Deepmind's SIMA can perform tasks in different video game worlds, such as Valheim or No Man's Sky, using only text prompts.
Google Deepmind researchers introduce SIMA (Scalable Instructable Multiworld Agent), an AI agent for 3D video game environments that can translate natural language instructions into actions.
SIMA was trained and tested in collaboration with eight game studios and across nine different video games, including No Man's Sky, Valheim, and Teardown.
Video: Google Deepmind
The Deepmind team trained SIMA using game recordings in which a player either gave instructions to another player or described their own game. The team then linked these instructions to game actions.
The agent is primarily trained to imitate behavior (behavioral cloning). It imitates the actions performed by the people in the collected data while following the language instructions.
In this way, the agent learns to make connections between the language descriptions, visual impressions, and corresponding actions.
Google Deepmind SIMA uses pre-trained models and learns from humans
The core of the SIMA agent consists of several components that work together to convert visual input (what the agent "sees") and language input (the instructions it receives) into actions (keyboard and mouse commands).
Image and text encoders are responsible for translating the visual and language input into a form that the agent can process. This is done using pre-trained models that already have a comprehensive understanding of images and text.
A transformer model integrates the information from the encoders and past actions to form an internal representation of the current state. A special memory mechanism helps the agent to remember previous actions and their results, which is crucial for understanding multi-step tasks.
Finally, the agent uses this state representation to decide which actions to perform next. These actions are keyboard and mouse commands executed in the virtual environment.
SIMA does not require access to the game's source code, only screen images and natural language instructions. The agent interacts with the virtual environment via keyboard and mouse and is therefore potentially compatible with any virtual environment.
SIMA masters 600 skills
In tests, SIMA mastered 600 basic skills such as navigation, object interaction, and menu control. The team expects future agents to be able to perform complex strategic planning and multifaceted tasks.
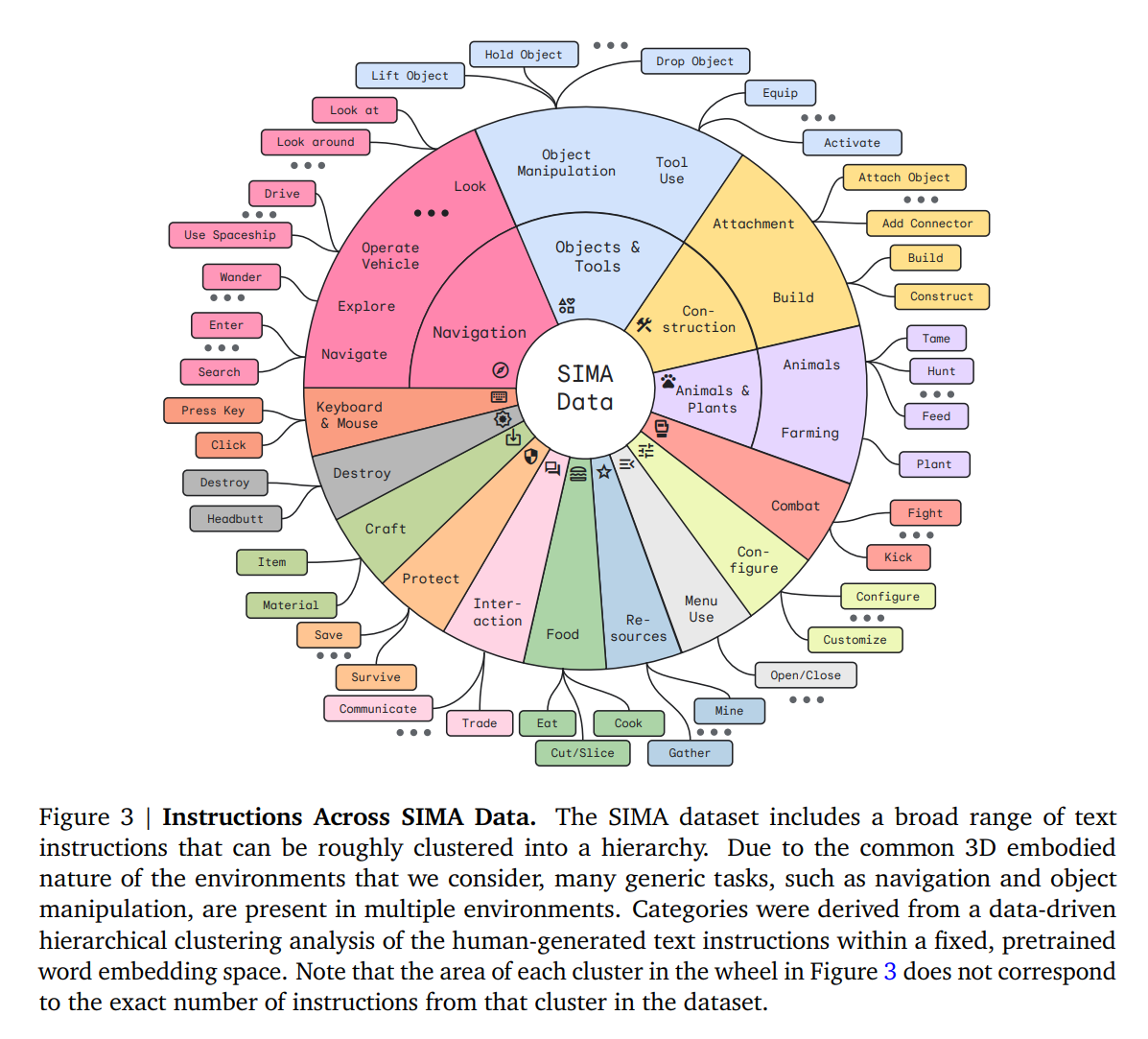
SIMA differs from other AI systems for video games in that it takes a broad approach, learning in a variety of environments rather than focusing on one or a few specific tasks.
Research shows that an agent trained in many games performs better than an agent specialized in a single game. In addition, SIMA integrates pre-trained models to take advantage of existing knowledge about language and visual perception, and combines this with specific training data from the 3D environments.
The team hopes that this research will contribute to the development of a new generation of general-purpose, language-driven AI agents. With more sophisticated models, projects like SIMA could one day achieve complex goals and become useful on the Internet and in the real world.