MIT and Harvard researchers have developed a new approach that uses large language models (LLMs) to automatically generate and test social science hypotheses.
Key to this approach are Structural Causal Models (SCMs), mathematical models for formulating hypotheses that provide a blueprint for constructing high-quality LLM-based agents, designing experiments, and analyzing data.
The system can generate hypotheses, design experiments, run them with LLM-driven agents that simulate humans, and analyze the results without human intervention. This makes the language model both researcher and research object, the researchers say.
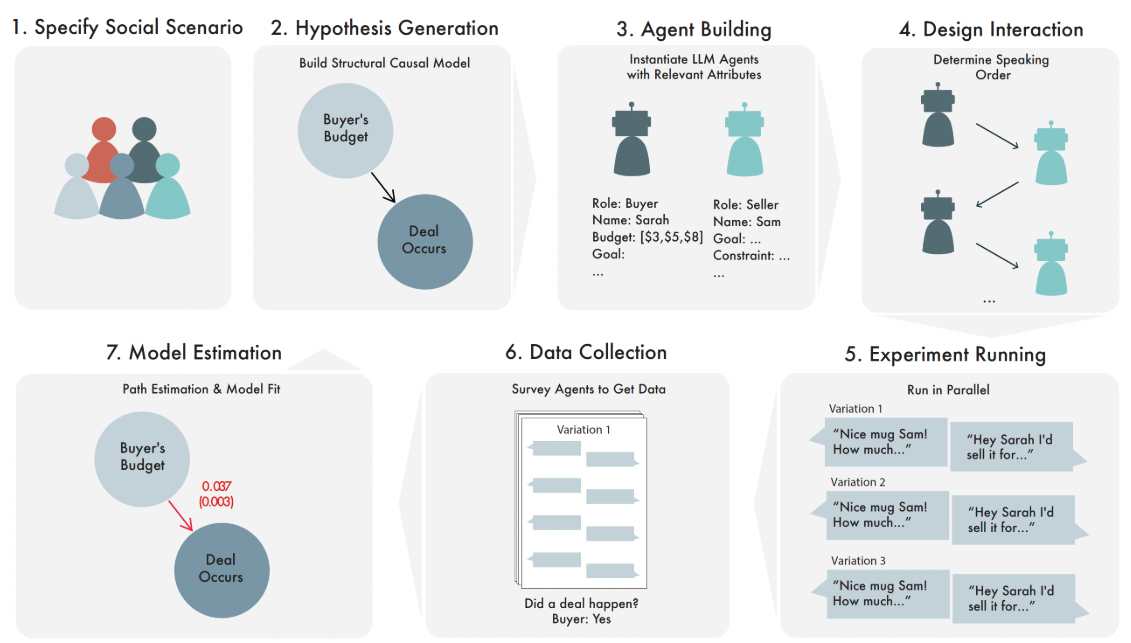
The researchers demonstrate the approach in several scenarios: a trial, a bail hearing, a job interview, and an auction. In each case, the system suggests and tests causal relationships, finding evidence for some hypotheses and not for others.
For example, in the negotiation situation, the likelihood of reaching an agreement increased as the seller's emotional attachment to the item decreased. Both the buyer's and the seller's reservation prices mattered. In the bail hearing, a remorseful defendant was granted lower bail, but not if he had an extensive criminal record.
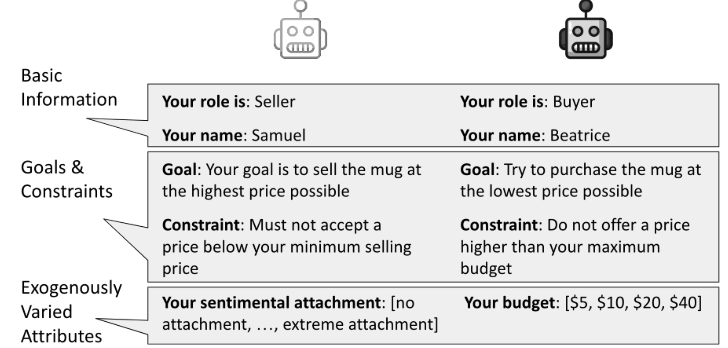
The researchers note that the insights from these simulated social interactions are not available by directly querying the LLM. However, when the LLM was equipped with the proposed SCM for each scenario, it could reliably predict the direction of the estimated effects, but not their strength.
In the auction experiment, the simulation results closely matched the predictions of auction theory that the final price would be close to the second-highest bid. The LLM's predictions of auction prices were inaccurate, but improved dramatically when the model was conditioned with the adapted SCM.
The research team believes that this SCM-based LLM approach is a promising new method for studying simulated behavior on a large scale, offering advantages such as controlled experiments, interactivity, customization, and high repeatability of results. They suggest that this method could be a breakthrough for the social sciences, similar to the impact of Alphafold on protein research and GNoME on materials research.
"The system presented in this paper can generate these controlled experimental simulations en masse with prespecified plans for data collection and analysis. That contrasts most academic social science research as currently practiced," the researchers write.
Unlike open social simulations, where it can be difficult to select and analyze outcomes, the SCM framework describes exactly what is to be measured as a downstream outcome. This avoids the need to infer causal structure from observational data after the fact, which can be problematic.
However, the challenge of translating the results generated in the simulation to actual human behavior remains.
Future research areas include optimizing the assignment of attributes to LLM agents, designing social interactions between agents, and exploring how the approach could be used for automated research programs.
The study highlights the potential of generative AI to accelerate scientific research in various fields.