step" toward general biomedical AI. Other challenges include the high-quality and sometimes rare data needed for scaling, and benchmarking needs to be greatly expanded. The MultiMedBench presented is still limited in scope and variety of possible tasks, the researchers write.