A new study by MIT researchers underscores a notion that has been gaining traction in recent years: Less data can lead to better language models.
The team developed a technique in which small AI models select only the most useful parts of training data sets. They then used this selected data to train much larger models. They found that the language models both performed better on benchmarks and required fewer training steps.
The approach, called "perplexity-based data pruning," has the smaller model assign a perplexity value to each training data set. Perplexity is a measure of how "surprised" the model is by a given example. The idea is that the examples with higher perplexity contain the most information and are therefore potentially the most useful for training the model.
Different approaches for different kinds of training data
In experiments, the researchers used a comparatively small model with 125 million parameters to reduce the training data for models more than 30 times larger.
The large models trained with this reduced data significantly outperformed the base models trained with the full data sets. In one test, pruning increased the accuracy of a model with three billion parameters by more than two percentage points.
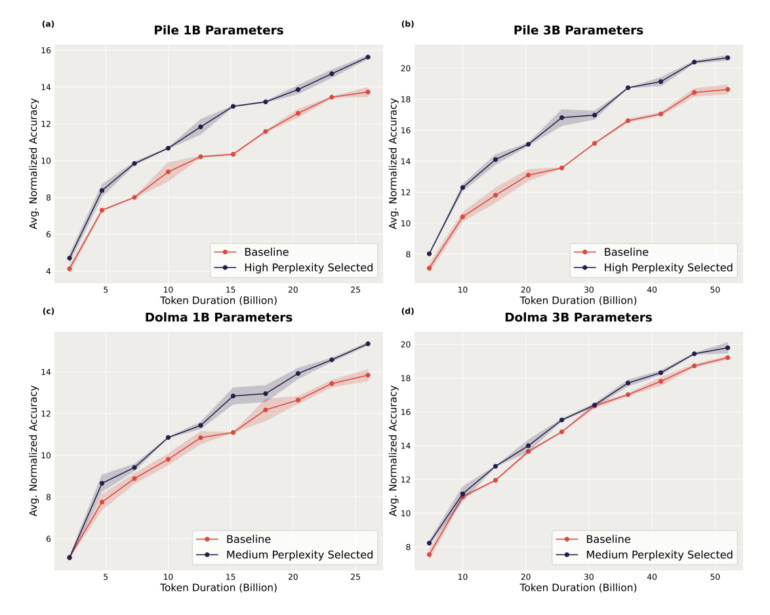
Interestingly, they found that different datasets benefit from different pruning approaches, depending on the composition of the data. As a result, they recommend tailoring the choice of method to the particular data set.
The MIT researchers see their work as an important step toward making data reduction a standard part of AI training, and it confirms previous research that more data does not necessarily lead to better language models.