Meta's PEER is designed to simplify collaboration with large language models, opening them up to text collaborations with humans.
Large language models like OpenAI's GPT-3 show impressive generative capabilities. They can write prose, poetry, HTML code, or Excel spreadsheets. And, their results are sometimes indistinguishable from human-generated content. Such AI models generate their output from left to right - they supplement input text provided by humans according to learned probabilities.
Input text triggers the models. Translation examples lead to further translations. The program poetically completes a sonnet prompt. Or it thematically presents a story about the tension between anarcho-syndicalism and classical monarchies.
Large language models are hard to control
Away from this simple bump, the ability to control the output of the models is severely limited. Further, reviewing the generated text is difficult because they often generate strange content and cannot explain why.
Companies such as OpenAI, Google, and Meta are therefore looking at approaches to make the models more reliable. Google, for example, is relying on fact training vs. AI nonsense, and OpenAI is experimenting with search engine access.
Despite such attempts, current language models are soloists. Text collaboration with human authors is not possible. AI researchers at Meta want to change that and are now introducing PEER. The acronym stands for "Plan, Edit, Explain, Repeat" and is the name of a new collaborative language model trained with editing processes from Wikipedia, for example.
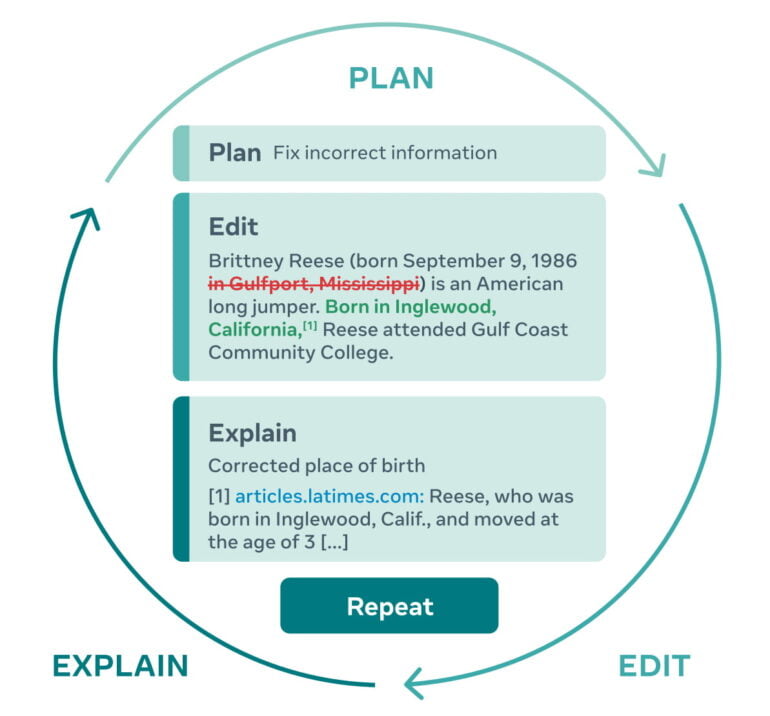
PEER is intended to cover the entire writing process and can, for example, edit texts and explain changes that have been made.
Meta shows what collaboration between humans and PEER could look like
PEER works in several steps that, according to Meta, mirror the human writing process. For a given text, a human or the AI model can create a plan, for example, via text input. Such a plan might include correcting misinformation contained in the text, adding source citations, or formatting changes.
The model can then explain these changes, such as with a reference to a source. For this purpose, each input text is provided with possibly relevant background information before it is further processed by PEER.
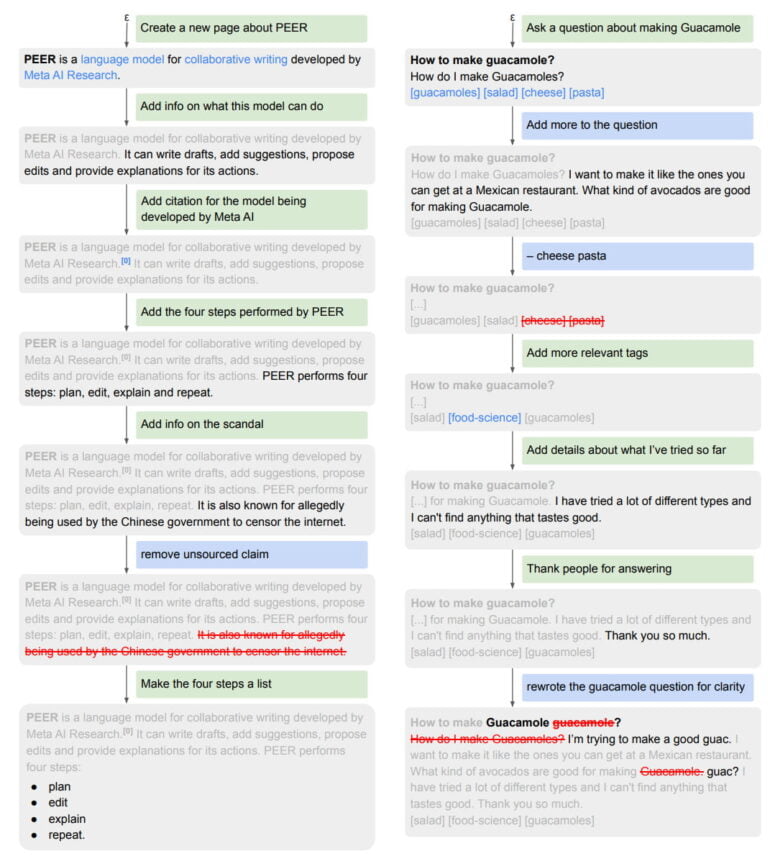
Human authors repeat this process as many times as needed. This iterative approach divides the complex task of writing a consistent and factual text into several simpler subtasks. Between any of these subtasks, humans can also intervene or take over.
PEER learns with Wikipedia and is just the beginning for Meta
For training, Meta draws on Wikipedia's extensive dataset of text edits and associated comments, often getting explanations for those changes. To make PEER useful outside of Wikipedia-like texts, variants of PEER also learn to reconstruct the original, non-edited text from a finished edited text and documents relevant to it.
The three billion parameter PEER performs better as a writing assistant than other and significantly larger language models such as GPT-3 or Meta's OPT. Still, all of this only scratches the surface of PEER's capabilities as a collaborative language model, Meta said. In the future, the team plans to collect complete sessions of numerous AI-human interactions to improve PEER.
To do so, the team must also solve current limitations of the model, such as providing access to a real-world retrieval engine that can find relevant documents on its own, developing methods for evaluating text co-authored by humans and language models, and making PEER more efficient so it can process entire documents.