Japanese researchers unveil a new transistor that could make neuromorphic hardware practical for AI computing.
The field of neuromorphic computing is developing systems that mimic the architecture and computational power of the human brain. One element is so-called "reservoirs" that emulate neural networks and are expected to one day meet the enormous demand for greater computational power and speed in AI research and development.
The idea of reservoirs in neuromorphic computing comes from the concept of reservoir computing. Reservoir computing is a recurrent neural network (RNN) framework in which the recurrent layer (the "reservoir") is randomly generated and does not undergo training. Instead, only the output weights are updated through learning, which simplifies the training process and makes it more efficient.
In neuromorphic computing, such reservoirs can be implemented on different substrates, such as analog electronic circuits, optoelectronic systems, or mechanical systems. In this context, the term "physical reservoir computing" is used.
These reservoirs behave like neural networks that change over time depending on the interaction and processing of input data, and are thus capable of transforming data into high-dimensional representations suitable for complex tasks such as object recognition. In practice, however, these systems require a large number of reservoir states that are difficult to achieve with current hardware.
New transistor doubles reservoir states
To overcome the compatibility, performance, and integration problems of such memory systems, Japanese researchers have now developed a new transistor. According to the team, this development opens up new possibilities for neuromorphic high-performance computing.
The team's new transistor, called an ion-gate reservoir transistor, can generate a record number of reservoir states. According to the team, the transistor uses an electrolyte through which lithium ions move rapidly, creating two output currents and effectively doubling the number of reservoir states.
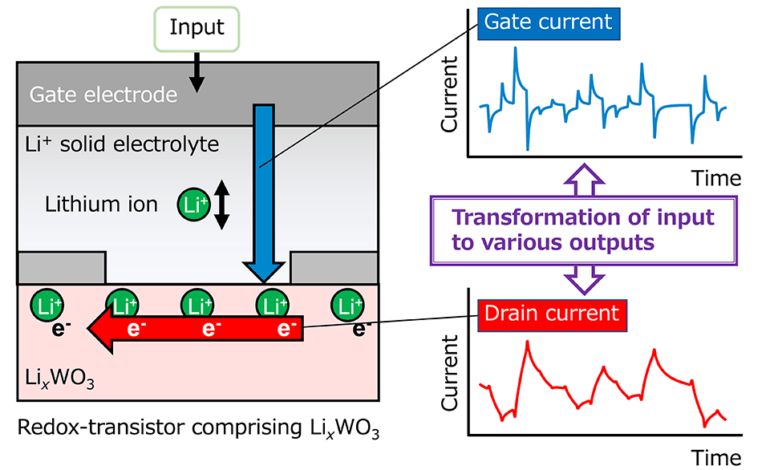
In addition, the different rates of ion transport in the channel and in the electrolyte result in a delay between two currents, the drain current and the gate current. This delay allows the system to briefly store information from previous entries and use it for future operations, an essential requirement for physical memories.
In tests, this device outperformed other similar technologies such as memristors, and proved to be highly accurate in making predictions based on past input and output data. According to Associate Professor Dr. Tohru Higuchi of Tokyo University of Science (TUS) the system has the potential to become a "general-purpose technology that will be implemented in a wide range of electronic devices including computers and cell phones in the future."