Researchers have developed a method called "WildGaussians" that extends 3D Gaussian splatting for scenes with varying appearances and lighting conditions. The approach enables photorealistic 3D reconstruction from unstructured image collections.
A team of researchers from the Czech Technical University in Prague and ETH Zurich has introduced a method called "WildGaussians" that makes the 3D Gaussian splatting (3DGS) technique accessible for unstructured photo collections from the web, such as images of landmarks.
Video: Kulhanek, Peng et al.
WildGaussians addresses two main challenges in 3D reconstruction of such unstructured image collections: varying appearances and lighting, and occlusion by moving objects. To tackle these issues, the team developed two key components: appearance modeling and uncertainty modeling.
Appearance modeling allows WildGaussians to process images captured under different conditions, such as time of day or weather. It uses trainable embeddings for each training image and Gaussian distribution. A neural network (MLP) utilizes these embeddings to adapt the colors of the Gaussian distributions to the respective capture conditions.
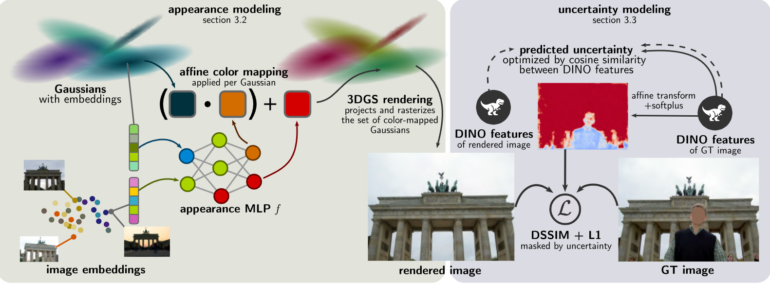
Uncertainty modeling helps identify and ignore occlusions like pedestrians or cars during training. The researchers rely on pre-trained DINOv2 features, which are more robust to changes in the landscape compared to conventional methods.
WildGaussians outperforms existing methods and runs at nearly 120 images per second
The scientists evaluated WildGaussians on two challenging datasets: the NeRF On-the-go Dataset with varying degrees of occlusion and the Photo Tourism Dataset containing user-captured images of famous landmarks under different conditions. The new approach surpassed the quality of current state-of-the-art methods in most examples while enabling real-time rendering at 117 images per second on an Nvidia RTX 4090 GPU.
The researchers see WildGaussians as an important step towards robust and versatile photorealistic reconstruction from noisy, user-generated data sources. However, they acknowledge that the method still has limitations, such as the representation of specular highlights on objects. They aim to reduce these limitations in the future by integrating additional information sources like diffusion models.
More examples and comparisons are available on the project page.