OpenAI introduces a new method that dramatically simplifies and accelerates the training of AI image models.
OpenAI has introduced a new method called sCM (simplified, stabilized and scaled Consistency Models) that improves the training of image generation models. The technique builds on Consistency Models (CMs), a class of diffusion-based generative models that OpenAI has been researching to optimize fast image sampling.
The new sCM method makes training these models more stable and scalable. According to OpenAI, the new models can generate high-quality images in just two computation steps, while previous methods required significantly more steps. OpenAI reports that their largest sCM model, with 1.5 billion parameters, achieves an image generation time of only 0.11 seconds per image on an A100 GPU without special optimizations. This represents a 50-fold speed increase compared to conventional diffusion models.
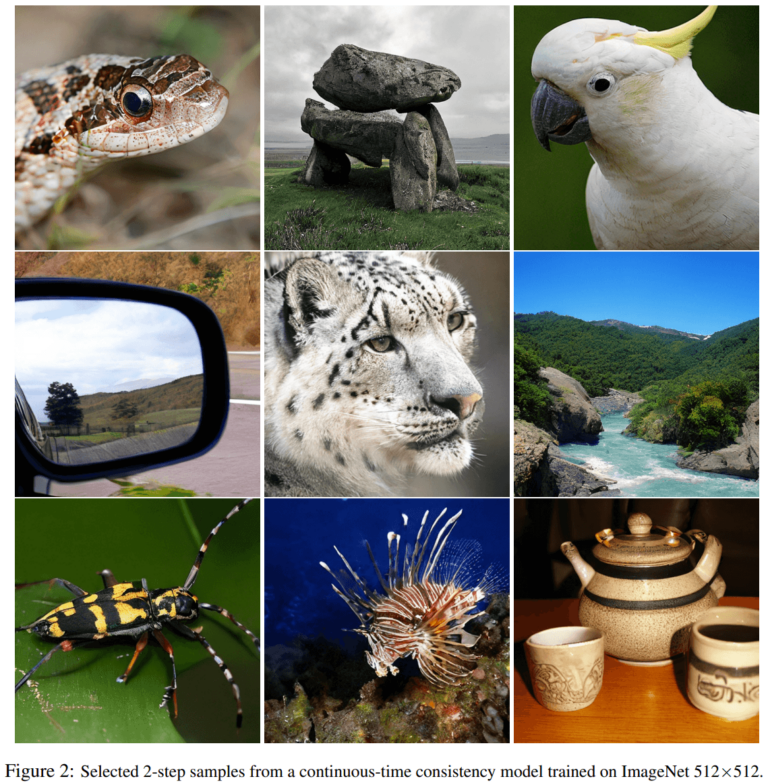
Technical breakthrough in image generation
OpenAI says the new method solves a fundamental problem: Previous Consistency Models worked with discrete time steps, which required additional parameters and was error-prone. The researchers developed a simplified theoretical framework that unifies various approaches. This allowed them to identify and fix the main causes of training instabilities.
The results are significant: In tests, the system achieved FID scores of 2.06 on the CIFAR-10 dataset and 1.88 on ImageNet with 512x512 pixel images using just two computation steps. By these metrics, the quality of the generated images is only about ten percent behind the best existing diffusion models.
Scaling to record size possible
The new method also excels at scaling. OpenAI successfully trained models with up to 1.5 billion parameters on the ImageNet dataset - an unprecedented size for this type of model. The researchers observed that image quality consistently improves as model size increases.
This suggests the method could work for even larger models. It's an important development for the future of AI image generation - and potentially for video, audio, and 3D models as well.
More details and examples are available in OpenAI's blog post.