Meta AI has developed an AI system that uses magnetoencephalography (MEG) to decode visual representations in the brain. In the long term, this could pave the way for non-invasive brain-computer interfaces.
The AI system can reconstruct almost in real time how images are perceived and processed in the brain. It provides science with a new tool for understanding how images are represented and used as the basis of human intelligence.
In the long term, this research could pave the way for non-invasive brain-computer interfaces in clinical settings and help people who have lost the ability to speak due to brain injury, the Meta researchers write.
Three-Part AI System
Meta AI's system is based on its recently developed architecture for decoding speech perception from MEG signals. The new system consists of an image encoder, a brain encoder, and an image decoder.
The image encoder generates a set of representations of an image independently of the brain, while the brain encoder matches the MEG signals to these image encodings. Finally, the image decoder generates a plausible image based on the brain representations.
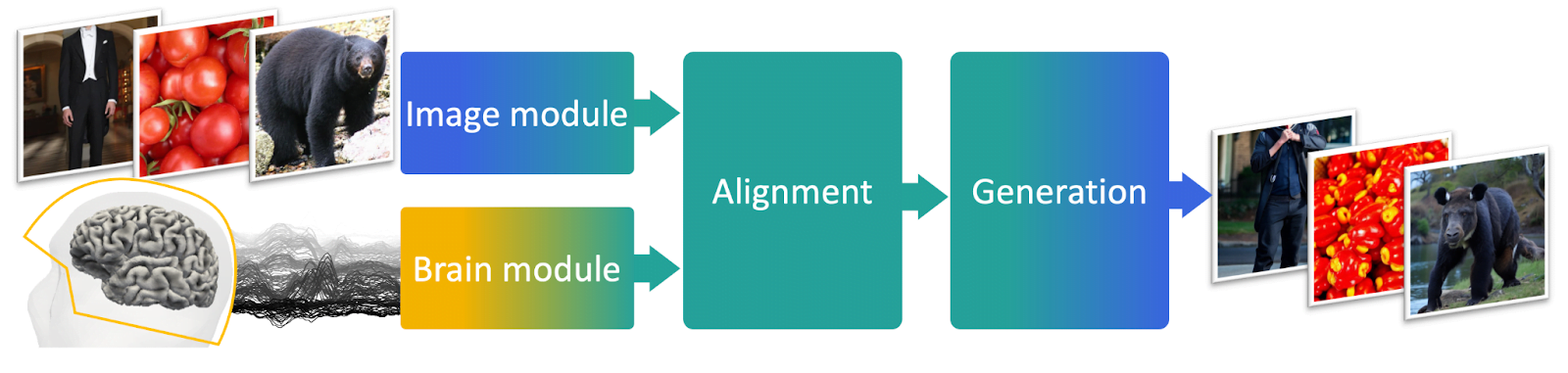
The architecture was trained using a publicly available dataset of MEG recordings from healthy volunteers published by the international academic research consortium Things.
AI systems learn brain-like representations
The research team compared the decoding performance of different pre-trained image modules and found that brain signals are the best match for advanced vision AI systems such as DINOv2. DINOv2 is a self-supervising AI architecture that can learn visual representations without human guidance.
According to Meta AI, the discovery confirms that self-supervised learning leads AI systems to develop brain-like representations, with artificial neurons in the algorithm activating similarly to physical neurons in the brain when exposed to the same image.
While functional magnetic resonance imaging (fMRI) data can provide more accurate images, the MEG decoder can decode images within milliseconds, providing a continuous stream of images directly from brain activity.
Constant decoding of brain activity
These images, while not perfect, represent at a higher level the characteristics of the image as seen by a human, such as the object category (e.g., train, animal, human, etc.). Inaccuracies occur at the detail level (which animal exactly, etc.).
The MEG decoder is not very precise in image generation, but it is very fast, decoding images almost in real-time. | Video: Meta AI
With fMRI techniques, image predictions based on brain data are more accurate, but also slower. | Video: Meta AI
The team's findings show that MEG can decode complex representations generated in the brain with millisecond accuracy. Meta says this contributes to its long-term research vision of understanding the foundations of human intelligence and developing AI systems that learn and think like humans.
Meta's fundamental research in human-like machine intelligence
In early 2022, Yann LeCun, director of research at Meta AI, unveiled a new AI architecture designed to overcome the limitations of current systems. Then this summer, a team from several institutions, including Meta AI and New York University, demonstrated I-JEPA, a visual AI model that follows this new architecture.
I-JEPA, based on the Vision Transformer, was trained in a self-supervised manner to predict details of parts of an image that are not visible. It learned abstract representations of objects rather than operating at the pixel or token level.
These abstract representations, in turn, could help develop AI models that more closely resemble human learning and can draw logical conclusions, according to LeCun's theory.