Microsoft claims that GPT-4, combined with a special prompting strategy, outperforms Google Gemini Ultra in the language understanding benchmark MMLU (Measuring Massive Multitask Language Understanding).
Medprompt is a prompting strategy recently introduced by Microsoft that was originally developed for medical challenges. However, Microsoft researchers have found that it is also suitable for more general applications.
By running GPT-4 with a modified version of Medprompt, Microsoft has now achieved a new State-of-the-Art (SoTA) score on the MMLU benchmark.
Microsoft's announcement is special in that Google highlighted Ultra's new top score on the MMLU benchmark during the big reveal of its new Gemini AI system last week.
Microsoft tricks back: Complex prompts improve benchmark performance
Google's messaging at the time of Gemini's launch was somewhat misleading: the Ultra model achieved the best result in the MMLU benchmark to date, but with a more complex prompting strategy than is usual in this benchmark. With the standard prompting strategy (5-shot), Gemini Ultra performs worse than GPT-4.
The GPT-4 performance in the MMLU now reported by Microsoft with Medprompt+ reaches a record high of 90.10 percent, surpassing Gemini Ultra's 90.04 percent.
To achieve this result, Microsoft researchers extended Medprompt to Medprompt+ by adding a simpler prompt method to Medprompt and formulating a strategy for deriving a final answer that combines answers from both the basic Medprompt strategy and the simpler prompt method.
The MMLU Benchmark is a comprehensive test of general knowledge and reasoning. It contains tens of thousands of items from 57 subject areas, including mathematics, history, law, computer science, engineering, and medicine. It is considered the most important benchmark for language models.
When Microsoft measures performance, GPT-4 outperforms Gemini Ultra on even more benchmarks
In addition to the MMLU benchmark, Microsoft has published results for other benchmarks that show the performance of GPT-4 compared to Gemini Ultra using simple prompts common to these benchmarks. GPT-4 is said to outperform Gemini Ultra in several benchmarks using this measurement method, including GSM8K, MATH, HumanEval, BIG-Bench-Hard, DROP, and HellaSwag.
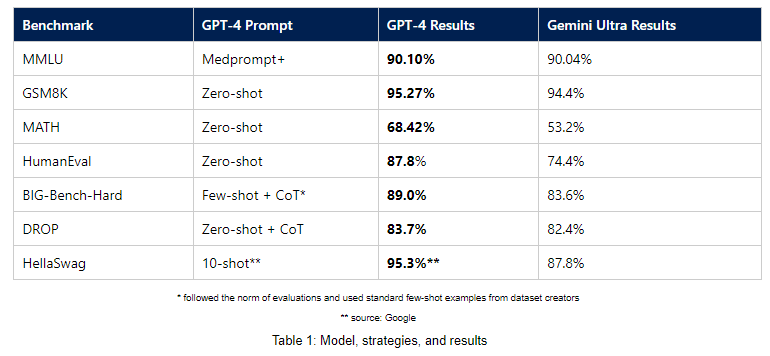
Microsoft publishes Medprompt and similar prompting strategies in a GitHub repository called Promptbase. The repository contains scripts, general tools, and information to help reproduce the results and improve the performance of the base models.
The mostly small differences in the benchmarks are unlikely to matter much in practice; they are mainly used by Microsoft and Google for PR purposes. However, what Microsoft is emphasizing here, and what was already apparent when Ultra was announced, is that the two models are on par.
This could mean that OpenAI is either ahead of Google - or that it is very difficult to develop a much more capable LLM than GPT-4. It could be that LLM technology in its current form has already reached its limits, as Bill Gates recently suggested. GPT-4.5 or GPT-5 from OpenAI might provide some clarity here.