An AI model called Phenaki can generate minutes of coherent video based on detailed, sequential text input.
On the same day as Meta's "Make a Video," a second text-to-video system made the rounds online: it's called Phenaki, and according to the authors, it can generate minutes-long, connected videos based on sequential text commands.
The authors are anonymous because the work is still in the review process for the AI conference International Conference on Learning Representations (ICLR).
Temporal correlations
Unlike AI-generated images, single prompts are not sufficient for AI-generated videos, the authors say. An AI video system must be conditioned to multiple, interrelated prompts - a story that describes events over time.
The researchers therefore developed a transformer-based model that compresses videos into small representations of discrete tokens. This tokenizer uses time-dependent causal attention so that video sequences can be strung together along a temporal sequence of events described in a prompt.
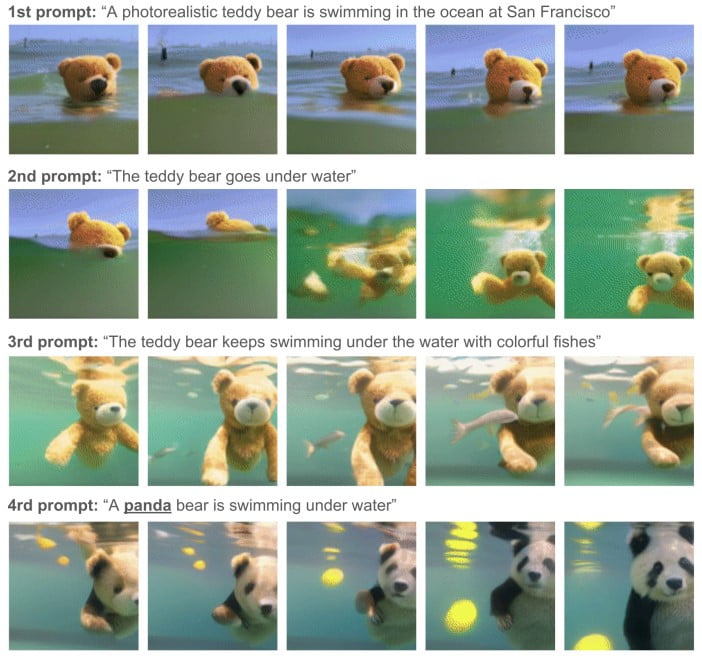
"We treat videos as a temporal sequence of images (rather than a single sequence, editor's note), which substantially decreases the number of video tokens given the redundancy in video generation, and results in a much lower training cost," the research team writes.
Simultaneous learning from image-text and video-text pairs
Like large image systems, the multimodal model was trained primarily with text-image pairs. In addition, the researchers trained Phenaki with 1.4-second short video-text pairs at eight frames per second.
"We demonstrate how joint training on a large corpus of image-text pairs as well as a smaller number of video-text examples can result in generalization beyond what is available in the video datasets," the researchers write.
The system can even process prompts that represent a new composition of concepts or animate pre-existing images based on text input, according to the researchers.
As an example of a longer video, the team shows the following clip. It was generated using a detailed text command that describes scene by scene.
Lots of traffic in futuristic city. An alien spaceship arrives to the futuristic city. The camera gets inside the alien spaceship. The camera moves forward until showing an astronaut in the blue room. The astronaut is typing in the keyboard. The camera moves away from the astronaut. The astronaut leaves the keyboard and walks to the left. The astronaut leaves the keyboard and walks away. The camera moves beyond the astronaut and looks at the screen. The screen behind the astronaut displays fish swimming in the sea. Crash zoom into the blue fish. We follow the blue fish as it swims in the dark ocean. The camera points up to the sky through the water. The ocean and the coastline of a futuristic city. Crash zoom towards a futuristic skyscraper. The camera zooms into one of the many windows. We are in an office room with empty desks. A lion runs on top of the office desks. The camera zooms into the lion's face, inside the office. Zoom out to the lion wearing a dark suit in an office room. The lion wearing looks at the camera and smiles. The camera zooms out slowly to the skyscraper exterior. Timelapse of sunset in the modern city.
video: Phenaki demo
No publication yet due to ethical concerns
According to the authors, Phenaki was trained with various datasets, including the LAION-400M dataset, which contains violent, gory, and pornographic content, but improved the quality of generation. A current version of Phenaki uses datasets that minimize "such problems."
Nevertheless, the team does not want to publish the models trained for Phenaki, the code, the data, or an interactive demo at this time for ethical reasons. Before a possible release, it first wants to better understand the data, inputs and filtered outputs. It also wants to measure the bias contained in the generated videos to minimize bias at each step of model development (data selection, training, filtering).