Researchers are giving AI agents access to a "world knowledge model". This should enable them to solve tasks more effectively, without going nowhere or generating nonsense.
Agents based on large language models such as GPT-4 repeatedly show potential in planning and solving complex tasks. However, they often operate on a trial-and-error basis and hallucinate unrealistic actions. In a new study, researchers from Zhejiang University and Alibaba are investigating whether an external, learned "World Knowledge Model" (WKM), which provides AI agents with additional knowledge, can improve the performance of the models.
Similar to how humans build mental models of the world, AI agents should be able to use such a model.
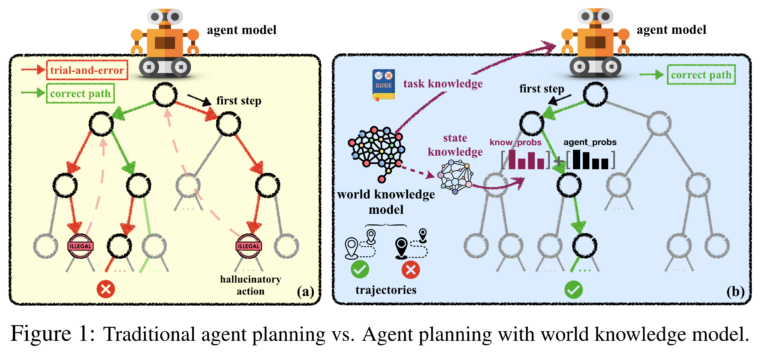
The researchers distinguish between global "task knowledge" and local "state knowledge". Task knowledge is intended to give the agent an overview of the steps required to solve a task in advance and to avoid taking the wrong path. State knowledge records what the agent knows about the current state of the world at each step. This is to avoid contradictions.
To train the external model, the researchers allow the agent to extract knowledge from successful and unsuccessful problem-solving attempts by humans and from its own attempts. The agent then uses this to generate relevant task and state knowledge.
External model improves performance in new tasks
In the planning phase, the WKM first provides the task knowledge as a guideline. It then generates a description of the current state for each step. It searches a knowledge base for similar states and their subsequent actions. The next action is then selected from their probabilities and those of the agent model.
Experiments show that AI agents with WKM perform significantly better than those without. Knowledge of the world pays off, especially for new, unknown tasks.
Specifically, the team tested the agents on three complex, realistically simulated datasets: ALFWorld, WebShop and ScienceWorld.
In ALFWorld, the agents have to perform tasks in a virtual household situation, such as picking up objects and interacting with household appliances. WebShop simulates a shopping experience where the agents have to find and buy certain items in a virtual store. ScienceWorld requires agents to perform scientific experiments in a virtual laboratory environment.
The team tested open source LLMs (Mistral-7B, Gemma-7B and Llama-3-8B) and WKMs and compared their performance with that of GPT-4. The experiments showed that the developed WKMs were able to significantly improve the performance of the agents and in some tasks outperformed GPT-4 in a duel. In a separate experiment, the team also showed that the smaller models plus WKM can be used to train GPT-4 and significantly improve its performance.
Next, the researchers want to train a unified world knowledge model that can support different agents in different tasks.