In current models, the only way to describe where an object should be placed in an AI image is with text - with only moderate success. Researchers now present a model that uses bounding boxes.
AI image generation has rapidly evolved from diffuse visualizations to very concrete, sometimes even photorealistic results. The more detailed the specification, the better the generation can be influenced. Although details of the image composition can be described with text, such as where an object should be placed, these details are often only moderately implemented. A new method could make this easier in the future.
Scientists at the University of Wisconsin-Madison, Columbia University and Microsoft have presented GLIGEN, which stands for Grounded-Language-to-Image Generation. With a few exceptions, such as Stable Diffusion 2.0, image models can only be controlled by text. GLIGEN, on the other hand, uses so-called "grounding inputs".
Positional information through boxes or keypoints
"Grounding" here refers to the ability of a model to incorporate visual information. In the context of GLIGEN, this means that the AI model uses visual information, such as the position and size of bounding boxes, in addition to text input.
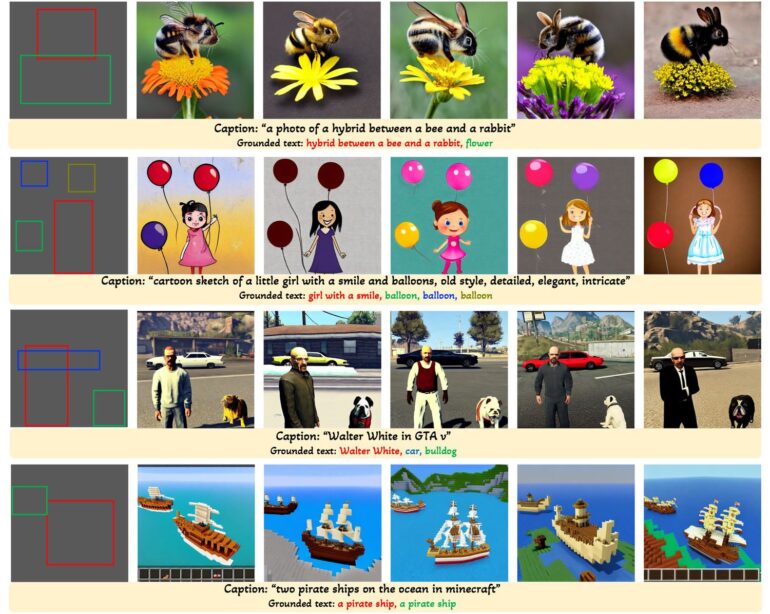
The boxes can overlap, be given more details using Img2Img, and added to existing images using inpainting. When creating images of people, key points can also be defined to reflect the person's posture and guide image synthesis accordingly.
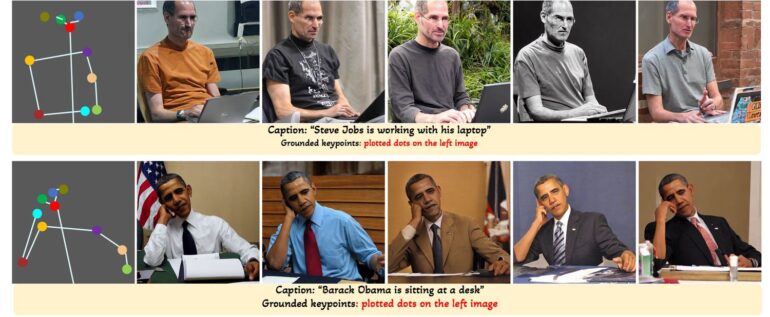
Training layer "like Lego" over the model.
For training, GLIGEN freezes all the weights of the underlying image model and trains the grounding information via box-text image pairs in new layers. In this way, the researchers ensure that the capabilities of the pre-trained model are preserved.
Compared with other ways of using a pretrained diffusion model such as full-model finetuning, our newly added modulated layers are continual pre-trained on large grounding data (image-text-box) and is more cost-efficient. Just like Lego, one can plug and play different trained layers to enable different new capabilities.
Li et al.
GLIGEN is similar to the recently released ControlNet, which however comes with additional control capabilities. This allows users to have much more control over the output of the AI image generators, allowing them to generate images exactly as desired.
You can try GLIGEN for free in the browser on Hugging Face, but it has not yet made it into an application for an image model like Stable Diffusion.