VideoControlNet uses diffusion models and ControlNet for more control over AI-generated video.
There are several extensions to better control image synthesis for Stable Diffusion. One of the most important is ControlNet. It allows, for example, the poses of people or the structure of a room to be extracted from an input image and used as a template for image synthesis.
Researchers at Beihang University and the University of Hong Kong now present VideoControlNet, an extension that applies this idea to video synthesis.
Video synthesis using diffusion models is still plagued by artifacts and difficult to control, despite some impressive results such as Runways Gen-2. VideoControlNet, on the other hand, uses prompts and an input video together to generate new videos. This makes it possible to replace backgrounds, lighting, or people while preserving the geometry and temporal structure of the original.
VideoControlNet is based on video codec methods
VideoControlNet is inspired by the way video codecs reduce unnecessary repetitive information in a video sequence. Specifically, the team defines the first frame as an I-frame and divides subsequent frames into different groups of frames (GoPs), with the last frame of each GoP defined as a key frame (P-frame) and the other frames as B-frames.
The first frame of the video, called the I-frame, is generated using a diffusion model and ControlNet. Then the P-frames are generated based on changes in the previous frame, that is, the I-frame or other P-frames. The team has developed a technique they call motion-guided P-frame generation (MgPG). When parts of the image are occluded, the diffusion model fills them in.
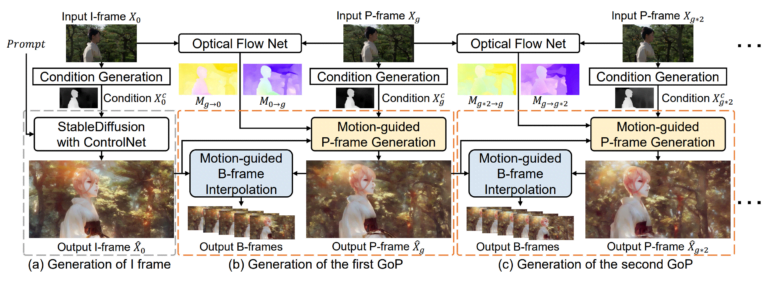
Finally, all the remaining frames, or B-frames, are generated using a method the team calls motion-guided B-frame interpolation (MgBI). These B-frames are based on information from previous and subsequent B-frames.
Next project aims to improve consistency
In experiments, the team shows that VideoControlNet retains the generative capabilities of the diffusion model it uses and successfully extends them to video by using motion information.
The team shows examples of style transfer, foreground, and background video editing.
Style Transfer
video: Hu, Xu
Foreground
Video: Hu, Xu
Background
Video: Hu, Xu
Next, the team hopes to integrate more learning networks to improve consistency.
More examples and the code are available on the VideoControlNet project page.